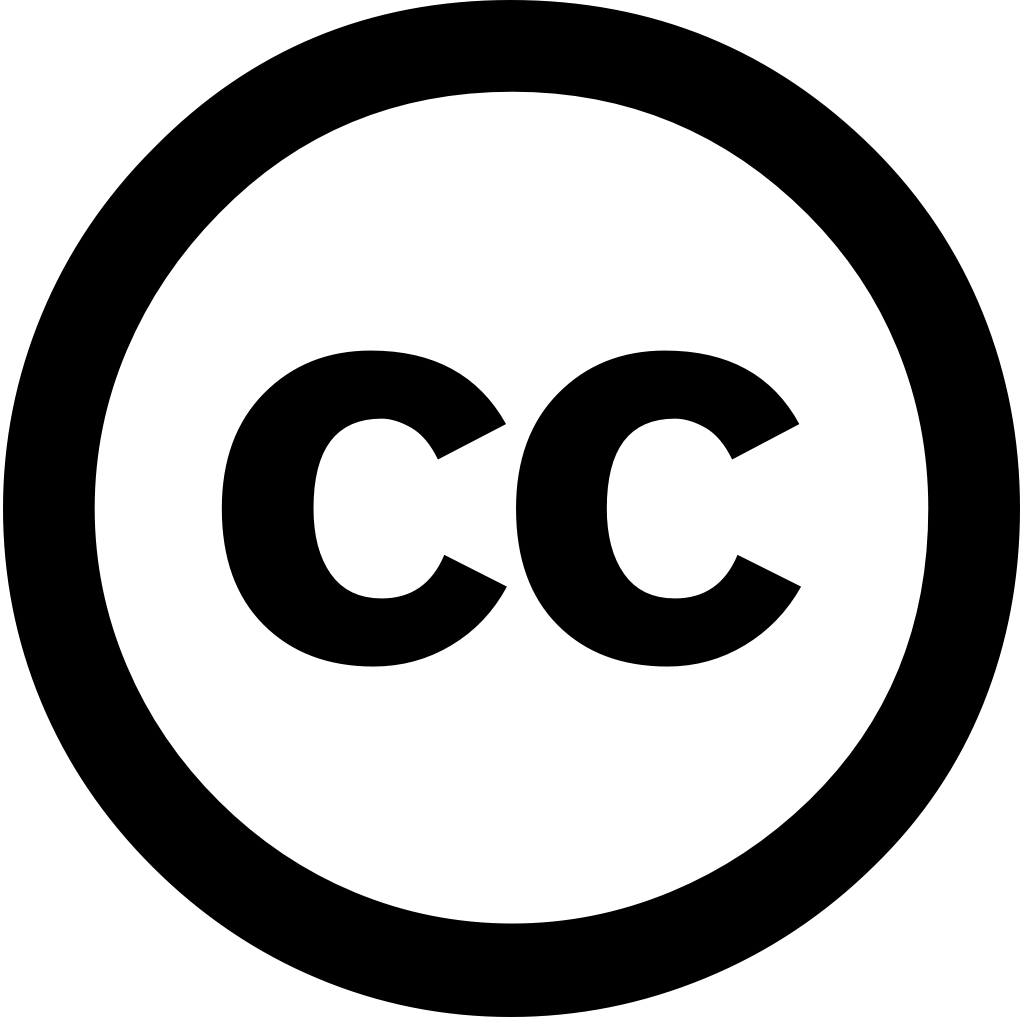
Опубликована: Сен. 25, 2024
Язык: Английский
Опубликована: Сен. 25, 2024
Язык: Английский
ISPRS Journal of Photogrammetry and Remote Sensing, Год журнала: 2024, Номер 211, С. 262 - 280
Опубликована: Апрель 17, 2024
Язык: Английский
Процитировано
13Remote Sensing, Год журнала: 2024, Номер 16(3), С. 446 - 446
Опубликована: Янв. 23, 2024
Since 1971, remote sensing techniques have been used to map and monitor phenomena parameters of the coastal zone. However, updated reviews only considered one phenomenon, parameter, data source, platform, or geographic region. No review has offered an overview that can be accurately mapped monitored with data. This systematic was performed achieve this purpose. A total 15,141 papers published from January 2021 June 2023 were identified. The 1475 most cited screened, 502 eligible included. Web Science Scopus databases searched using all possible combinations between two groups keywords: geographical names in areas platforms. demonstrated that, date, many (103) (39) (e.g., coastline land use cover changes, climate change, urban sprawl). Moreover, authors validated 91% retrieved parameters, 39 1158 times (88% combined together other parameters), 75% over time, 69% several compared results each available products. They obtained 48% different methods, their 17% GIS model techniques. In conclusion, addressed requirements needed more effectively analyze employing integrated approaches: they data, merged
Язык: Английский
Процитировано
11Remote Sensing, Год журнала: 2023, Номер 15(16), С. 4068 - 4068
Опубликована: Авг. 17, 2023
Marine heatwaves (MHWs) are extreme events characterized by abnormally high sea surface temperatures, and they have significant impacts on marine ecosystems human society. The rapid accurate forecasting of MHWs is crucial for preventing responding to the can lead to. However, research relevant methods limited, a dedicated system specifically tailored South China Sea (SCS) region has yet be reported. This study proposes novel utilizing U-Net ConvLSTM models predict in SCS. Specifically, model used forecast intensity MHWs, while employed probability their occurrence. indication an MHW relies both forecasted exceeding threshold T occurrence predicted surpassing P. Incorporating sensitivity analysis, optimal thresholds determined as 0.9 °C, 0.8 1.0 °C 1-, 3-, 5-, 7-day times, respectively. Similarly, P identified 0.29, 0.30, 0.20, 0.28. Employing these yields highest accuracy rates 0.92, 0.89, 0.88, 0.87 corresponding times. innovative approach gives better predictions SCS, providing invaluable reference information management authorities make well-informed decisions issue timely warnings.
Язык: Английский
Процитировано
22Frontiers in Earth Science, Год журнала: 2023, Номер 11
Опубликована: Фев. 16, 2023
Utilization and exploitation of marine resources by humans have contributed to the growth research. As technology progresses, artificial intelligence (AI) approaches are progressively being applied maritime research, complementing traditional forecasting models observation techniques some degree. This article takes algorithmic model as its starting point, references several application trials, methodically elaborates on emerging research trend mixing machine learning physical modeling concepts. discusses evolution methodologies for building ocean observations, remote sensing satellites, smart sensors, intelligent underwater robots, construction big data. We also cover method identifying internal waves (IW), heatwaves, El Niño-Southern Oscillation (ENSO), sea ice using algorithms. In addition, we analyze applications in prediction components, including physics-driven numerical models, model-driven statistical data-driven deep combined with models. review shows routes observation, phenomena identification, elements forecasting, examples forecasts their future development trends from angles points view, categorizing various uses sector.
Язык: Английский
Процитировано
19The Science of The Total Environment, Год журнала: 2024, Номер 922, С. 171009 - 171009
Опубликована: Фев. 24, 2024
Язык: Английский
Процитировано
7PFG – Journal of Photogrammetry Remote Sensing and Geoinformation Science, Год журнала: 2025, Номер unknown
Опубликована: Фев. 13, 2025
Язык: Английский
Процитировано
0Journal of Marine Science and Engineering, Год журнала: 2025, Номер 13(2), С. 359 - 359
Опубликована: Фев. 15, 2025
Monitoring bottom dissolved oxygen (DO) is crucial for understanding hypoxia, a threat to marine ecosystems and fisheries. However, traditional observations are limited in spatiotemporal coverage, while numerical models consume tremendous computing resources. This study develops an interpretable machine learning framework simulate the DO distribution on East China Sea (ECS) shelf explore its potential driving mechanisms. By integrating remote sensing, situ observations, model outputs, generates high-resolution (4 km) estimates from 1998 2024. Validation against independent datasets confirms improved accuracy spatial resolution, with RMSE below 1 mg/L. The results reveal persistent decline DO, strongly linked rising sea surface temperature (SST), underscoring role of warming water deoxygenation. Model interpretability further identifies SST bathymetry as key factors. provides robust tool assessing trends, their ecological impacts, supporting future monitoring management ECS shelf.
Язык: Английский
Процитировано
0Water, Год журнала: 2025, Номер 17(6), С. 780 - 780
Опубликована: Март 7, 2025
With the planning and construction of marine ranching in China, water quality has become one critical limiting factors for development ranching. Due to geographical differences, ranches exhibit varying conditions under influence continental shelf. To best our knowledge, there is limited research on satellite-based monitoring spatiotemporal variations different locations. Chlorophyll-a (Chl-a) a key indicator ecological health disaster prevention capacity ranching, as it reflects eutrophication crucial high-quality, sustainable operation Using physically based model, this study focuses retrieval Chl-a concentration Daya Bay. The coefficient determination (R2) between model values situ data 0.69, with root mean square error (RMSE) 1.52 μg/L absolute percentage (MAPE) 44.25%. Seasonal are observed Bay higher spring–summer lower autumn–winter. In YangMeikeng waters, shows declining trend A comparison (nearshore) XiaoXingshan (offshore) suggests that offshore may be less impacted by terrestrial pollutants. primary sources input Dan’ao River aquaculture areas northeastern part bay. This can provide valuable information protection management
Язык: Английский
Процитировано
0Ömer Halisdemir Üniversitesi Mühendislik Bilimleri Dergisi, Год журнала: 2025, Номер 14(2), С. 615 - 629
Опубликована: Апрель 15, 2025
Water is essential for the sustainability of life and healthy functioning ecosystems. Increasing pollution poses a serious threat to world's waters, making monitoring protection water quality strategic imperative. Chlorophyll-a one most important indicators ecosystem health, as it measure photosynthetic activity phytoplankton density, lifeblood aquatic Remote sensed data provide unique opportunity analyse chlorophyll-a changes in lake In this study, concentration was modelled by machine deep learning techniques using measurements, Landsat-8 surface reflectance values spectral indices Lake Mogan between 2018 2024. The RF, ANN, CNN models achieved R² 0.84, 0.85, 0.92, respectively. With its ability learn relationships, identify patterns complex datasets, superior process remote sensing imagery, thematic maps were generated model, which performed best study. results study demonstrate potential sensing-based approaches chlorophyll-a. produce highly accurate results, provides literature with an effective tool future studies.
Язык: Английский
Процитировано
0Knowledge-Based Systems, Год журнала: 2024, Номер 301, С. 112279 - 112279
Опубликована: Июль 27, 2024
The increasing occurrence of Harmful Algal Blooms (HABs) in water systems poses significant challenges to ecological health, public safety, and economic stability globally. Deep Learning (DL) models, notably Convolutional Neural Networks (CNN) Long-Short Term Memory (LSTM), have been widely employed for HAB prediction. However, the emergence state-of-the-art multi-horizon forecasting DL architectures such as Basis Expansion Analysis Interpretable Time Series Forecasting (N-BEATS) provides a novel solution long-term This study compares performance N-BEATS with LSTM CNN models using high temporal granularity quality data from As Conchas reservoir (NW Spain) forecast chlorophyll-a (Chl-a) concentrations, key indicator HABs. evaluation encompasses one-day one-week prediction horizons, aligning World Health Organization (WHO) alert criteria. Results indicate that outperforms predictions when multiple consecutive days within week. Furthermore, augmenting input additional variables does not significantly enhance predictive accuracy, challenging assumption complexity always improves model performance. also explores transferability trained across different monitoring buoys same body, emphasizing adaptability broad applicability diverse aquatic environments. research underscores potential valuable tool prediction, particularly longer-term forecasting.
Язык: Английский
Процитировано
3