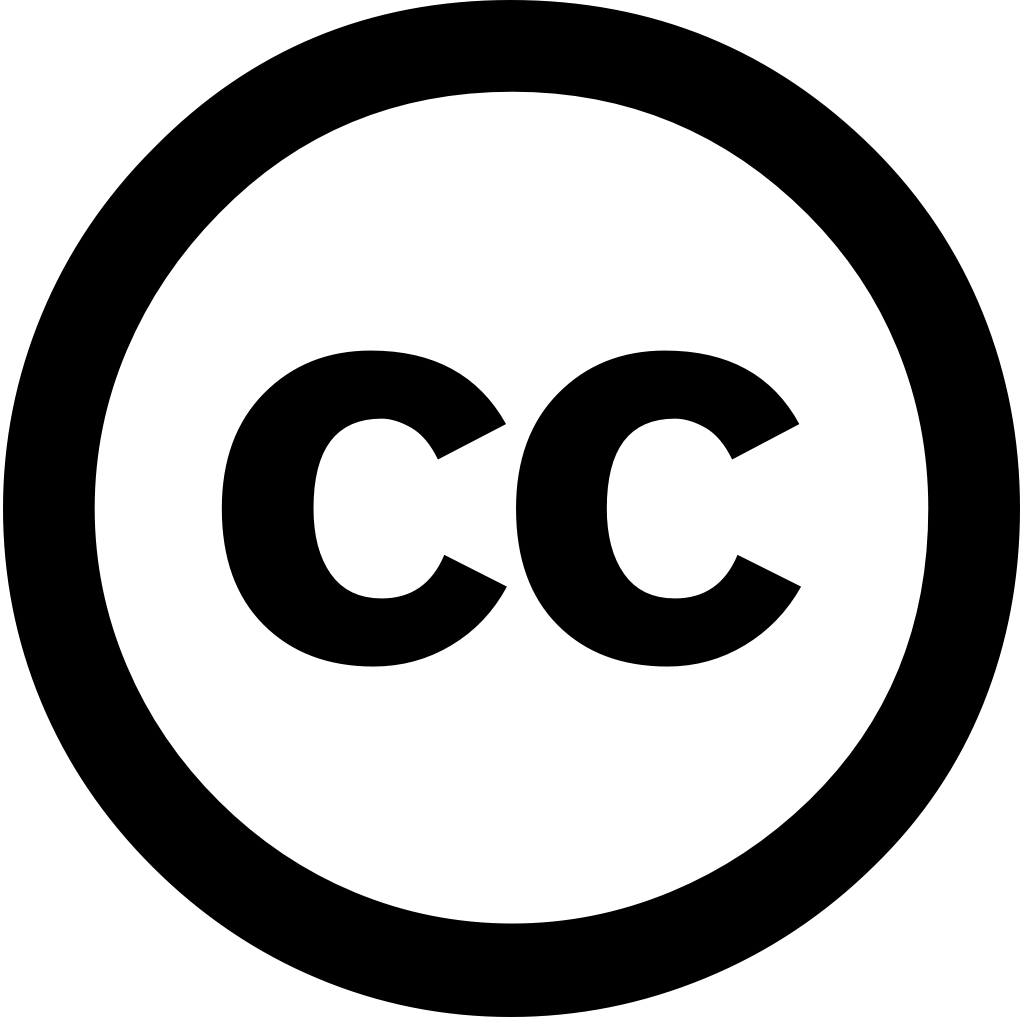
Research Square (Research Square), Год журнала: 2024, Номер unknown
Опубликована: Апрель 2, 2024
Язык: Английский
Research Square (Research Square), Год журнала: 2024, Номер unknown
Опубликована: Апрель 2, 2024
Язык: Английский
Diagnostics, Год журнала: 2025, Номер 15(3), С. 285 - 285
Опубликована: Янв. 25, 2025
Background: Breast cancer screening through mammography interpretation is crucial for early detection and improved patient outcomes. However, the manual classification of mammograms using BIRADS (Breast Imaging-Reporting Data System) remains challenging due to subtle imaging features, inter-reader variability, increasing radiologist workload. Traditional computer-aided systems often struggle with complex feature extraction contextual understanding mammographic abnormalities. To address these limitations, this study proposes MammoViT, a novel hybrid deep learning framework that leverages both ResNet50’s hierarchical capabilities Vision Transformer’s ability capture long-range dependencies in images. Methods: We implemented multi-stage approach utilizing pre-trained ResNet50 model initial from mammogram significant class imbalance our four-class dataset, we applied SMOTE (Synthetic Minority Over-sampling Technique) generate synthetic samples minority classes. The extracted arrays were transformed into non-overlapping patches positional encodings Transformer processing. employs multi-head self-attention mechanisms local global relationships between image patches, each attention head different aspects spatial dependencies. was optimized Keras Tuner trained 5-fold cross-validation stopping prevent overfitting. Results: MammoViT achieved 97.4% accuracy classifying images across categories. model’s effectiveness validated comprehensive evaluation metrics, including report, confusion matrix, probability distribution, comparison existing studies. Conclusions: effectively combines architectures while addressing challenge imbalanced medical datasets. high robust performance demonstrate its potential as reliable tool supporting clinical decision-making breast screening.
Язык: Английский
Процитировано
0Proceedings of international conference on intelligent systems and new applications., Год журнала: 2024, Номер unknown
Опубликована: Апрель 28, 2024
Breast cancer, a heterogeneous disease, can be classified into several subtypes, each associated with distinct genetic mutations and clinical outcomes. As per the research article by National Institutes of Health it was stated that 25% hereditary cases are due to mutation highly penetrant genes which leads 80% lifetime risk breast cancer [15]. This study aims apply advanced deep learning machine algorithms predict subtypes identify key contributing disease using comprehensive gene expression dataset. We analyzed dataset comprising 1904 samples, encompassing 331 175 mutations, sourced from public platform including PAM50 Claudin low categorizations. Due limited observations, SMOTE employed for data augmentation, Principal Component Analysis (PCA) used assess variance. Several models, Random Forest Classifier, Support Vector Machine, K-Nearest Neighbor, XGBoost, Stacked were applied alongside techniques like Convolutional Neural Network Multi-Layer Perceptron. The model demonstrated superior performance an accuracy 0.955, outperforming other models. models achieved accuracies 0.911 (CNN) 0.936 (MLP). KNN analysis revealed potential clusters based on data, silhouette metric identifying "siah1_mut," "nras_mut," "hras_mut" as significant mutations. optimal clustering score 0.997 two clusters. These may play pivotal roles in pathogenesis could serve targets therapeutic interventions. Our findings demonstrate effectiveness integrating stacked predicting subtypes. identification through provides valuable insights underpinnings guide future development targeted therapies. highlights computational approaches elucidating complex landscape genomics paves way personalized medicine oncology.
Язык: Английский
Процитировано
1Опубликована: Ноя. 24, 2024
Язык: Английский
Процитировано
0Research Square (Research Square), Год журнала: 2024, Номер unknown
Опубликована: Апрель 2, 2024
Язык: Английский
Процитировано
0