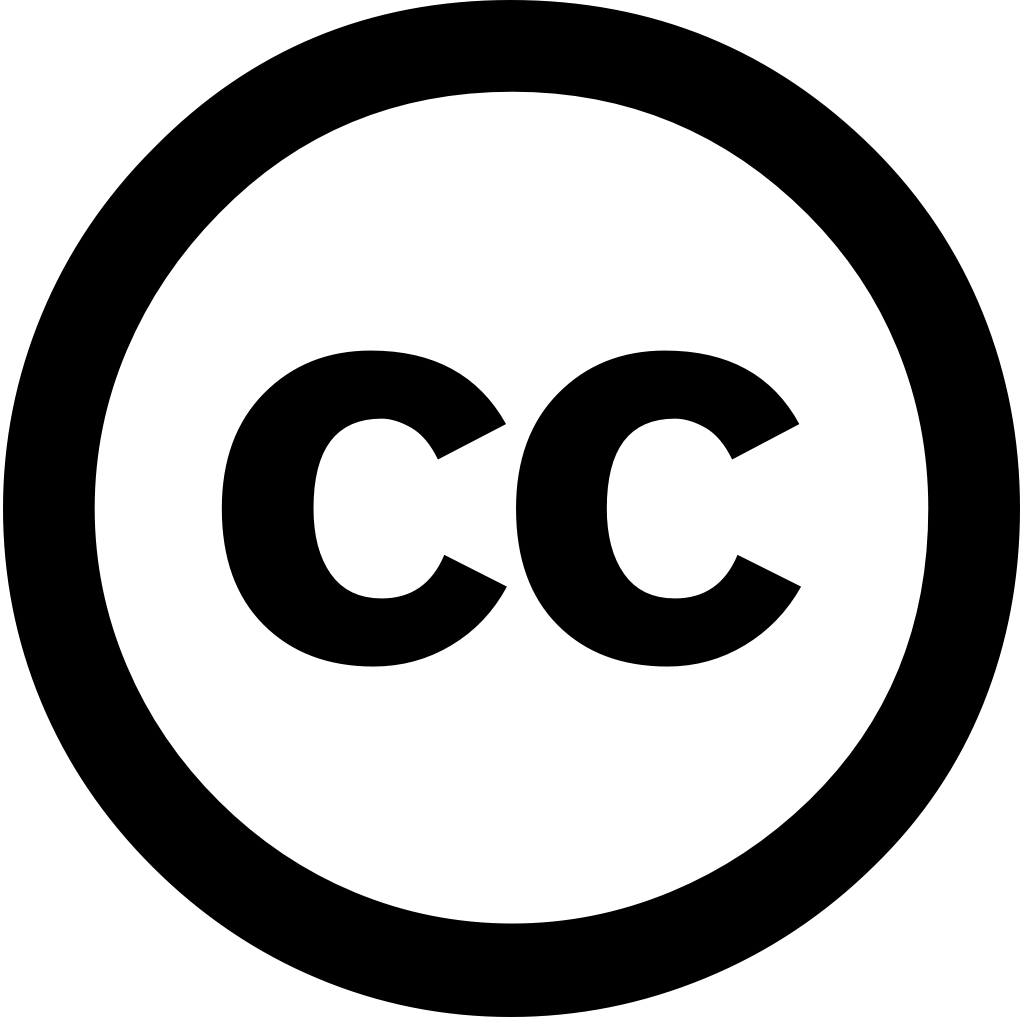
Scientific Reports, Год журнала: 2025, Номер 15(1)
Опубликована: Янв. 21, 2025
Язык: Английский
Scientific Reports, Год журнала: 2025, Номер 15(1)
Опубликована: Янв. 21, 2025
Язык: Английский
Advanced Engineering Informatics, Год журнала: 2023, Номер 57, С. 102036 - 102036
Опубликована: Май 30, 2023
Язык: Английский
Процитировано
30Cancers, Год журнала: 2023, Номер 15(19), С. 4694 - 4694
Опубликована: Сен. 23, 2023
Background: Melanoma, the deadliest form of skin cancer, poses a significant public health challenge worldwide. Early detection is crucial for improved patient outcomes. Non-invasive imaging techniques allow diagnostic accuracy; however, their use often limited due to need skilled practitioners trained interpret images in standardized fashion. Recent innovations artificial intelligence (AI)-based lesion image interpretation show potential AI early melanoma. Objective: The aim this study was evaluate current state AI-based used combination with non-invasive modalities including reflectance confocal microscopy (RCM), optical coherence tomography (OCT), and dermoscopy. We also aimed determine whether application can lead accuracy Methods: A systematic search conducted via Medline/PubMed, Cochrane, Embase databases eligible publications between 2018 2022. Screening methods adhered 2020 version PRISMA (Preferred Reporting Items Systematic Reviews Meta-Analyses) guidelines. Included studies utilized algorithms melanoma directly addressed review objectives. Results: retrieved 40 papers amongst three databases. All comparing performance dermatologists reported superior or equivalent improving In algorithm on dermoscopy dermatologists, achieved higher ROC (>80%) these comparative using dermoscopic images, mean sensitivity 83.01% specificity 85.58%. Studies evaluating machine learning conjunction OCT boasted 95%, while RCM rate 82.72%. Conclusions: Our results demonstrate robust improve outcomes through identification Further are needed assess generalizability across different populations types, standardization processing, further compare board-certified clinical applicability.
Язык: Английский
Процитировано
25Bioengineering, Год журнала: 2023, Номер 10(2), С. 203 - 203
Опубликована: Фев. 3, 2023
Due to the rapid rate of SARS-CoV-2 dissemination, a conversant and effective strategy must be employed isolate COVID-19. When it comes determining identity COVID-19, one most significant obstacles that researchers overcome is propagation virus, in addition dearth trustworthy testing models. This problem continues difficult for clinicians deal with. The use AI image processing has made formerly insurmountable challenge finding COVID-19 situations more manageable. In real world, there handled about difficulties sharing data between hospitals while still honoring privacy concerns organizations. training global deep learning (DL) model, crucial handle fundamental such as user collaborative model development. For this study, novel framework designed compiles information from five different databases (several hospitals) edifies using blockchain-based federated (FL). validated through blockchain technology (BCT), FL trains on scale maintaining secrecy proposed divided into three parts. First, we provide method normalization can diversity collected sources several computed tomography (CT) scanners. Second, categorize patients, ensemble capsule network (CapsNet) with incremental extreme machines (IELMs). Thirdly, interactively BCT anonymity. Extensive tests employing chest CT scans comparison classification performance DL algorithms predicting protecting variety users, were undertaken. Our findings indicate improved effectiveness identifying patients achieved an accuracy 98.99%. Thus, our provides substantial aid medical practitioners their diagnosis
Язык: Английский
Процитировано
24Engineering Technology & Applied Science Research, Год журнала: 2024, Номер 14(1), С. 12734 - 12739
Опубликована: Фев. 8, 2024
The application of Computer Vision (CV) and image processing in the medical sector is great significance, especially recognition skin cancer using dermoscopic images. Dermoscopy denotes a non-invasive imaging system that offers clear visuals cancers, allowing dermatologists to analyze identify various features crucial for lesion assessment. Over past few years, there has been an increasing fascination with Deep Learning (DL) applications recognition, particular focus on impressive results achieved by Neural Networks (DNNs). DL approaches, predominantly CNNs, have exhibited immense potential automating classification detection cancers. This study presents Automated Skin Cancer Detection Classification method Cat Swarm Optimization (ASCDC-CSODL). main objective ASCDC-CSODL enforce model recognize classify tumors In ASCDC-CSODL, Bilateral Filtering (BF) applied noise elimination U-Net employed segmentation process. Moreover, exploits MobileNet feature extraction Gated Recurrent Unit (GRU) approach used cancer. Finally, CSO algorithm alters hyperparameter values GRU. A wide-ranging simulation was performed evaluate performance model, demonstrating significantly improved over other approaches.
Язык: Английский
Процитировано
14Cluster Computing, Год журнала: 2024, Номер unknown
Опубликована: Июнь 17, 2024
Abstract
Skin
cancer
is
one
of
the
most
dangerous
types
due
to
its
immediate
appearance
and
possibility
rapid
spread.
It
arises
from
uncontrollably
growing
cells,
rapidly
dividing
cells
in
area
body,
invading
other
bodily
tissues,
spreading
throughout
body.
Early
detection
helps
prevent
progress
reaching
critical
levels,
reducing
risk
complications
need
for
more
aggressive
treatment
options.
Convolutional
neural
networks
(CNNs)
revolutionize
skin
diagnosis
by
extracting
intricate
features
images,
enabling
an
accurate
classification
lesions.
Their
role
extends
early
detection,
providing
a
powerful
tool
dermatologists
identify
abnormalities
their
nascent
stages,
ultimately
improving
patient
outcomes.
This
study
proposes
novel
deep
convolutional
network
(DCNN)
approach
classifying
The
proposed
DCNN
model
evaluated
using
two
unbalanced
datasets,
namely
HAM10000
ISIC-2019.
compared
with
transfer
learning
models,
including
VGG16,
VGG19,
DenseNet121,
DenseNet201,
MobileNetV2.
Its
performance
assessed
four
widely
used
evaluation
metrics:
accuracy,
recall,
precision,
F1-score,
specificity,
AUC.
experimental
results
demonstrate
that
outperforms
(DL)
models
utilized
these
datasets.
achieved
highest
accuracy
ISIC-2019
$$98.5\%$$
Язык: Английский
Процитировано
10Diagnostics, Год журнала: 2024, Номер 14(4), С. 454 - 454
Опубликована: Фев. 19, 2024
In recent years, there has been growing interest in the use of computer-assisted technology for early detection skin cancer through analysis dermatoscopic images. However, accuracy illustrated behind state-of-the-art approaches depends on several factors, such as quality images and interpretation results by medical experts. This systematic review aims to critically assess efficacy challenges this research field order explain usability limitations highlight potential future lines work scientific clinical community. study, was carried out over 45 contemporary studies extracted from databases Web Science Scopus. Several computer vision techniques related image video processing diagnosis were identified. context, focus process included algorithms employed, result accuracy, validation metrics. Thus, yielded significant advancements using deep learning machine algorithms. Lastly, establishes a foundation research, highlighting contributions opportunities improve effectiveness learning.
Язык: Английский
Процитировано
8BMC Medical Informatics and Decision Making, Год журнала: 2025, Номер 25(1)
Опубликована: Янв. 31, 2025
Abstract This paper introduces SkinWiseNet (SWNet), a deep convolutional neural network designed for the detection and automatic classification of potentially malignant skin cancer conditions. SWNet optimizes feature extraction through multiple pathways, emphasizing width augmentation to enhance efficiency. The proposed model addresses potential biases associated with conditions, particularly in individuals darker tones or excessive hair, by incorporating fusion assimilate insights from diverse datasets. Extensive experiments were conducted using publicly accessible datasets evaluate SWNet’s effectiveness.This study utilized four datasets-Mnist-HAM10000, ISIC2019, ISIC2020, Melanoma Skin Cancer-comprising images categorized into benign classes. Explainable Artificial Intelligence (XAI) techniques, specifically Grad-CAM, employed interpretability model’s decisions. Comparative analysis was performed three pre-existing learning networks-EfficientNet, MobileNet, Darknet. results demonstrate superiority, achieving an accuracy 99.86% F1 score 99.95%, underscoring its efficacy gradient propagation capture across various levels. research highlights significant advancing classification, providing robust tool accurate early diagnosis. integration enhances mitigates hair tones. outcomes this contribute improved patient healthcare practices, showcasing exceptional capabilities classification.
Язык: Английский
Процитировано
1Expert Systems with Applications, Год журнала: 2022, Номер 215, С. 119352 - 119352
Опубликована: Ноя. 28, 2022
Язык: Английский
Процитировано
31Sensors, Год журнала: 2023, Номер 23(20), С. 8457 - 8457
Опубликована: Окт. 13, 2023
Skin cancer is considered a dangerous type of with high global mortality rate. Manual skin diagnosis challenging and time-consuming method due to the complexity disease. Recently, deep learning transfer have been most effective methods for diagnosing this deadly cancer. To aid dermatologists other healthcare professionals in classifying images into melanoma nonmelanoma enabling treatment patients at an early stage, systematic literature review (SLR) presents various federated (FL) (TL) techniques that widely applied. This study explores FL TL classifiers by evaluating them terms performance metrics reported research studies, which include true positive rate (TPR), negative (TNR), area under curve (AUC), accuracy (ACC). was assembled systemized reviewing well-reputed studies published eminent fora between January 2018 July 2023. The existing compiled through search seven databases. A total 86 articles were included SLR. SLR contains recent on algorithms malignant In addition, taxonomy presented summarizes many non-malignant classes. results highlight limitations challenges research. Consequently, future direction work opportunities interested researchers are established help automated classification cancers.
Язык: Английский
Процитировано
16Diagnostics, Год журнала: 2023, Номер 13(15), С. 2531 - 2531
Опубликована: Июль 29, 2023
A dermatologist-like automatic classification system is developed in this paper to recognize nine different classes of pigmented skin lesions (PSLs), using a separable vision transformer (SVT) technique assist clinical experts early cancer detection. In the past, researchers have few systems PSLs. However, they often require enormous computations achieve high performance, which burdensome deploy on resource-constrained devices. paper, new approach designing SVT architecture based SqueezeNet and depthwise CNN models. The primary goal find deep learning with parameters that has comparable accuracy state-of-the-art (SOTA) architectures. This modifies design for improved runtime performance by utilizing convolutions rather than simple conventional units. To develop Assist-Dermo system, data augmentation applied control PSL imbalance problem. Next, pre-processing step integrated select most dominant region then enhance lesion patterns perceptual-oriented color space. Afterwards, designed improve efficacy several layers multiple filter sizes but fewer filters parameters. For training evaluation models, set images collected from online sources such as Ph2, ISBI-2017, HAM10000, ISIC On chosen dataset, it achieves an (ACC) 95.6%, sensitivity (SE) 96.7%, specificity (SP) 95%, area under curve (AUC) 0.95. experimental results show suggested outperformed SOTA algorithms when recognizing performed better other competitive can support dermatologists diagnosis wide variety PSLs through dermoscopy. model code freely available GitHub scientific community.
Язык: Английский
Процитировано
16