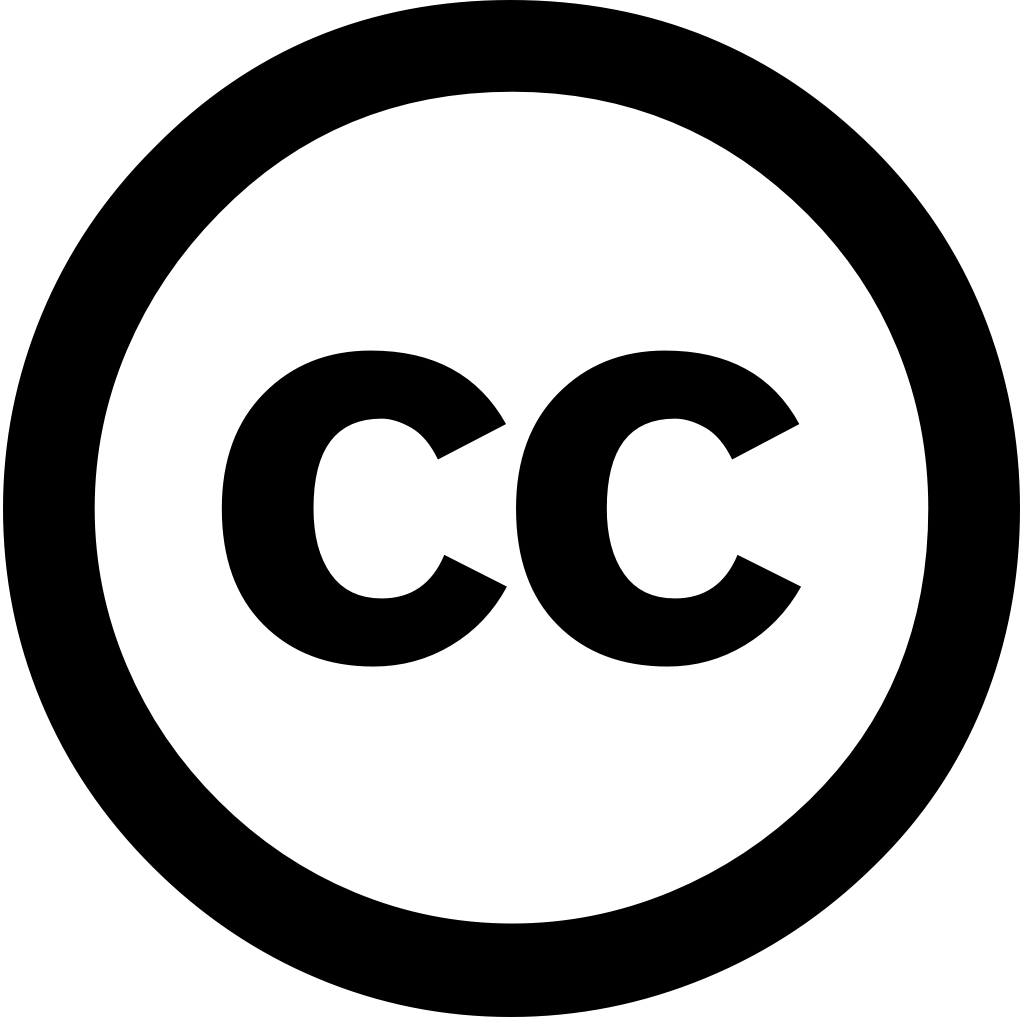
Scientific Reports, Год журнала: 2025, Номер 15(1)
Опубликована: Янв. 21, 2025
Язык: Английский
Scientific Reports, Год журнала: 2025, Номер 15(1)
Опубликована: Янв. 21, 2025
Язык: Английский
Cancers, Год журнала: 2024, Номер 16(3), С. 629 - 629
Опубликована: Фев. 1, 2024
The objective of this study is to systematically analyze the current state literature regarding novel artificial intelligence (AI) machine learning models utilized in non-invasive imaging for early detection nonmelanoma skin cancers. Furthermore, we aimed assess their potential clinical relevance by evaluating accuracy, sensitivity, and specificity each algorithm assessing risk bias. Two reviewers screened MEDLINE, Cochrane, PubMed, Embase databases peer-reviewed studies that focused on AI-based cancer classification involving cancers were published between 2018 2023. search terms included neoplasms, nonmelanoma, basal-cell carcinoma, squamous-cell diagnostic techniques procedures, intelligence, algorithms, computer systems, dermoscopy, reflectance confocal microscopy, optical coherence tomography. Based results, only directly answered review objectives efficacy measures recorded. A QUADAS-2 assessment bias was then conducted. total 44 our review; 40 utilizing 3 using microscopy (RCM), 1 hyperspectral epidermal (HEI). average accuracy AI algorithms applied all modalities combined 86.80%, with same dermoscopy. Only one three applying RCM measured a result 87%. Accuracy not regard based HEI interpretation. exhibited an overall favorable performance diagnosis via noninvasive techniques. Ultimately, further research needed isolate pooled as many testing datasets also include melanoma other pigmented lesions.
Язык: Английский
Процитировано
5Scientific Reports, Год журнала: 2024, Номер 14(1)
Опубликована: Июль 27, 2024
Abstract Skin cancer is a type of disease in which abnormal alterations skin characteristics can be detected. It treated if it detected early. Many artificial intelligence-based models have been developed for detection and classification. Considering the development numerous according to various scenarios selecting optimum model was rarely considered previous works. This study aimed develop classification select model. Convolutional neural networks (CNNs) form AlexNet, Inception V3, MobileNet V2, ResNet 50 were used feature extraction. Feature reduction carried out using two algorithms grey wolf optimizer (GWO) addition original features. images classified into four classes based on six machine learning (ML) classifiers. As result, 51 with different combinations CNN algorithms, without GWO ML To best results, multicriteria decision-making approach utilized rank alternatives by perimeter similarity (RAPS). Model training testing conducted International Imaging Collaboration (ISIC) 2017 dataset. Based nine evaluation metrics RAPS method, AlexNet algorithm classical yielded model, achieving accuracy 94.5%. work presents first benchmarking many models. not only reduces time spent but also improves accuracy. The method has proven its robustness problem
Язык: Английский
Процитировано
5Expert Systems with Applications, Год журнала: 2024, Номер 252, С. 124056 - 124056
Опубликована: Апрель 30, 2024
Язык: Английский
Процитировано
4Scientific Reports, Год журнала: 2025, Номер 15(1)
Опубликована: Янв. 7, 2025
Accurate acne severity grading is crucial for effective clinical treatment and timely follow-up management. Although some artificial intelligence methods have been developed to automate the process of grading, diversity image capture sources various application scenarios can affect their performance. Therefore, it's necessary design special evaluate them systematically before introducing into practice. To develop a deep learning-based algorithm that could accurately accomplish lesion detection simultaneously in different healthcare scenarios. We collected 2,157 facial images from two public three self-built datasets model development evaluation. An called AcneDGNet was constructed with feature extraction module, module module. Its performance evaluated both online offline Experimental results on largest most diverse evaluation revealed overall achieved accuracies 89.5% 89.8% For visits scenarios, accuracy detecting changing trends reached 87.8%, total counting error 1.91 ± 3.28 all lesions. Additionally, prospective demonstrated not only much more accurate than junior dermatologists but also comparable senior dermatologists. These findings indicated effectively assist patients diagnosis management acne,
Язык: Английский
Процитировано
0Scientific Reports, Год журнала: 2025, Номер 15(1)
Опубликована: Янв. 21, 2025
Язык: Английский
Процитировано
0