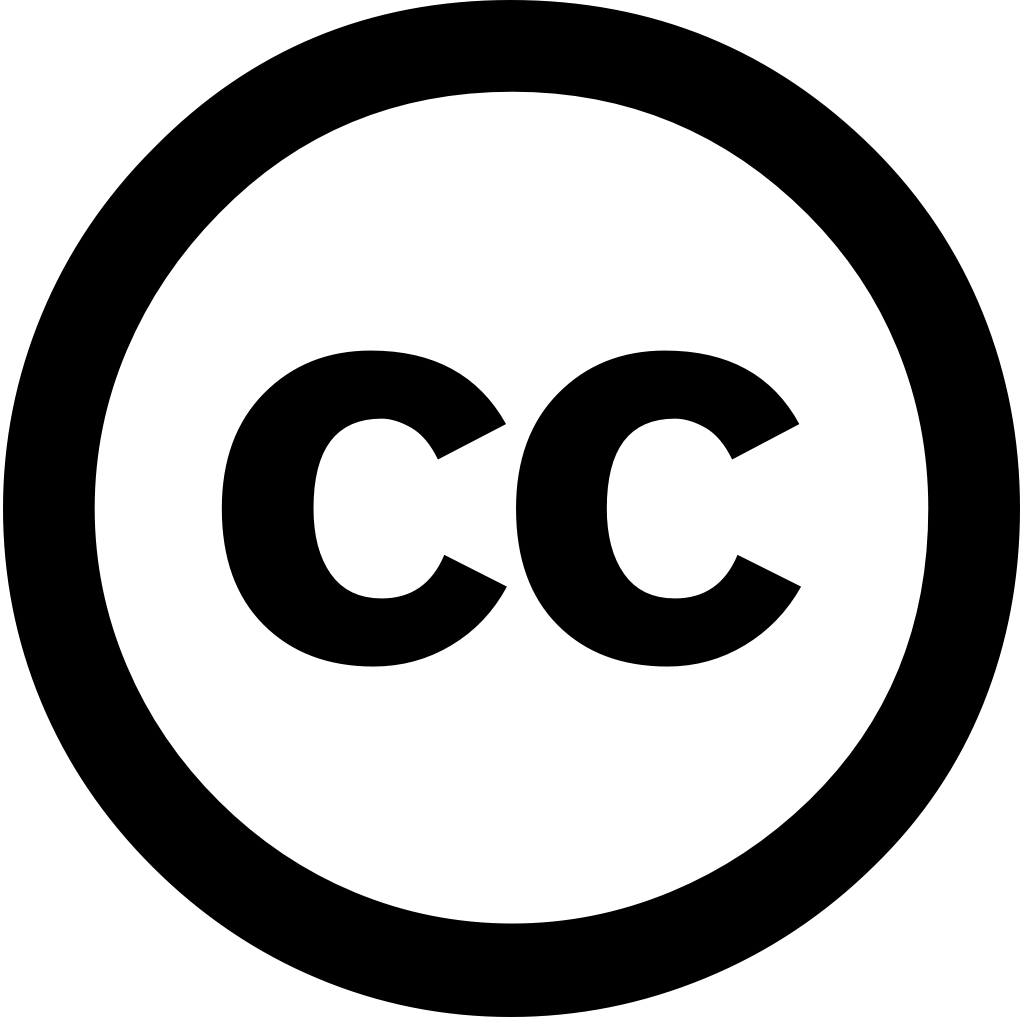
Sensors, Год журнала: 2024, Номер 24(23), С. 7857 - 7857
Опубликована: Дек. 9, 2024
Recent applications of wearable inertial measurement units (IMUs) for predicting human movement have often entailed estimating action-level (e.g., walking, running, jumping) and joint-level ankle plantarflexion angle) motion. Although or information is frequently the focus intent prediction, contextual necessary a more thorough approach to recognition. Therefore, combination may offer comprehensive intent. In this study, we devised novel hierarchical-based method combining classification subsequent regression predict joint angles 100 ms into future. K-nearest neighbors (KNN), bidirectional long short-term memory (BiLSTM), temporal convolutional network (TCN) models were employed classification, random forest model trained on action-specific IMU data was used prediction. A action-generic multiple actions backward kneeling down, up, walking) also angle. Compared with approach, had lower prediction error up. TCN BiLSTM classifiers achieved accuracies 89.87% 89.30%, respectively, they did not surpass performance when in an model. This been because from actions. study demonstrates advantage leveraging large, disparate sources over Moreover, it efficacy IMU-driven, task-agnostic future across
Язык: Английский