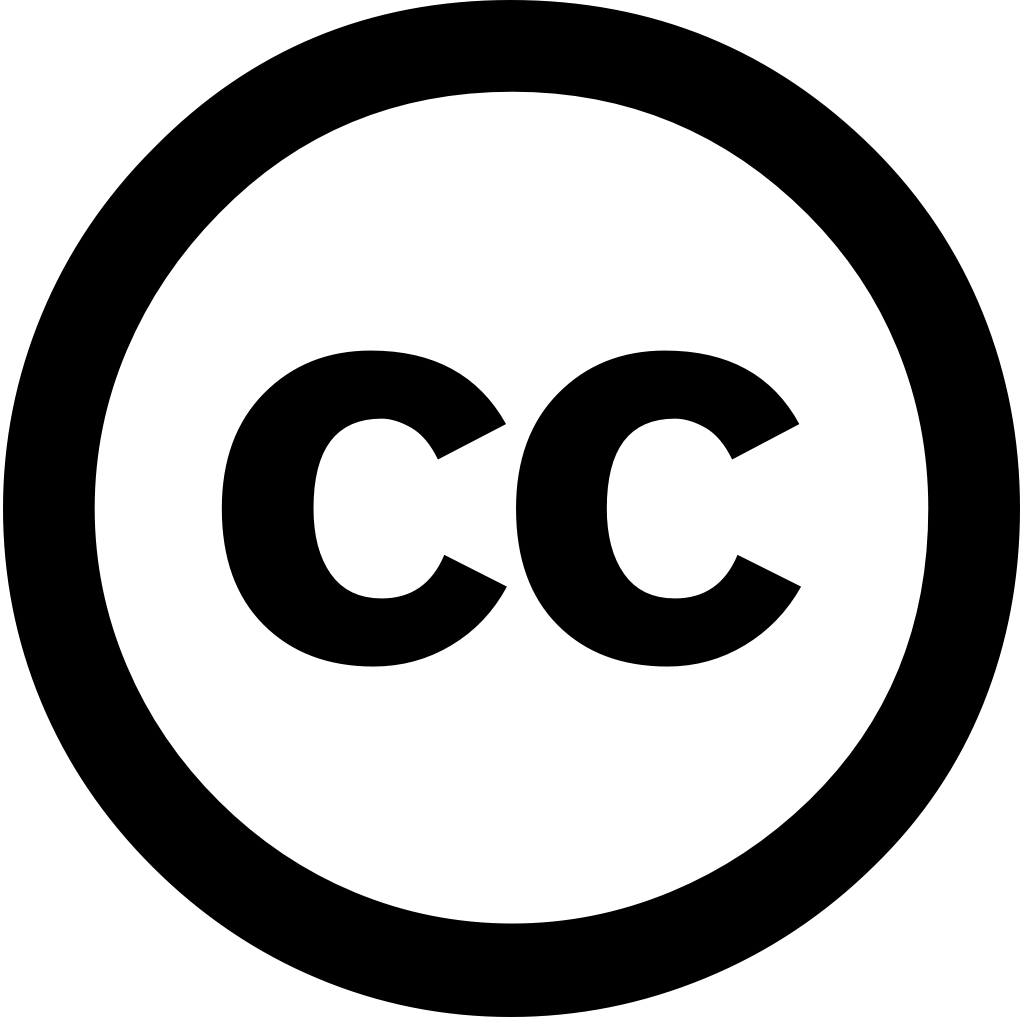
Bioengineering, Год журнала: 2025, Номер 12(1), С. 73 - 73
Опубликована: Янв. 15, 2025
Breast cancer ranks as the second most prevalent globally and is frequently diagnosed among women; therefore, early, automated, precise detection essential. Most AI-based techniques for breast are complex have high computational costs. Hence, to overcome this challenge, we presented innovative LightweightUNet hybrid deep learning (DL) classifier accurate classification of cancer. The proposed model boasts a low cost due its smaller number layers in architecture, adaptive nature stems from use depth-wise separable convolution. We employed multimodal approach validate model’s performance, using 13,000 images two distinct modalities: mammogram imaging (MGI) ultrasound (USI). collected datasets seven different sources, including benchmark DDSM, MIAS, INbreast, BrEaST, BUSI, Thammasat, HMSS. Since various resized them uniform size 256 × pixels normalized Box-Cox transformation technique. USI dataset smaller, applied StyleGAN3 generate 10,000 synthetic images. In work, performed separate experiments: first on real without augmentation + GAN-augmented our method. During experiments, used 5-fold cross-validation method, obtained good results (87.16% precision, 86.87% recall, 86.84% F1-score, accuracy) adding any extra data. Similarly, experiment provides better performance (96.36% 96.35% accuracy). This approach, which utilizes LightweightUNet, enhances by 9.20% 9.48% 9.51% increase accuracy combined dataset. works very well thanks creative network design, fake data, training These show that has lot potential clinical settings.
Язык: Английский