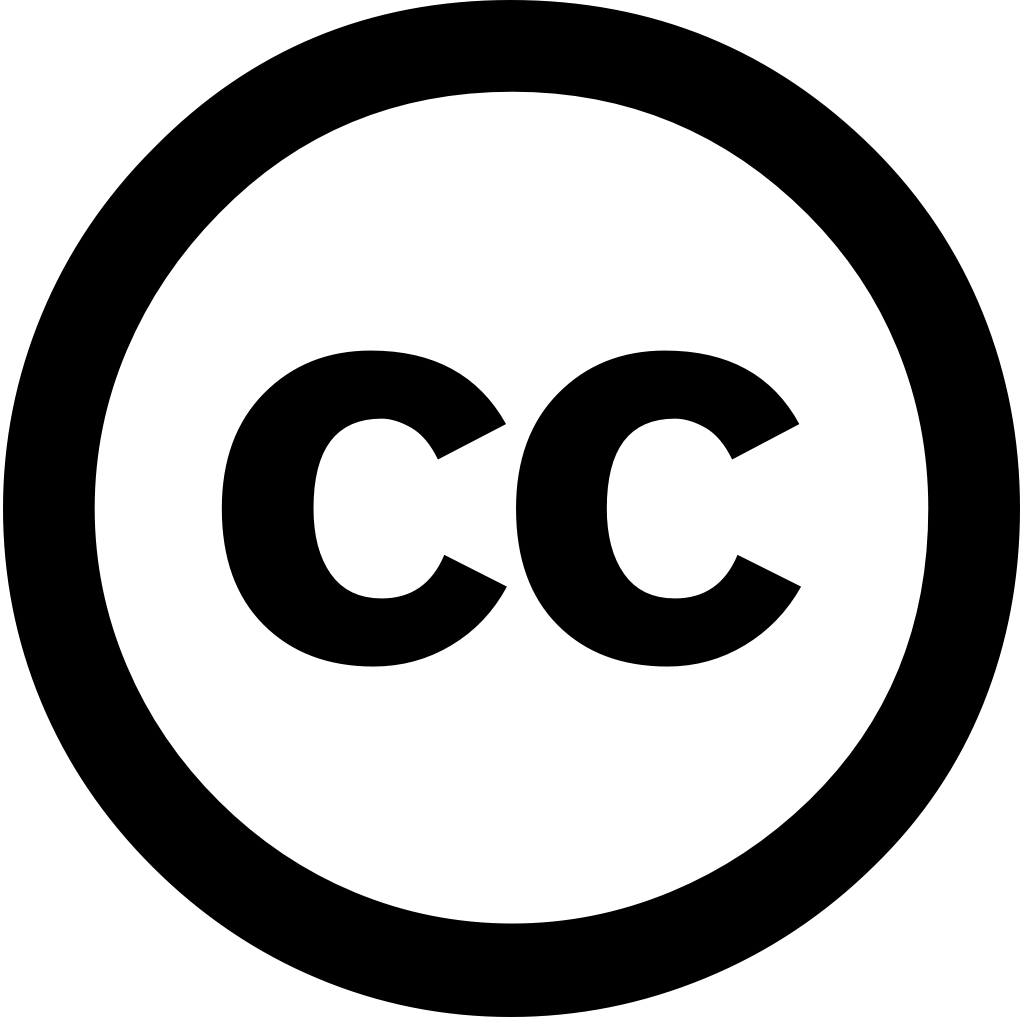
Agronomy, Год журнала: 2025, Номер 15(3), С. 525 - 525
Опубликована: Фев. 21, 2025
This study addresses the challenge of segmenting strawberry leaves and lesions in natural backgrounds, which is critical for accurate disease severity assessment automated dosing. Focusing on powdery mildew, we propose an enhanced YOLOv8-based segmentation method leaf lesion detection. Four instance models (SOLOv2, YOLACT, YOLOv7-seg, YOLOv8-seg) were compared, using YOLOv8-seg as baseline. To improve performance, SCDown PSA modules integrated into backbone to reduce redundancy, decrease computational load, enhance detection small objects complex backgrounds. In neck, C2f module was replaced with C2fCIB module, SimAM attention mechanism incorporated target differentiation noise interference. The loss function combined CIOU MPDIOU adaptability challenging scenarios. Ablation experiments demonstrated a accuracy 92%, recall 85.2%, mean average precision (mAP) 90.4%, surpassing baseline by 4%, 2.9%, respectively. Compared SOLOv2, improved model’s mAP increased 14.8%, 5.8%, 3.9%, model reduces missed detections enhances localization, providing theoretical support subsequent applications intelligent, dosage-based management.
Язык: Английский