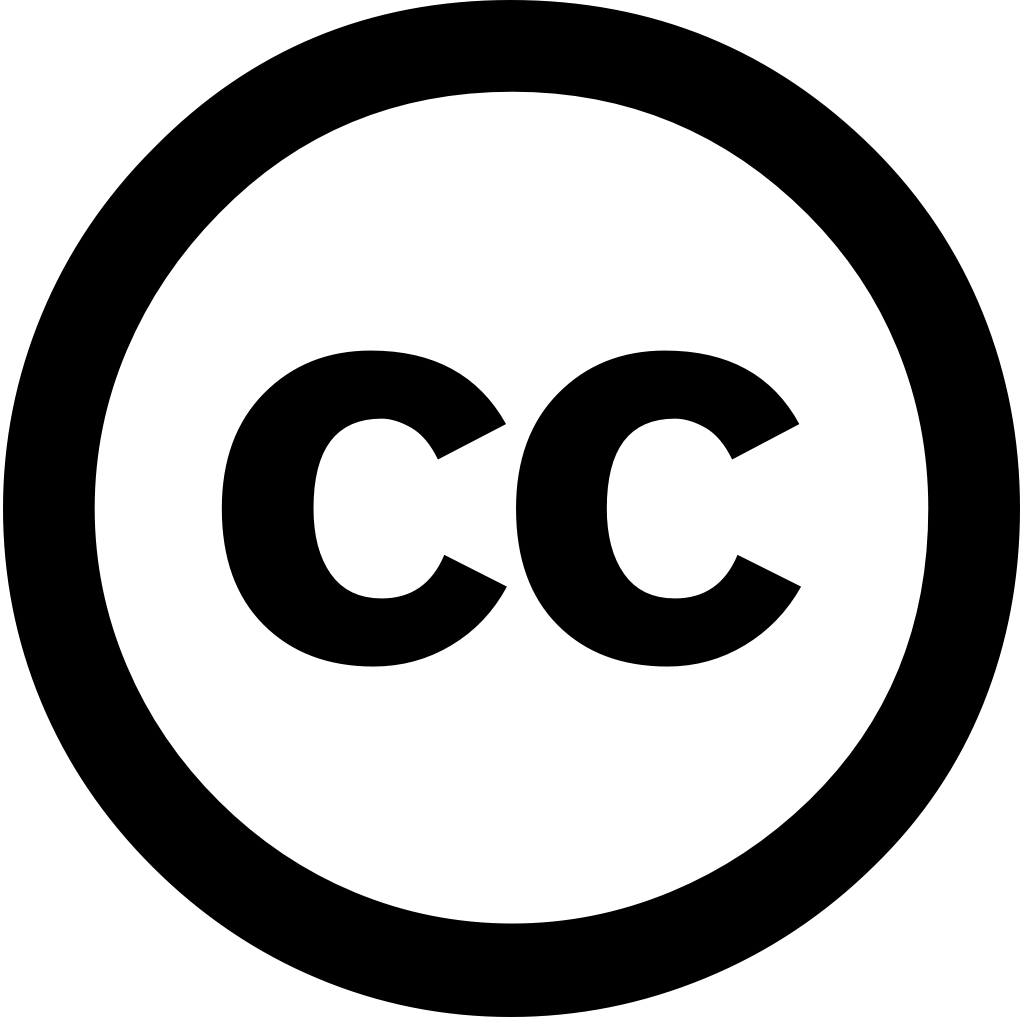
Computation, Год журнала: 2025, Номер 13(3), С. 61 - 61
Опубликована: Март 2, 2025
Fatigue has always been one of the major causes structural failure, where repeated loading and unloading cycles reduce fracture energy material, causing it to fail at stresses lower than its monotonic strength. However, predicting fatigue life is a highly challenging task and, in this context, present study proposes fundamentally new hybrid physics-informed data-driven approach. Firstly, an energy-based model developed simulate behavior concrete under compressive cyclic loading. The data generated from these numerical simulations are then utilized train machine learning (ML) models. stress–strain curve S-N compression, obtained model, validated against experimental data. For ML models, two different algorithms used as follows: k-Nearest Neighbors (KNN) Deep Neural Networks (DNN), total 1962 instances for training testing Furthermore, performance models evaluated out-of-range inputs, DNN with three hidden layers (a complex 128, 64, 32 neurons) provides best predictions, only 0.6% overall error.
Язык: Английский