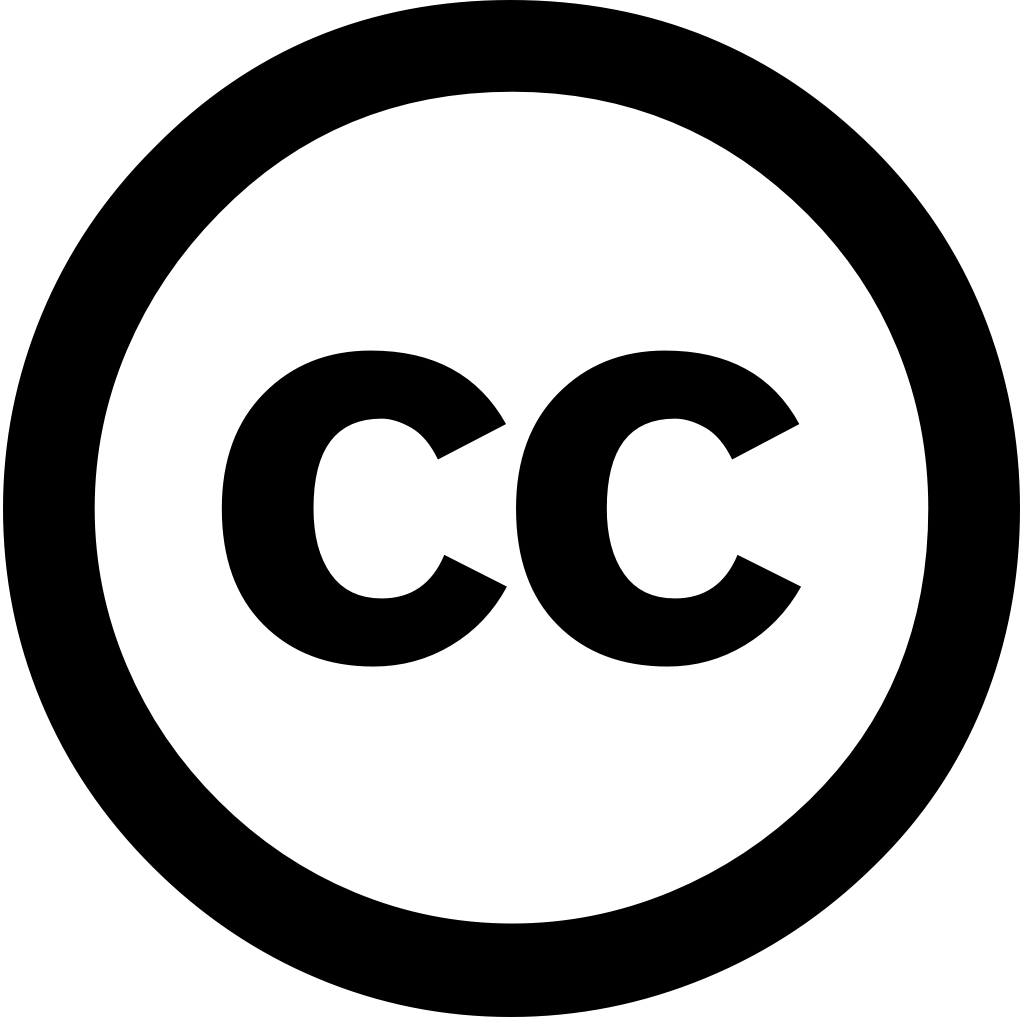
Journal of Marine Science and Engineering, Год журнала: 2025, Номер 13(3), С. 410 - 410
Опубликована: Фев. 22, 2025
Data generated during the shutdown or start-up processes of wind turbines, particularly in complex conditions such as offshore environments, often accumulate low-wind-speed region, leading to reduced multivariate power estimation accuracy. Therefore, developing efficient filtering methods is crucial improving data quality and model performance. This paper proposes a novel method that integrates control strategies variable-speed, variable-pitch maximum-power point tracking (MPPT) pitch angle control, with statistical distribution characteristics derived from supervisory acquisition (SCADA). First, thresholds for rotor speed are determined based on SCADA distribution, effect visualized. Subsequently, sliding window technique employed secondary confirmation potential outliers, enabling further anomaly detection (AD). Finally, performance validated using two turbine datasets machine learning algorithms, results compared without filtering. The demonstrate proposed significantly enhances accuracy estimation, proving its effectiveness turbines operating diverse environments.
Язык: Английский