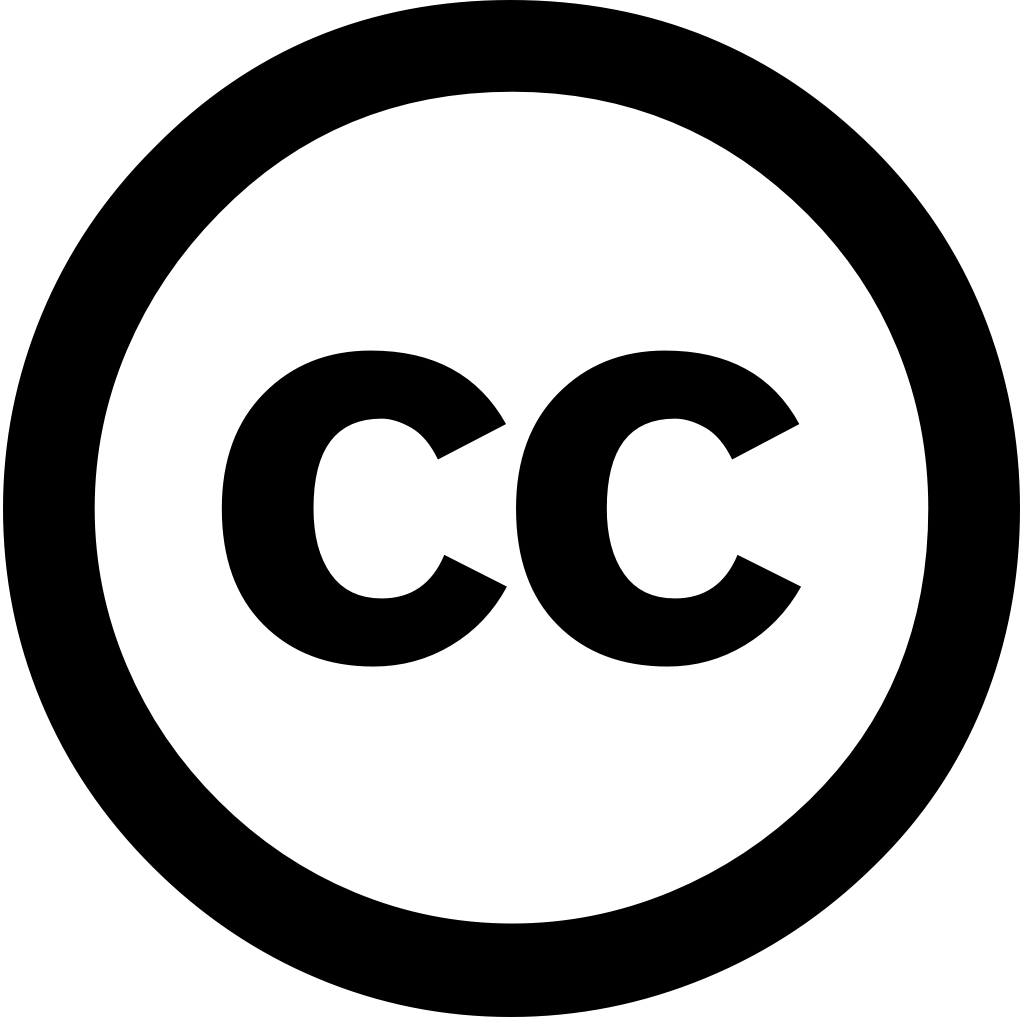
Algorithms, Год журнала: 2025, Номер 18(2), С. 118 - 118
Опубликована: Фев. 19, 2025
This paper introduces an improved version of the Federated Random High Local Performance (Fed-RHLP) algorithm, specifically aimed at addressing difficulties posed by Non-IID (Non-Independent and Identically Distributed) data within context federated learning. The refined Fed-RHLP algorithm implements a more targeted client selection approach, emphasizing clients based on size their datasets, diversity labels, performance local models. It employs biased roulette wheel mechanism for selecting clients, which improves aggregation global model. approach ensures that model is primarily influenced high-performing while still permitting contributions from those with lower during training process. Experimental findings indicate significantly surpasses existing methodologies, including FederatedAveraging (FedAvg), Power Choice (PoC), FedChoice, achieving superior accuracy, accelerated convergence rates, decreased execution times, especially under conditions high data. Furthermore, exhibits resilience even when number participating in updates diminished each communication round. characteristic positively influences conservation limited computational resources.
Язык: Английский