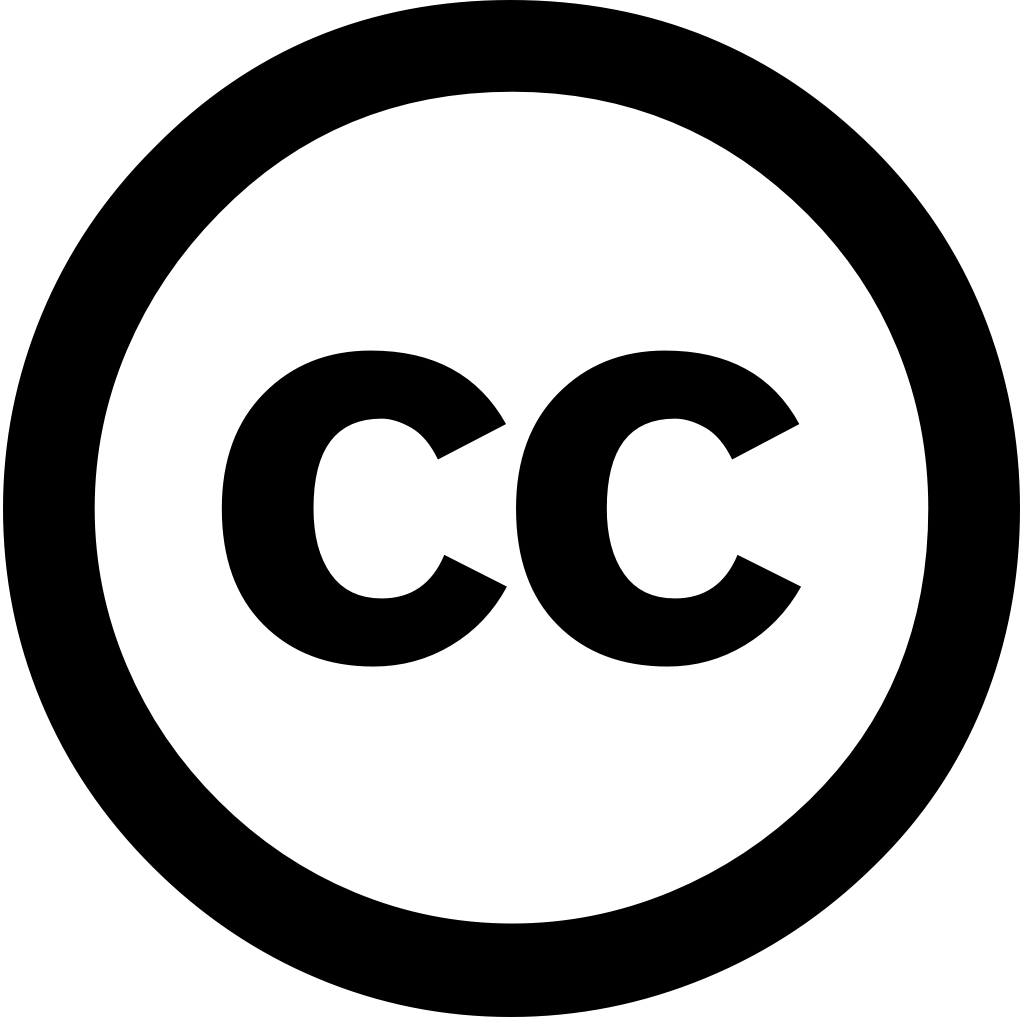
Mathematics, Год журнала: 2025, Номер 13(2), С. 266 - 266
Опубликована: Янв. 15, 2025
Table detection in document images is a challenging problem due to diverse layouts, irregular structures, and embedded graphical elements. In this study, we present HTTD (Hierarchical Transformer for Detection), cutting-edge model that combines Swin-L backbone with advanced Transformer-based mechanisms achieve superior performance. addresses three key challenges: handling including historical modern structures; improving computational efficiency training convergence; demonstrating adaptability non-standard tasks like medical imaging receipt detection. Evaluated on benchmark datasets, achieves state-of-the-art results, precision rates of 96.98% ICDAR-2019 cTDaR, 96.43% TNCR, 93.14% TabRecSet. These results validate its effectiveness efficiency, paving the way analysis data digitization tasks.
Язык: Английский