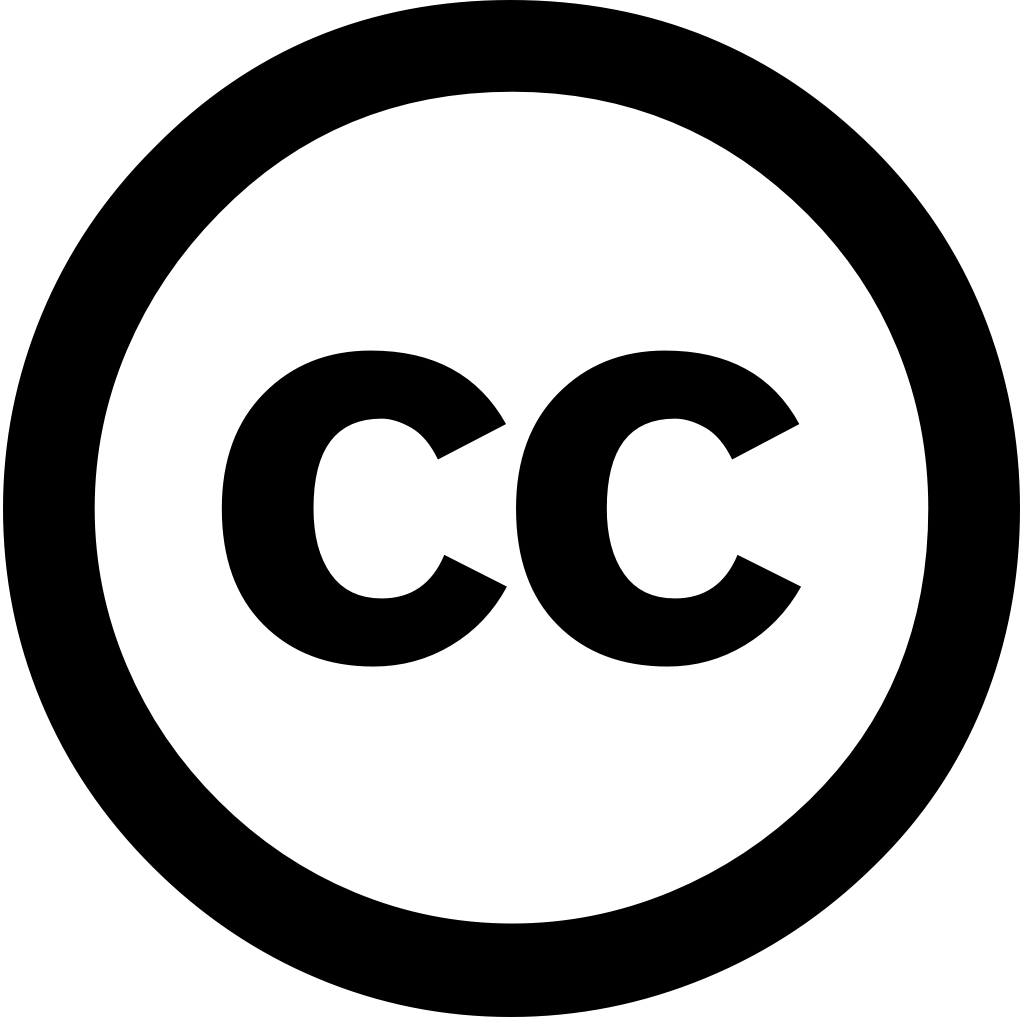
Research Square (Research Square), Год журнала: 2024, Номер unknown
Опубликована: Ноя. 15, 2024
Язык: Английский
Research Square (Research Square), Год журнала: 2024, Номер unknown
Опубликована: Ноя. 15, 2024
Язык: Английский
Water, Год журнала: 2025, Номер 17(3), С. 362 - 362
Опубликована: Янв. 27, 2025
Groundwater serves as an indispensable global resource, essential for agriculture, industry, and the urban water supply. Predicting groundwater level in karst regions presents notable challenges due to intricate geological structures fluctuating climatic conditions. This study examines Qingzhen City, China, introducing innovative hybrid model, Hodrick–Prescott (HP) filter–Long Short-Term Memory (LSTM) network (HP-LSTM), which integrates HP filter with LSTM enhance precision of forecasting. By attenuating short-term noise, HP-LSTM model improves long-term trend prediction accuracy. Findings reveal that significantly outperformed conventional LSTM, attaining R2 values 0.99, 0.96, 0.98 on training, validation, test datasets, respectively, contrast 0.92, 0.76, 0.95. The achieved RMSE 0.0276 a MAPE 2.92% set, outperforming (RMSE: 0.1149; MAPE: 9.14%) capturing patterns reducing fluctuations. While is effective at modeling dynamics, it more prone resulting greater errors. Overall, demonstrates superior robustness prediction, whereas may be better suited scenarios requiring rapid adaptation variations. Selecting appropriate tailored specific predictive needs can thus optimize management strategies.
Язык: Английский
Процитировано
1Acta Geophysica, Год журнала: 2025, Номер unknown
Опубликована: Март 24, 2025
Язык: Английский
Процитировано
0Expert Systems with Applications, Год журнала: 2024, Номер unknown, С. 125379 - 125379
Опубликована: Сен. 1, 2024
Язык: Английский
Процитировано
2Remote Sensing, Год журнала: 2024, Номер 16(22), С. 4196 - 4196
Опубликована: Ноя. 11, 2024
This review examines the integration of remote sensing technologies and machine learning models for efficient monitoring management lake water quality. It critically evaluates performance various satellite platforms, including Landsat, Sentinel-2, MODIS, RapidEye, Hyperion, in assessing key quality parameters chlorophyll-a (Chl-a), turbidity, colored dissolved organic matter (CDOM). highlights specific advantages each platform, considering factors like spatial temporal resolution, spectral coverage, suitability these platforms different sizes characteristics. In addition to this paper explores application a wide range models, from traditional linear tree-based methods more advanced deep techniques convolutional neural networks (CNNs), recurrent (RNNs), generative adversarial (GANs). These are analyzed their ability handle complexities inherent data, high dimensionality, non-linear relationships, multispectral hyperspectral data. also discusses effectiveness predicting parameters, offering insights into most appropriate model–satellite combinations scenarios. Moreover, identifies challenges associated with data quality, model interpretability, integrating imagery models. emphasizes need advancements fusion techniques, improved generalizability, developing robust frameworks multi-source concludes by targeted recommendations future research, highlighting potential interdisciplinary collaborations enhance sustainable management.
Язык: Английский
Процитировано
1Research Square (Research Square), Год журнала: 2024, Номер unknown
Опубликована: Ноя. 15, 2024
Язык: Английский
Процитировано
0