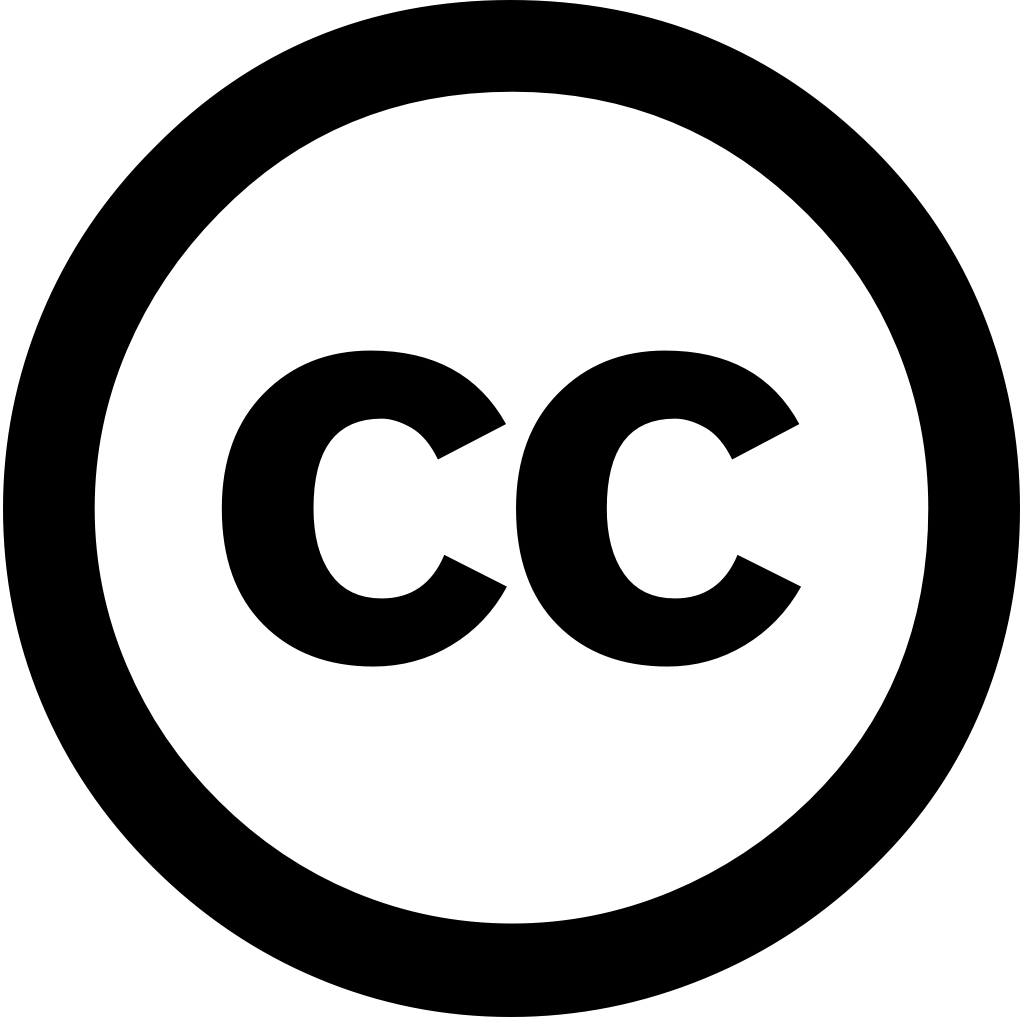
Scientific Reports, Год журнала: 2025, Номер 15(1)
Опубликована: Янв. 21, 2025
Язык: Английский
Scientific Reports, Год журнала: 2025, Номер 15(1)
Опубликована: Янв. 21, 2025
Язык: Английский
Cancers, Год журнала: 2023, Номер 15(7), С. 2179 - 2179
Опубликована: Апрель 6, 2023
Skin cancer is one of the most lethal kinds human illness. In present state health care system, skin identification a time-consuming procedure and if it not diagnosed initially then can be threatening to life. To attain high prospect complete recovery, early detection crucial. last several years, application deep learning (DL) algorithms for has grown in popularity. Based on DL model, this work intended build multi-classification technique diagnosing cancers such as melanoma (MEL), basal cell carcinoma (BCC), squamous (SCC), melanocytic nevi (MN). paper, we have proposed novel learning-based classification network (DSCC_Net) that based convolutional neural (CNN), evaluated three publicly available benchmark datasets (i.e., ISIC 2020, HAM10000, DermIS). For diagnosis, performance DSCC_Net model compared with six baseline networks, including ResNet-152, Vgg-16, Vgg-19, Inception-V3, EfficientNet-B0, MobileNet. addition, used SMOTE Tomek handle minority classes issue exists dataset. The obtained 99.43% AUC, along 94.17%, accuracy, recall 93.76%, precision 94.28%, an F1-score 93.93% categorizing four distinct types diseases. rates accuracy MobileNet, Inception-V3 are 89.32%, 91.68%, 92.51%, 91.12%, 89.46% 91.82%, respectively. results showed our performs better models, thus offering significant support dermatologists experts diagnose cancer.
Язык: Английский
Процитировано
103Diagnostics, Год журнала: 2023, Номер 13(7), С. 1314 - 1314
Опубликована: Апрель 1, 2023
Melanoma is one of the deadliest types skin cancer that leads to death if not diagnosed early. Many lesions are similar in early stages, which causes an inaccurate diagnosis. Accurate diagnosis helps dermatologists save patients’ lives. In this paper, we propose hybrid systems based on advantages fused CNN models. models receive dermoscopy images ISIC 2019 dataset after segmenting area and isolating them from healthy through Geometric Active Contour (GAC) algorithm. Artificial neural network (ANN) Random Forest (Rf) features classify with high accuracy. The first methodology involved analyzing diagnosing their type using CNN-ANN CNN-RF. (AlexNet, GoogLeNet VGG16) only produce depth feature maps. Thus, deep maps were reduced by PCA then classified ANN RF networks. second CNN-RF It worth noting serially integrated reducing dimensions Principal Component Analysis (PCA). Hybrid achieved promising results for dermatoscopic data set distinguishing other lesions. AlexNet-GoogLeNet-VGG16-ANN model AUC 94.41%, sensitivity 88.90%, accuracy 96.10%, precision 88.69%, specificity 99.44%.
Язык: Английский
Процитировано
53Sensors, Год журнала: 2023, Номер 23(2), С. 743 - 743
Опубликована: Янв. 9, 2023
Coronavirus Disease 2019 (COVID-19) is still a threat to global health and safety, it anticipated that deep learning (DL) will be the most effective way of detecting COVID-19 other chest diseases such as lung cancer (LC), tuberculosis (TB), pneumothorax (PneuTh), pneumonia (Pneu). However, data sharing across hospitals hampered by patients' right privacy, leading unexpected results from neural network (DNN) models. Federated (FL) game-changing concept since allows clients train models together without their source with anybody else. Few studies, however, focus on improving model's accuracy stability, whereas existing FL-based detection techniques aim maximize secondary objectives latency, energy usage, privacy. In this work, we design novel model named decision-making-based federated (DMFL_Net) for medical diagnostic image analysis distinguish four distinct disorders including LC, TB, PneuTh, Pneu. The DMFL_Net has been suggested gathers variety hospitals, constructs using DenseNet-169, produces accurate predictions information kept secure only released authorized individuals. Extensive experiments were carried out X-rays (CXR), performance proposed was compared two transfer (TL) models, i.e., VGG-19 VGG-16 in terms (ACC), precision (PRE), recall (REC), specificity (SPF), F1-measure. Additionally, also default FL configurations. + DenseNet-169 achieves an 98.45% outperforms approaches classifying successfully protects privacy among diverse clients.
Язык: Английский
Процитировано
51Decision Analytics Journal, Год журнала: 2023, Номер 8, С. 100278 - 100278
Опубликована: Июнь 25, 2023
Artificial intelligence (AI) systems can assist in analyzing medical images and aiding the early detection of diseases. AI also ensure quality services by avoiding misdiagnosis caused human errors. This study proposes a deep neural network (DNN) model with fine-tuned training improved learning performance on dermoscopic for skin cancer detection. A knowledge base DL models is constructed combining different datasets. Transfer fine-tuning are implemented faster proposed limited dataset. The data augmentation techniques applied to enhance model. total 58,032 refined were used this study. output layered architecture aggregated perform binary classification cancer. trained investigated multiclass tasks. metrics confirm that DNN modified EfficientNetV2-M outperforms state-of-the-art learning-based models.
Язык: Английский
Процитировано
46Mathematics, Год журнала: 2024, Номер 12(7), С. 1030 - 1030
Опубликована: Март 29, 2024
The medical sciences are facing a major problem with the auto-detection of disease due to fast growth in population density. Intelligent systems assist professionals early detection and also help provide consistent treatment that reduces mortality rate. Skin cancer is considered be deadliest most severe kind cancer. Medical utilize dermoscopy images make manual diagnosis skin This method labor-intensive time-consuming demands considerable level expertise. Automated methods necessary for occurrence hair air bubbles dermoscopic affects research aims classify eight different types cancer, namely actinic keratosis (AKs), dermatofibroma (DFa), melanoma (MELa), basal cell carcinoma (BCCa), squamous (SCCa), melanocytic nevus (MNi), vascular lesion (VASn), benign (BKs). In this study, we propose SNC_Net, which integrates features derived from through deep learning (DL) models handcrafted (HC) feature extraction aim improving performance classifier. A convolutional neural network (CNN) employed classification. Dermoscopy publicly accessible ISIC 2019 dataset utilized train validate model. proposed model compared four baseline models, EfficientNetB0 (B1), MobileNetV2 (B2), DenseNet-121 (B3), ResNet-101 (B4), six state-of-the-art (SOTA) classifiers. With an accuracy 97.81%, precision 98.31%, recall 97.89%, F1 score 98.10%, outperformed SOTA classifiers as well models. Moreover, Ablation study performed on its performance. therefore assists dermatologists other detection.
Язык: Английский
Процитировано
32PLoS ONE, Год журнала: 2024, Номер 19(3), С. e0297667 - e0297667
Опубликована: Март 20, 2024
Skin cancer is a common affecting millions of people annually. cells inside the body that grow in unusual patterns are sign this invasive disease. The then spread to other organs and tissues through lymph nodes destroy them. Lifestyle changes increased solar exposure contribute rise incidence skin cancer. Early identification staging essential due high mortality rate associated with In study, we presented deep learning-based method named DVFNet for detection from dermoscopy images. To detect images pre-processed using anisotropic diffusion methods remove artifacts noise which enhances quality A combination VGG19 architecture Histogram Oriented Gradients (HOG) used research discriminative feature extraction. SMOTE Tomek resolve problem imbalanced multiple classes publicly available ISIC 2019 dataset. This study utilizes segmentation pinpoint areas significantly damaged cells. vector map created by combining features HOG VGG19. Multiclassification accomplished CNN maps. achieves an accuracy 98.32% on Analysis variance (ANOVA) statistical test validate model's accuracy. Healthcare experts utilize model at early clinical stage.
Язык: Английский
Процитировано
21International Journal of Biomedical Imaging, Год журнала: 2024, Номер 2024, С. 1 - 18
Опубликована: Фев. 3, 2024
Skin cancer is a significant health concern worldwide, and early accurate diagnosis plays crucial role in improving patient outcomes. In recent years, deep learning models have shown remarkable success various computer vision tasks, including image classification. this research study, we introduce an approach for skin classification using transformer, state-of-the-art architecture that has demonstrated exceptional performance diverse analysis tasks. The study utilizes the HAM10000 dataset; publicly available dataset comprising 10,015 lesion images classified into two categories: benign (6705 images) malignant (3310 images). This consists of high-resolution captured dermatoscopes carefully annotated by expert dermatologists. Preprocessing techniques, such as normalization augmentation, are applied to enhance robustness generalization model. transformer adapted task. model leverages self-attention mechanism capture intricate spatial dependencies long-range within images, enabling it effectively learn relevant features Segment Anything Model (SAM) employed segment cancerous areas from images; achieving IOU 96.01% Dice coefficient 98.14% then pretrained used architecture. Extensive experiments evaluations conducted assess our approach. results demonstrate superiority over traditional architectures general with some exceptions. Upon experimenting on six different models, ViT-Google, ViT-MAE, ViT-ResNet50, ViT-VAN, ViT-BEiT, ViT-DiT, found out ML achieves 96.15% accuracy Google’s ViT patch-32 low false negative ratio test dataset, showcasing its potential effective tool aiding dermatologists cancer.
Язык: Английский
Процитировано
19BMC Cancer, Год журнала: 2025, Номер 25(1)
Опубликована: Янв. 13, 2025
Melanoma is a highly aggressive skin cancer, where early and accurate diagnosis crucial to improve patient outcomes. Dermoscopy, non-invasive imaging technique, aids in melanoma detection but can be limited by subjective interpretation. Recently, machine learning deep techniques have shown promise enhancing diagnostic precision automating the analysis of dermoscopy images. This systematic review examines recent advancements (ML) (DL) applications for prognosis using We conducted thorough search across multiple databases, ultimately reviewing 34 studies published between 2016 2024. The covers range model architectures, including DenseNet ResNet, discusses datasets, methodologies, evaluation metrics used validate performance. Our results highlight that certain such as DCNN demonstrated outstanding performance, achieving over 95% accuracy on HAM10000, ISIC other datasets from provides insights into strengths, limitations, future research directions methods prognosis. It emphasizes challenges related data diversity, interpretability, computational resource requirements. underscores potential transform through improved efficiency. Future should focus creating accessible, large interpretability increase clinical applicability. By addressing these areas, models could play central role advancing care.
Язык: Английский
Процитировано
5Diagnostics, Год журнала: 2025, Номер 15(1), С. 99 - 99
Опубликована: Янв. 3, 2025
Background/Objectives: Early and accurate diagnosis of skin cancer improves survival rates; however, dermatologists often struggle with lesion detection due to similar pigmentation. Deep learning transfer models have shown promise in diagnosing cancers through image processing. Integrating attention mechanisms (AMs) deep has further enhanced the accuracy medical classification. While significant progress been made, research is needed improve accuracy. Previous studies not explored integration pre-trained Xception model for binary classification cancer. This study aims investigate impact various on model’s performance detecting benign malignant lesions. Methods: We conducted four experiments HAM10000 dataset. Three integrated self-attention (SL), hard (HD), soft (SF) mechanisms, while fourth used standard without mechanisms. Each mechanism analyzed features from uniquely: examined input relationships, hard-attention selected elements sparsely, soft-attention distributed focus probabilistically. Results: AMs into architecture effectively its performance. The alone was 91.05%. With AMs, increased 94.11% using self-attention, 93.29% attention, 92.97% attention. Moreover, proposed outperformed previous terms recall metrics, which are crucial investigations. Conclusions: These findings suggest that can enhance relation complex imaging tasks, potentially supporting earlier improving treatment outcomes.
Язык: Английский
Процитировано
2Cancers, Год журнала: 2023, Номер 15(14), С. 3604 - 3604
Опубликована: Июль 13, 2023
Skin cancer is a major public health concern around the world. identification critical for effective treatment and improved results. Deep learning models have shown considerable promise in assisting dermatologists skin diagnosis. This study proposes SBXception: shallower broader variant of Xception network. It uses as base model classification increases its performance by reducing depth expanding breadth architecture. We used HAM10000 dataset, which contains 10,015 dermatoscopic images lesions classified into seven categories, training testing proposed model. Using we fine-tuned new reached an accuracy 96.97% on holdout test set. SBXception also achieved significant enhancement with 54.27% fewer parameters reduced time compared to Our findings show that architecture can greatly improve categorization.
Язык: Английский
Процитировано
34