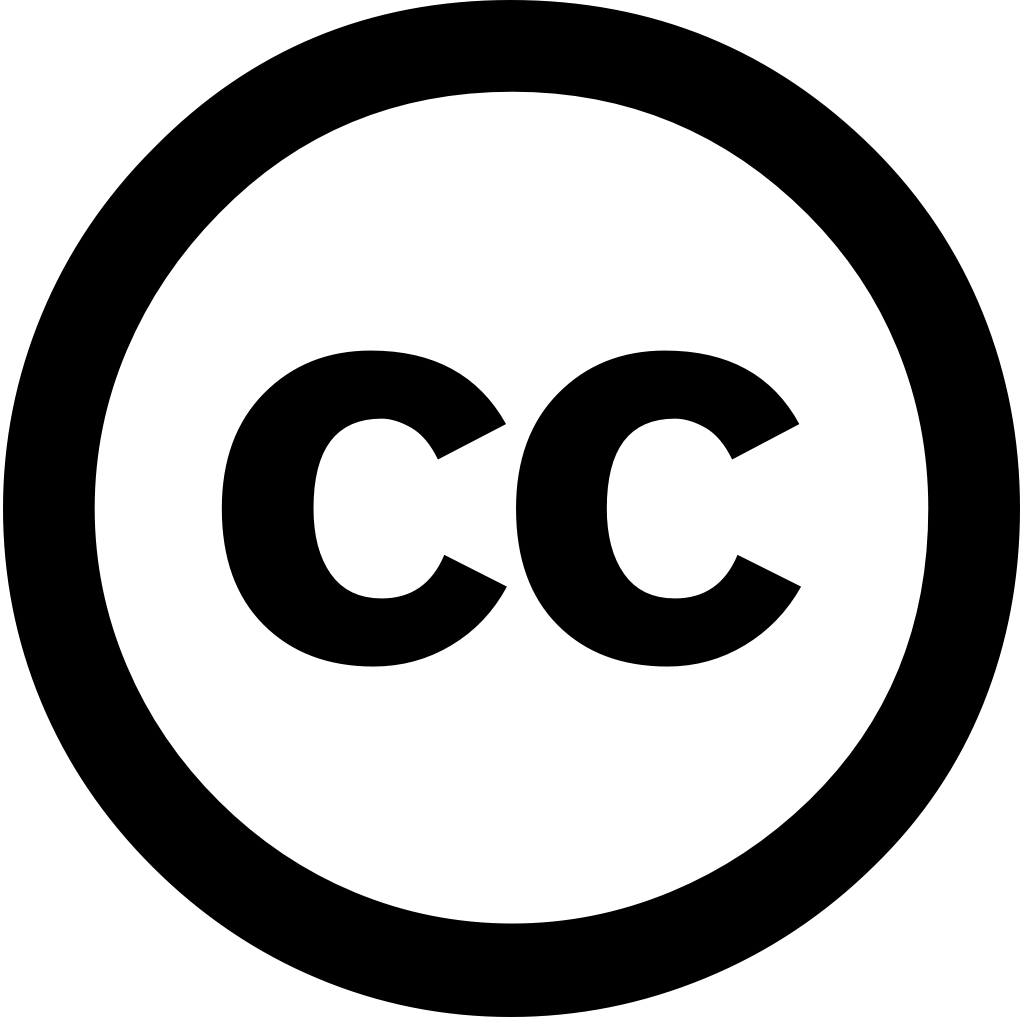
Research Square (Research Square), Год журнала: 2024, Номер unknown
Опубликована: Ноя. 15, 2024
Язык: Английский
Research Square (Research Square), Год журнала: 2024, Номер unknown
Опубликована: Ноя. 15, 2024
Язык: Английский
Water Resources Management, Год журнала: 2024, Номер 38(10), С. 3953 - 3972
Опубликована: Апрель 19, 2024
Язык: Английский
Процитировано
52Scientific Reports, Год журнала: 2025, Номер 15(1)
Опубликована: Янв. 22, 2025
Abstract Understanding snow and ice melt dynamics is vital for flood risk assessment effective water resource management in populated river basins sourced inaccessible high-mountains. This study provides an AI-enabled hybrid approach integrating glacio-hydrological model outputs (GSM-SOCONT), with different machine learning deep techniques framed as alternative ‘computational scenarios, leveraging both physical processes data-driven insights enhanced predictive capabilities. The standalone (CNN-LSTM), relying solely on meteorological data, outperformed its counterpart equivalents. Hybrid models (CNN-LSTM1 to CNN-LSTM15) were trained using data augmented representing snow-melt contributions streamflow. (CNN-LSTM14), only glacier-derived features, performed best high NSE (0.86), KGE (0.80), R (0.93) values during calibration, the highest (0.83), (0.88), (0.91), lowest RMSE (892) MAE (544) validation. Finally, a multi-scale analysis feature permutations was explored wavelet transformation theory, these into final (CNN-LSTM19), which significantly enhances accuracy, particularly high-flow events, evidenced by improved (from 0.83 0.97) reduced 892 442) comparative illustrates how AI-enhanced hydrological improve accuracy of runoff forecasting provide more reliable actionable managing resources mitigating risks - despite paucity direct measurements.
Язык: Английский
Процитировано
3Remote Sensing, Год журнала: 2024, Номер 16(6), С. 956 - 956
Опубликована: Март 8, 2024
Accurately simulating glacier mass balance (GMB) data is crucial for assessing the impacts of climate change on dynamics. Since physical models often face challenges in comprehensively accounting factors influencing glacial melt and uncertainties inputs, machine learning (ML) offers a viable alternative due to its robust flexibility nonlinear fitting capability. However, effectiveness ML modeling GMB across diverse types within High Mountain Asia has not yet been thoroughly explored. This study addresses this research gap by evaluating used simulation annual glacier-wide data, with specific focus comparing maritime glaciers Niyang River basin continental Manas basin. For purpose, meteorological predictive derived from monthly ERA5-Land datasets, topographical obtained Randolph Glacier Inventory, along target rooted geodetic observations, were employed drive four selective models: random forest model, gradient boosting decision tree (GBDT) deep neural network ordinary least-square linear regression model. The results highlighted that generally exhibit superior performance compared ones. Moreover, among models, GBDT model was found consistently coefficient determination (R2) values 0.72 0.67 root mean squared error (RMSE) 0.21 m w.e. 0.30 river basins, respectively. Furthermore, reveals climatic differentially influence simulations glaciers, providing key insights into dynamics response change. In summary, ML, particularly demonstrates significant potential simulation. application can enhance accuracy modeling, promising approach assess
Язык: Английский
Процитировано
8Remote Sensing, Год журнала: 2024, Номер 16(21), С. 3999 - 3999
Опубликована: Окт. 28, 2024
In order to anticipate residual errors and improve accuracy while reducing uncertainties, this work integrates the long short-term memory (LSTM) with Soil Water Assessment Tool (SWAT) create a deep learning (DL) model that is guided by physics. By forecasting of SWAT model, SWAT-informed LSTM (LSTM-SWAT) differs from typical approaches predict streamflow directly. Through numerical tests, performance LSTM-SWAT was evaluated both LSTM-only SWAT-only models in Upper Heihe River Basin. The outcomes showed performed better than other models, showing higher lower mean absolute error (MAE = 3.13 m3/s). Sensitivity experiments further how quality training dataset affects LSTM-SWAT. results study demonstrate may prediction greatly remote sensing situ observations. Additionally, emphasizes need for detailed consideration specific sources uncertainty predictive capabilities hybrid model.
Язык: Английский
Процитировано
5Journal of Hydrology, Год журнала: 2025, Номер unknown, С. 132779 - 132779
Опубликована: Фев. 1, 2025
Язык: Английский
Процитировано
0Journal of Hydrology Regional Studies, Год журнала: 2025, Номер 59, С. 102311 - 102311
Опубликована: Март 17, 2025
Язык: Английский
Процитировано
0Ecological Indicators, Год журнала: 2024, Номер 162, С. 112021 - 112021
Опубликована: Апрель 18, 2024
A profound comprehension of the relationship between built environment and waterlogging disasters in high-density urban areas is essential to improve resilience achieve safe sustainable living environments. Based on multi-source data, this study employs a spatial autocorrelation model, geodetector multi-scale geographically weighted regression model construct comprehensive method. This has led formulation more effective integration method for investigating non-stationary relationships disasters. The results show that: (1) distribution events displays strong dependence. (2) Floor area ratio, building structure index, slope, water surface rate, etc., exhibit limited independent explanatory capability concerning density events. However, their nonlinear augmentation effect becomes noteworthy upon amalgamation with other factors, especially combination characteristics number rain-sewage mixing nodes. (3) It reliable efficient reduce risk by optimizing rainwater sewage diversion system reducing nodes instead modifying underlying controlling morphological impervious ground. (4) Where demonstrates intricate diverse attributes, emergence heightened sensitivity changes environment. provides novel perspective understanding formation mechanisms heterogeneity
Язык: Английский
Процитировано
3Remote Sensing, Год журнала: 2024, Номер 16(19), С. 3723 - 3723
Опубликована: Окт. 7, 2024
Gross primary productivity (GPP) is vital for ecosystems and the global carbon cycle, serving as a sensitive indicator of ecosystems’ responses to climate change. However, impact future changes on GPP in Tibetan Plateau, an ecologically important climatically region, remains underexplored. This study aimed develop data-driven approach predict seasonal annual variations Plateau up year 2100 under changing climatic conditions. A convolutional neural network (CNN) was employed investigate relationships between various environmental factors, including variables, CO2 concentrations, terrain attributes. analyzed projected from Coupled Model Intercomparison Project Phase 6 (CMIP6) four scenarios: SSP1–2.6, SSP2–4.5, SSP3–7.0, SSP5–8.5. The results suggest that expected significantly increase throughout 21st century all scenarios. By 2100, reach 1011.98 Tg C, 1032.67 1044.35 1055.50 C scenarios, representing 0.36%, 4.02%, 5.55%, 5.67% relative 2021. analysis indicates spring autumn shows more pronounced growth SSP3–7.0 SSP5–8.5 scenarios due extended growing season. Furthermore, identified elevation band 3000 4500 m particularly change terms response. Significant increases would occur east Qilian Mountains upper reaches Yellow Yangtze Rivers. These findings highlight pivotal role driving dynamics this region. insights not only bridge existing knowledge gaps regarding over coming decades but also provide valuable guidance formulation adaptation strategies at ecological conservation management.
Язык: Английский
Процитировано
2Remote Sensing of Environment, Год журнала: 2024, Номер 315, С. 114437 - 114437
Опубликована: Окт. 17, 2024
Язык: Английский
Процитировано
2Natural Hazards, Год журнала: 2024, Номер unknown
Опубликована: Ноя. 4, 2024
Язык: Английский
Процитировано
1