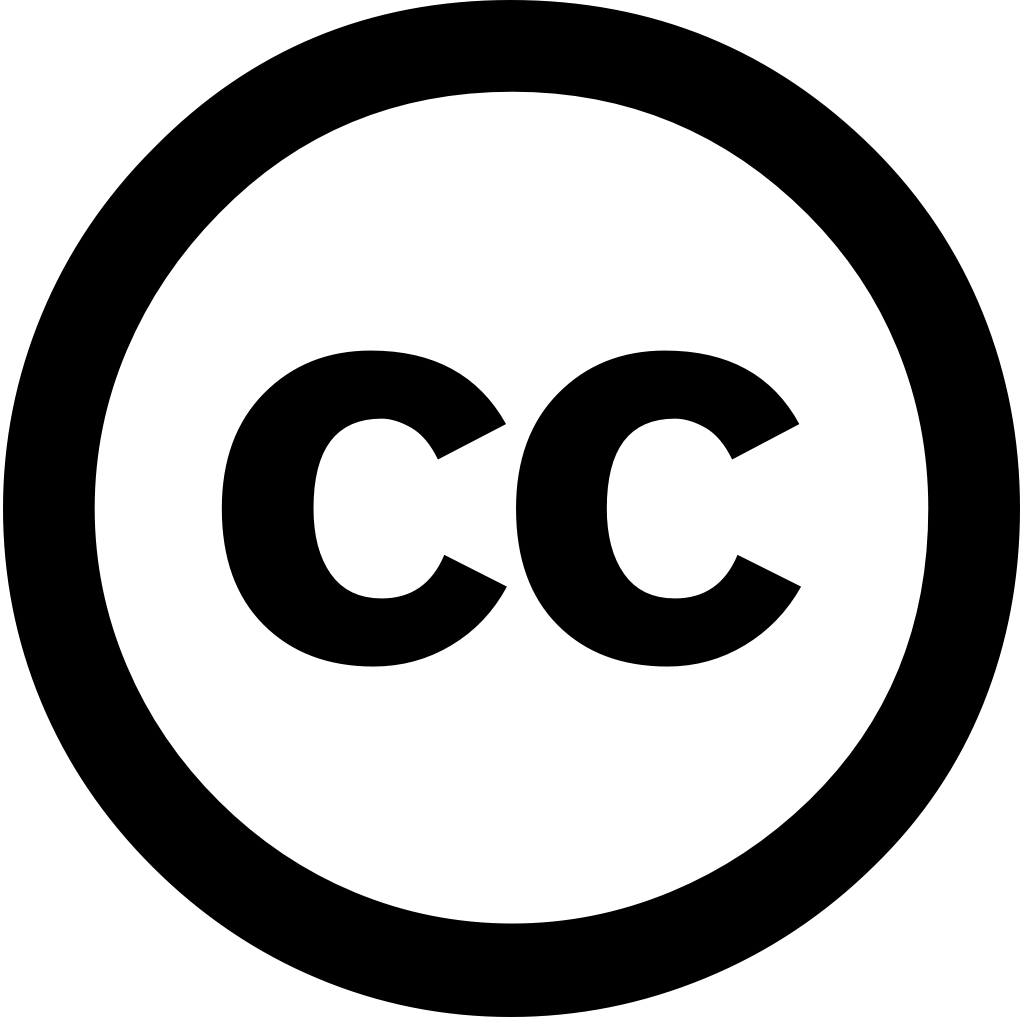
Discover Oncology, Год журнала: 2025, Номер 16(1)
Опубликована: Март 8, 2025
Endometrial cancer represents a significant health challenge, with rising incidence and complex prognostic challenges. This study aimed to develop robust predictive model integrating programmed cell death-related genes advanced machine learning techniques. Utilizing transcriptomic data from TCGA-UCEC GSE119041 datasets, we employed comprehensive approach involving 117 algorithms. Key methodologies included differential gene expression analysis, weighted co-expression network functional enrichment studies, immune landscape evaluation, multi-dimensional risk stratification. We identified 10 critical (PTGIS, TIMP3, SRPX, SNCA, HIC1, BAK1, STXBP2, TRIB3, RTKN2, E2F1) constructed superior performance. The StepCox[forward] + plsRcox algorithm combination demonstrated excellent accuracy (AUC > 0.8). Kaplan–Meier analysis revealed survival differences between high- low-risk groups in both training (HR = 3.37, p < 0.001) validation cohorts 2.05, 0.021). showed strong correlations clinical characteristics, infiltration patterns, potential therapeutic responses. presents novel, endometrial prognosis, molecular insights provide more precise stratification tool translation.
Язык: Английский