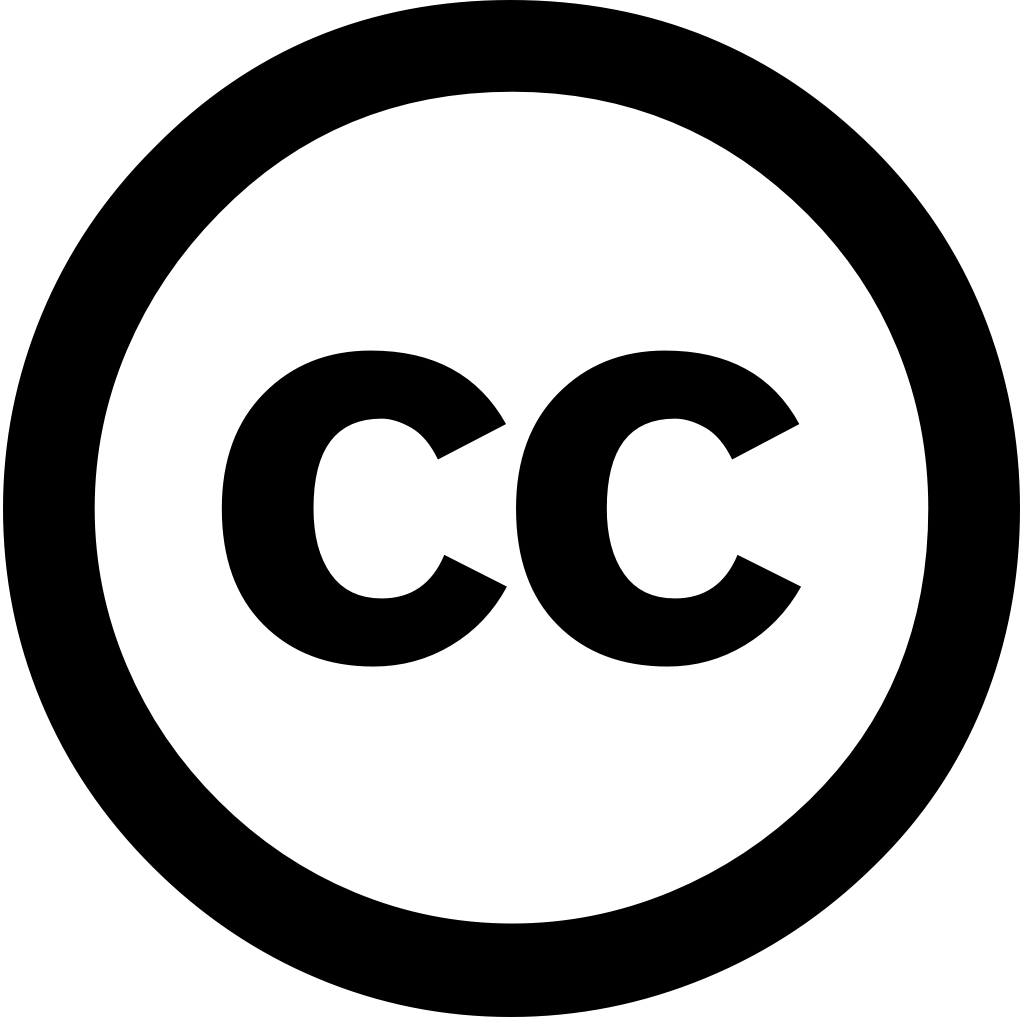
Scientific Reports, Год журнала: 2025, Номер 15(1)
Опубликована: Апрель 26, 2025
A methodology based on Principal Component Analysis (PCA) and machine learning (ML) regression was developed in this study for predicting 5-aminosalicylic acid drug release from polysaccharide-coated formulation. The Raman method used collection of spectral data which were then as inputs to the ML models estimation release. For modeling, we examined predictive accuracy three models-Elastic Net (EN), Group Ridge Regression (GRR), Multilayer Perceptron (MLP)-for forecasting behavior samples. dataset, consisting 155 points with over 1500 features, underwent preprocessing involving normalization, dimensionality reduction, outlier detection using Cook's Distance. Model hyperparameters tuned Slime Mould Algorithm (SMA), each model's performance evaluated through k-fold cross-validation (k = 3). Assessment metrics, such coefficient determination (R²), root mean square error (RMSE), absolute (MAE), emphasize MLP exceptional performance. On test set, achieved an R² 0.9989, notably higher than EN's 0.9760 GRR's 0.7137. Additionally, exhibited remarkably low RMSE MAE values at 0.0084 0.0067, respectively, comparison 0.0342 0.0267, well 0.0907 0.0744. Parity plots curves further validate MLP's reliability, demonstrating close alignment between actual predicted efficient minimal overfitting. Consequently, model emerges most effective approach task, offering a robust tool accurately modeling complex data. These findings underscore robustness model, providing reliable formulations, implications advancing colonic delivery systems.
Язык: Английский