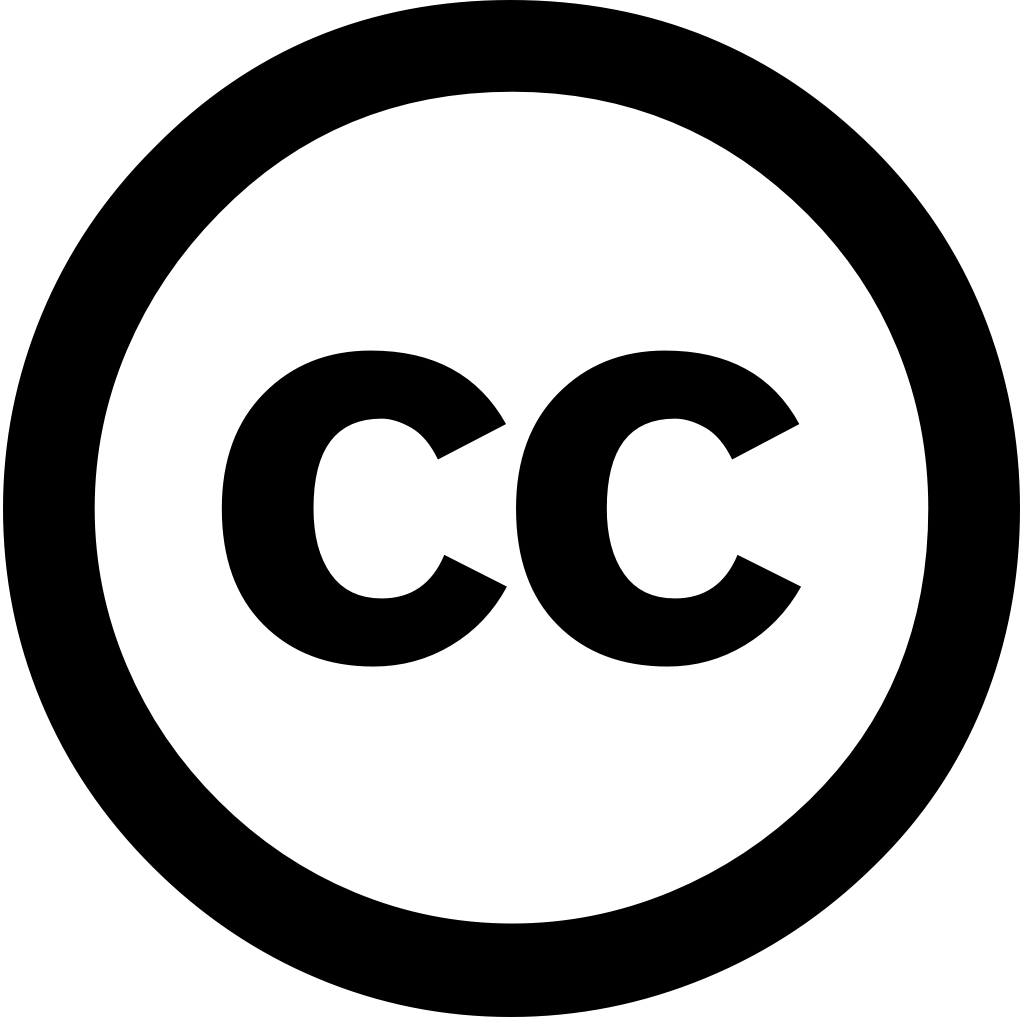
arXiv (Cornell University), Год журнала: 2023, Номер unknown
Опубликована: Янв. 1, 2023
Assigning repetitive and physically-demanding construction tasks to robots can alleviate human workers's exposure occupational injuries. Transferring necessary dexterous adaptive artisanal craft skills from workers is crucial for the successful delegation of achieving high-quality robot-constructed work. Predefined motion planning scripts tend generate rigid collision-prone robotic behaviors in unstructured site environments. In contrast, Imitation Learning (IL) offers a more robust flexible skill transfer scheme. However, majority IL algorithms rely on repeatedly demonstrate task performance at full scale, which be counterproductive infeasible case To address this concern, paper proposes an immersive, cloud robotics-based virtual demonstration framework that serves two primary purposes. First, it digitalizes process, eliminating need physical manipulation heavy objects. Second, employs federated collection reusable demonstrations are transferable similar future thus reduce requirement illustration by agents. Additionally, enhance trustworthiness, explainability, ethical soundness robot training, utilizes Hierarchical (HIL) model decompose into sequential reactive sub-skills. These layers represented deep generative models, enabling control actions. By delegating strains work human-trained robots, promotes inclusion with diverse capabilities educational backgrounds within industry.
Язык: Английский