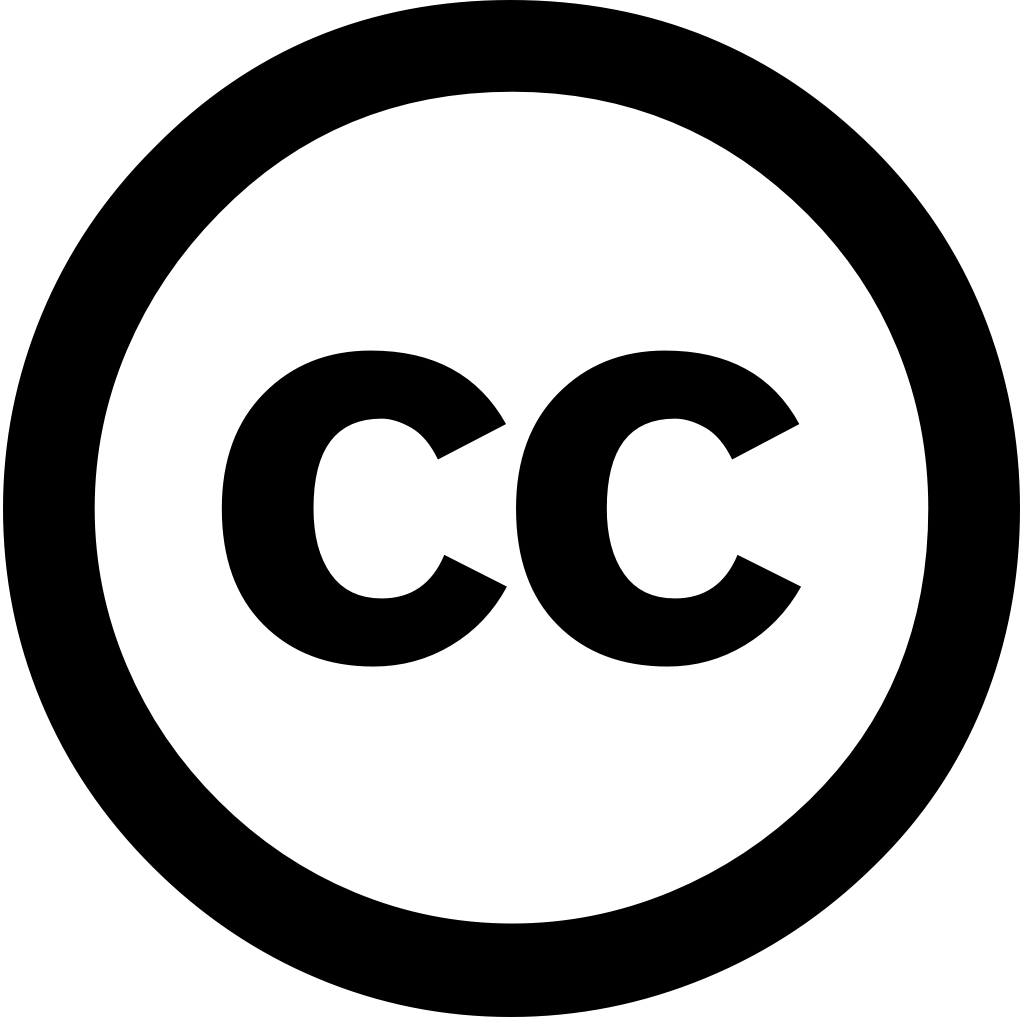
Patterns, Год журнала: 2025, Номер unknown, С. 101240 - 101240
Опубликована: Апрель 1, 2025
Язык: Английский
Patterns, Год журнала: 2025, Номер unknown, С. 101240 - 101240
Опубликована: Апрель 1, 2025
Язык: Английский
Algorithms, Год журнала: 2025, Номер 18(2), С. 77 - 77
Опубликована: Фев. 1, 2025
The brain acts as the body’s central command, overseeing diverse functions including thought, memory, speech, movement, and regulation of various organs. When healthy, seamlessly automatically; however, disruptions can lead to serious conditions such Alzheimer’s Disease, Brain Cancer, Stroke, Epilepsy. Epilepsy, a neurological disorder marked by recurrent seizures, results from irregular electrical activity in brain. These which strain both patients neurologists, are characterized symptoms like loss awareness, unusual behavior, confusion. This study presents an efficient EEG-based epileptic seizure detection framework utilizing hybrid Convolutional Neural Network (CNN), Long Short-Term Memory (LSTM), Gated Recurrent Unit (GRU) models approach support automated accurate diagnosis. Handling imbalanced EEG data, otherwise bias model outcomes reduce predictive accuracy, is key focus. Experimental indicate that proposed generally outperforms other Deep Learning Machine techniques with highest accuracy at 99.13%. Likewise, Explainable Artificial Intelligence (XAI) called SHAP (SHapley Additive exPlanations) utilized analyze improve interpretability medical decision-making. aligns objectives Medical Internet Things (MIoT), advancing smart applications services for effective detection.
Язык: Английский
Процитировано
2Diagnostics, Год журнала: 2025, Номер 15(5), С. 639 - 639
Опубликована: Март 6, 2025
Background/Objectives: Brain tumors are among the most aggressive diseases, significantly contributing to human mortality. Typically, classification of brain is performed through a biopsy, which often delayed until surgery necessary. An automated image technique crucial for accelerating diagnosis, reducing need invasive procedures and minimizing risk manual diagnostic errors being made by radiologists. Additionally, security sensitive MRI images remains major concern, with robust encryption methods required protect patient data from unauthorized access breaches in Medical Internet Things (MIoT) systems. Methods: This study proposes secure system that integrates chaotic Arnold techniques hybrid deep learning models using VGG16 neural network (DNN). The methodology ensures confidentiality while enabling accurate not compromising performance. Results: proposed demonstrated high performance under both scenarios. For encryption, it achieved an accuracy 93.75%, precision 94.38%, recall F-score 93.67%. model attained 94.1%, 96.9%, 96.6%. These results indicate encrypted can still be effectively classified, ensuring accuracy. Conclusions: approach provides secure, accurate, efficient solution tumor detection MIoT-based healthcare applications. By encrypting before classification, maintaining empower radiologists professionals worldwide, early diagnosis without procedures.
Язык: Английский
Процитировано
0Algorithms, Год журнала: 2025, Номер 18(3), С. 163 - 163
Опубликована: Март 13, 2025
With the advancements in deep learning methods, AI systems now perform at same or higher level than human intelligence many complex real-world problems. The data and algorithmic opacity of models, however, make task comprehending input information, model, model’s decisions quite challenging. This lack transparency constitutes both a practical an ethical issue. For present study, it is major drawback to deployment methods mandated with detecting patterns prognosticating Alzheimer’s disease. Many approaches presented medical literature for overcoming this critical weakness are sometimes cost sacrificing accuracy interpretability. study attempt addressing challenge fostering reliability AI-driven healthcare solutions. explores few commonly used perturbation-based interpretability (LIME) gradient-based (Saliency Grad-CAM) visualizing explaining dataset, MRI image-based disease identification using diagnostic predictive strengths ensemble framework comprising Convolutional Neural Networks (CNNs) architectures (Custom multi-classifier CNN, VGG-19, ResNet, MobileNet, EfficientNet, DenseNet), Vision Transformer (ViT). experimental results show stacking achieving remarkable 98.0% while hard voting reached 97.0%. findings valuable contribution growing field explainable artificial (XAI) imaging, helping end users researchers gain understanding backstory behind image dataset decisions.
Язык: Английский
Процитировано
0Neural Computing and Applications, Год журнала: 2025, Номер unknown
Опубликована: Март 15, 2025
Язык: Английский
Процитировано
0Bioengineering, Год журнала: 2025, Номер 12(4), С. 356 - 356
Опубликована: Март 29, 2025
According to recent global public health studies, chronic kidney disease (CKD) is becoming more and recognized as a serious risk many people are suffering from this disease. Machine learning techniques have demonstrated high efficiency in identifying CKD, but their opaque decision-making processes limit adoption clinical settings. To address this, study employs generative adversarial network (GAN) handle missing values CKD datasets utilizes few-shot techniques, such prototypical networks model-agnostic meta-learning (MAML), combined with explainable machine predict CKD. Additionally, traditional models, including support vector machines (SVM), logistic regression (LR), decision trees (DT), random forests (RF), voting ensemble (VEL), applied for comparison. unravel the “black box” nature of predictions, various AI, SHapley Additive exPlanations (SHAP) local interpretable explanations (LIME), understand predictions made by model, thereby contributing process significant parameters diagnosis Model performance evaluated using predefined metrics, results indicate that models integrated GANs significantly outperform techniques. Prototypical achieve highest accuracy 99.99%, while MAML reaches 99.92%. Furthermore, attain F1-score, recall, precision, Matthews correlation coefficient (MCC) 99.89%, 99.9%, 100%, respectively, on raw dataset. As result, experimental clearly demonstrate effectiveness suggested method, offering reliable trustworthy model classify This framework supports objectives Medical Internet Things (MIoT) enhancing smart medical applications services, enabling accurate prediction detection facilitating optimal making.
Язык: Английский
Процитировано
0Diagnostics, Год журнала: 2025, Номер 15(8), С. 976 - 976
Опубликована: Апрель 11, 2025
Background and Objective: Using echocardiogram data for cardiovascular disease (CVD) can lead to difficulties due imbalanced datasets, leading biased predictions. Machine learning models enhance prognosis accuracy, but their effectiveness is influenced by optimal feature selection robust classification techniques. This study introduces an event-based self-similarity approach automatic data. Critical features correlated with progression were identified leveraging patterns. used dataset, visual presentations of high-frequency sound wave signals, patients heart who are treated using three treatment methods: catheter ablation, ventricular defibrillator, drug control—over the course years. Methods: The dataset was classified into nine categories Recursive Feature Elimination (RFE) applied identify most relevant features, reducing model complexity while maintaining diagnostic accuracy. models, including XGBoost CATBoost, trained evaluated. Results: Both achieved comparable accuracy values, 84.3% 88.4%, respectively, under different normalization To further optimize performance, combined a voting ensemble, improving predictive Four essential features—age, aorta (AO), left (LV), atrium (LA)—were as critical found in Random Forest (RF)-voting ensemble classifier. results underscore importance techniques handling robustness, bias automated systems. Conclusions: Our findings highlight potential machine learning-driven analysis patient care providing accurate, data-driven assessments.
Язык: Английский
Процитировано
0Patterns, Год журнала: 2025, Номер unknown, С. 101240 - 101240
Опубликована: Апрель 1, 2025
Язык: Английский
Процитировано
0