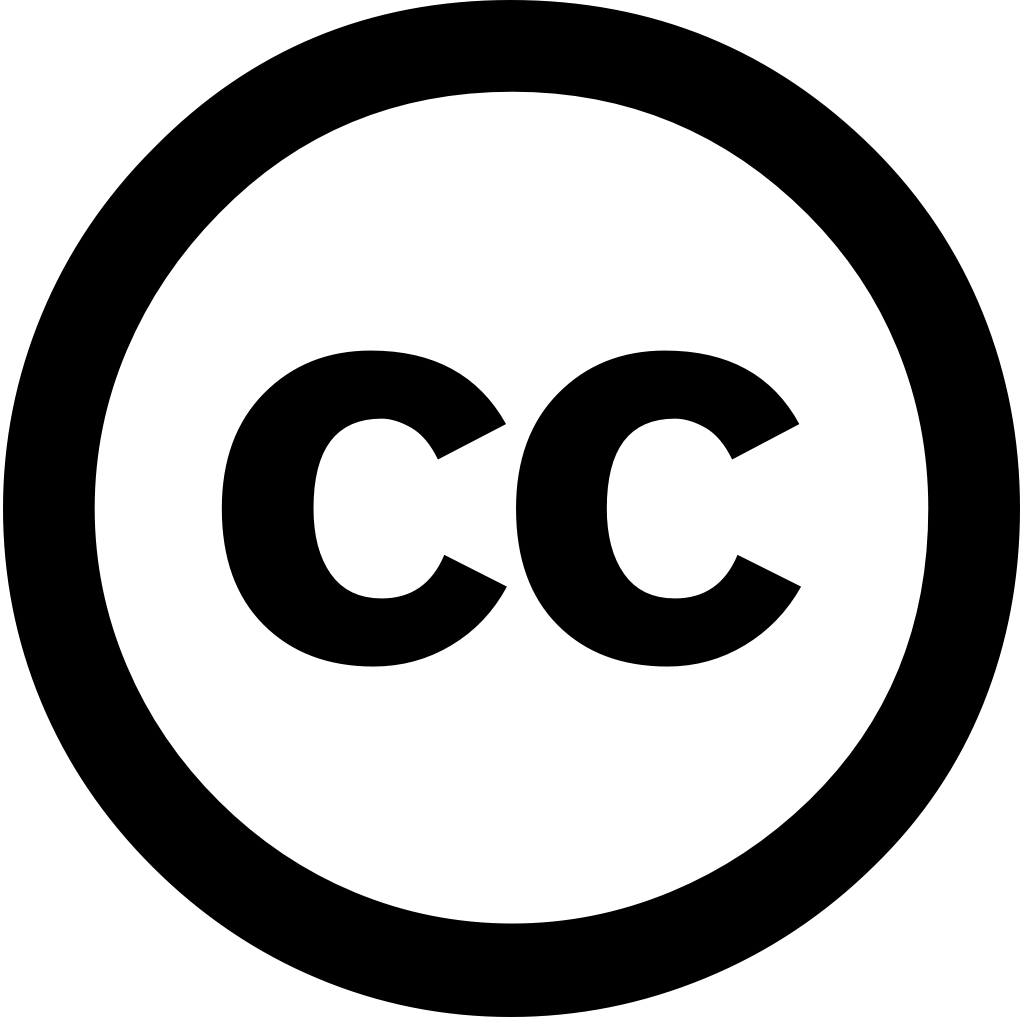
Applied System Innovation, Год журнала: 2025, Номер 8(2), С. 53 - 53
Опубликована: Апрель 15, 2025
This paper presents a novel Human Activity Recognition (HAR) framework using wearable sensors, specifically targeting applications in gait rehabilitation and assistive robots. The new methodology includes the usage of an open-source dataset. dataset surface electromyography (sEMG) inertial measurement units (IMUs) signals for lower limb 22 healthy subjects. Several activities daily living (ADLs) were included, such as walking, stairs up/down ramp walking. A signal conditioning, denoising, filtering, feature extraction activity classification is proposed. After testing several conditioning approaches, Wavelet transform (WT), Principal Component Analysis (PCA) Empirical Mode Decomposition (EMD), autocepstrum analysis (ACA)-based approach chosen. Such complex effective enables supervised classifiers like K-nearest neighbor (KNN), neural networks (NN) random forest (RF). classifier has shown best results with accuracy 97.63% EMG extracted from soleus muscle. Additionally, RF IMU 98.52%. These emphasize potential HAR systems rehabilitation, paving way real-time implementation devices.
Язык: Английский