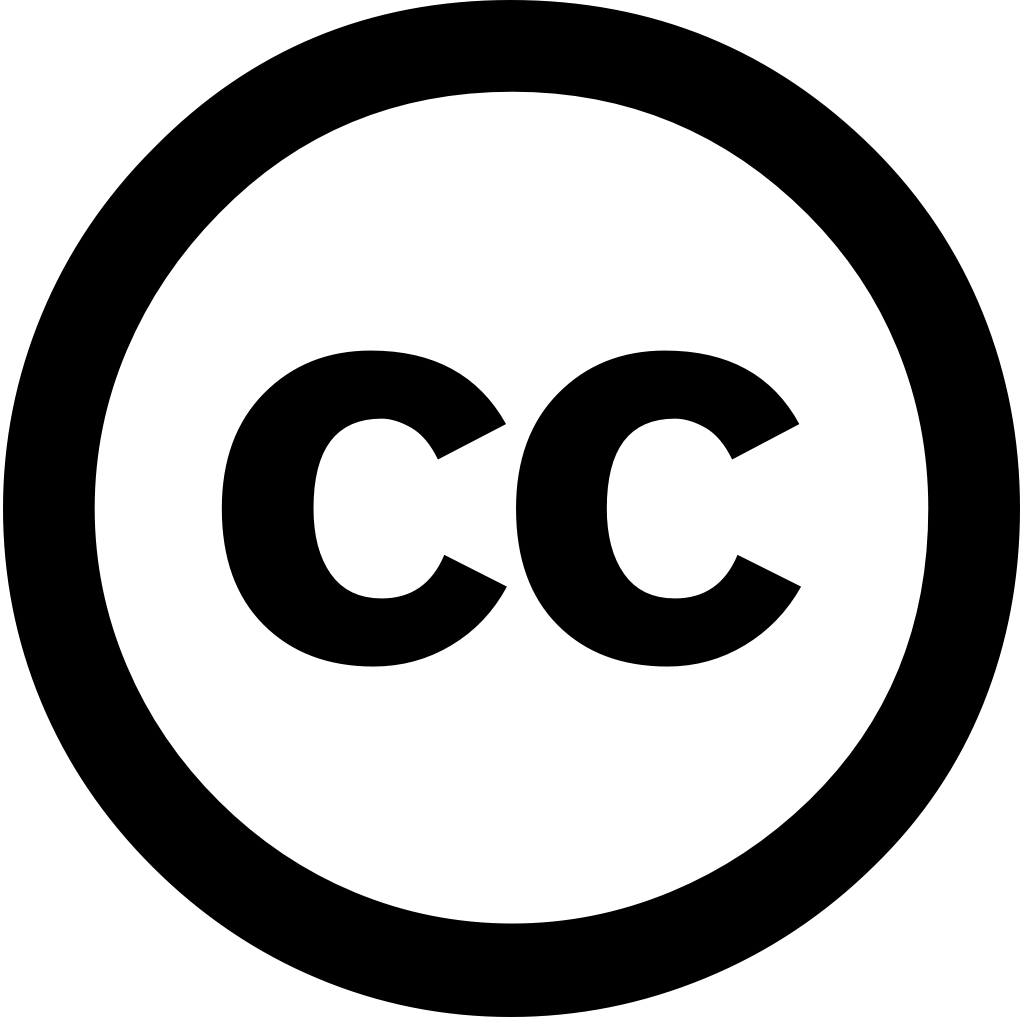
Land, Год журнала: 2025, Номер 14(5), С. 925 - 925
Опубликована: Апрель 24, 2025
The Jinsha River Basin in Yunnan serves as a crucial ecological barrier southwestern China. Objective assessment and identification of key driving factors are essential for the region’s sustainable development. Remote Sensing Ecological Index (RSEI) has been widely applied assessments. In recent years, interpretable machine learning (IML) introduced novel approaches understanding complex mechanisms. This study employed Google Earth Engine (GEE) to calculate three vegetation indices—NDVI, SAVI, kNDVI—for area from 2000 2022, along with their corresponding RSEI models (NDVI-RSEI, SAVI-RSEI, kNDVI-RSEI). Additionally, it analyzed spatiotemporal variations these relationship indices. Furthermore, an IML model (XGBoost-SHAP) was interpret RSEI. results indicate that (1) levels 2022 were primarily moderate; (2) compared NDVI-RSEI, SAVI-RSEI is more susceptible soil factors, while kNDVI-RSEI exhibits lower saturation tendency; (3) potential evapotranspiration, land cover, elevation drivers variations, affecting environment western, southeastern, northeastern parts area. XGBoost-SHAP approach provides valuable insights promoting regional
Язык: Английский