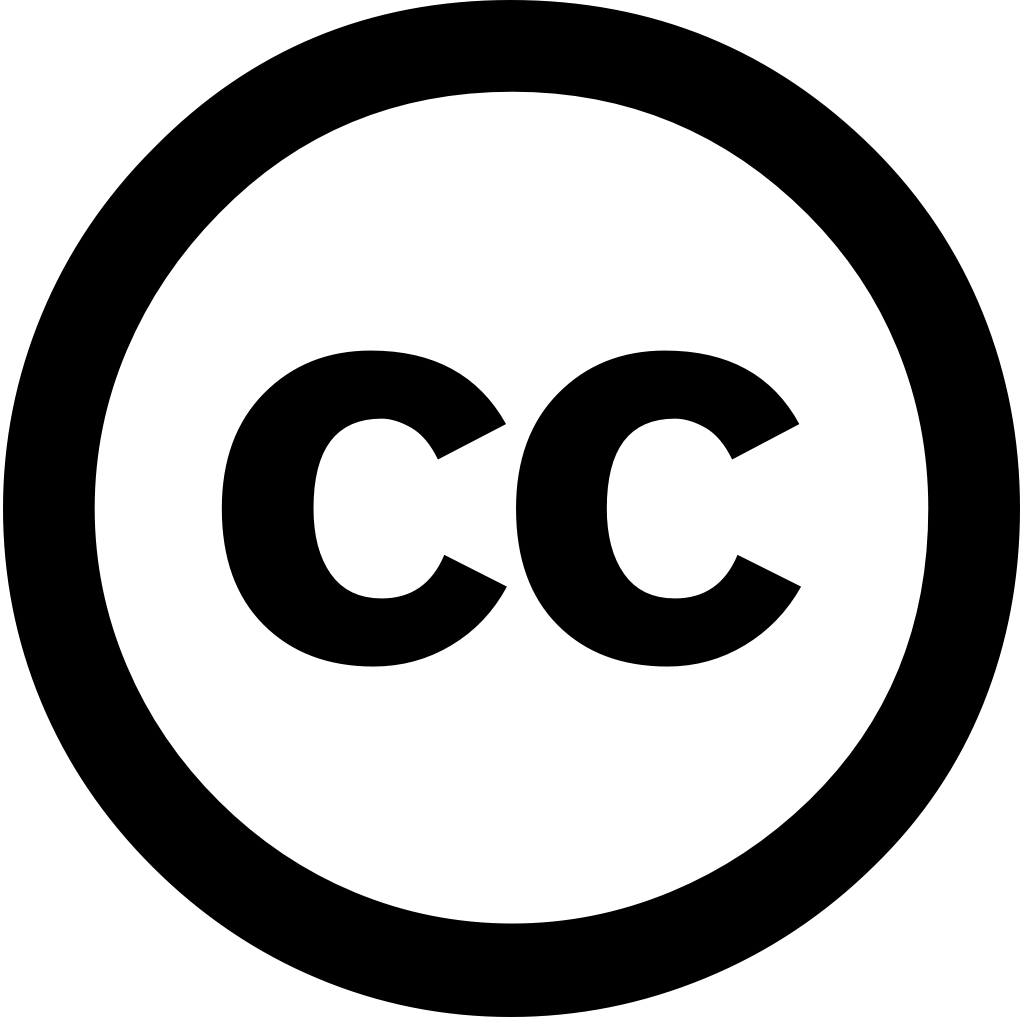
IEEE Access, Год журнала: 2024, Номер 12, С. 180481 - 180519
Опубликована: Янв. 1, 2024
Язык: Английский
IEEE Access, Год журнала: 2024, Номер 12, С. 180481 - 180519
Опубликована: Янв. 1, 2024
Язык: Английский
Bioengineering, Год журнала: 2025, Номер 12(3), С. 300 - 300
Опубликована: Март 16, 2025
Protecting people at work and leisure, improving their quality of life, is one the major challenges faced in this century [...]
Язык: Английский
Процитировано
0International Journal of Low-Carbon Technologies, Год журнала: 2024, Номер 19, С. 1368 - 1379
Опубликована: Янв. 1, 2024
Abstract With the urgency of mitigating global warming, low-carbon transformation power grid systems has emerged as a pivotal industry upgrade for sustainable development. We proposed novel deep neural network-based approach investment decision planning in grids, which aimed to address multidimensional key indicators related and provided reliable electricity layouts plans system decisions. To achieve this, three targeted branch models were established, encompassing behavior, production consumption, predictions new capacity investment. These effectively tackled challenges associated with distribution, price scheduling, carbon quotas, feasibility generation technologies. Subsequently, model was constructed, employing spatiotemporal networks recurrent networks, integrated aforementioned incorporated existing data. A comparative analysis conducted, examining predicted results against actual values from perspectives: portfolio, economy, overall plans. The demonstrated effectiveness our method accurately predicting future installed diverse technologies, sustainability indices, returns. Notably, achieves an impressive forecasting accuracy over 90% compared past 4 years.
Язык: Английский
Процитировано
2Опубликована: Сен. 22, 2024
Язык: Английский
Процитировано
1International Journal of Low-Carbon Technologies, Год журнала: 2024, Номер 19, С. 1968 - 1980
Опубликована: Янв. 1, 2024
Abstract Energy imbalance in electric vehicle energy storage battery packs poses a challenge due to design and usage variations. Traditional balancing control algorithms struggle cope with large-scale data complex nonlinear relationship modeling, which jeopardizes the stability of systems. To overcome this issue, we propose reinforcement learning (RL)-based strategy for pack control. Our approach begins adaptive modeling followed by employment an active determine duration charge state rank strength individual cells. Subsequently, RL network is employed learn dynamic parameters that capture variations, enabling subsequent automatic prediction effective strategies while simultaneously selecting optimal policy. simulation experiments demonstrate our ensures orderly discharge process cells, achieving impressive balance efficiency 91% when compared other similar methods. Furthermore, optimization methods results significant improvements efficiency, stability, operational costs. Notably, method also outperforms terms utilization rates, establishing its superiority category.
Язык: Английский
Процитировано
0International Journal of Low-Carbon Technologies, Год журнала: 2024, Номер 19, С. 2299 - 2308
Опубликована: Янв. 1, 2024
Abstract The inherent variability and uncertainty of distributed wind power generation exert profound impact on the stability equilibrium storage systems. In response to this challenge, we present a pioneering methodology for allocation capacities in integration storage. Firstly, introduce meticulously designed modeling technique aimed at optimizing forecasting deviations, thus augmenting controllability variations. Subsequently, establish cutting-edge real-time dynamic optimization model state charge, which effectively mitigates fluctuations associated with grid-connected power. Moreover, employ state-of-the-art robust algorithm enhance system. Through comprehensive simulation testing, our findings unequivocally demonstrate efficacy approach preserving harmonious balance between load output demand, thereby assuring unwavering operation entire Notably, attains an exceptional capacity efficiency 91% rigorous grid-smoothing test, outperforming comparable methodologies. Lastly, proffer essential recommendations pertaining attenuation effective level batteries, safeguarding long-term energy
Язык: Английский
Процитировано
0International Journal of Low-Carbon Technologies, Год журнала: 2024, Номер 19, С. 2363 - 2374
Опубликована: Янв. 1, 2024
Abstract In light of the pressing need to mitigate carbon emissions and safeguard environment, imperative imbuing landscape planning with low-carbon principles has taken center stage. This investigative endeavor embarks on a pioneering trajectory by presenting groundbreaking methodology for planning, employing twin long short-term memory (LSTM) model. innovative approach endeavors seamlessly amalgamate urban development practices. Initially, we lay foundation introducing an all-encompassing big data framework, which serves as bedrock processing historical environmental within domain. Subsequently, advocate adoption LSTM model, proficiently anticipates dynamic trajectories environments. Moreover, ascertain that optimization feature correlations in process culminates formulation bespoke strategies tailored planning. The empirical evidence substantiates superiority our approach, surpassing analogous algorithms both performance scope. Furthermore, holistic diligently accounts far-reaching ramifications socio-economic factors, ecological considerations, cultural landscapes. comprehensive vantage point augments efficacy durability initiatives, thereby shepherding landscapes towards realm characterized practices sustainable construction.
Язык: Английский
Процитировано
0IEEE Access, Год журнала: 2024, Номер 12, С. 180481 - 180519
Опубликована: Янв. 1, 2024
Язык: Английский
Процитировано
0