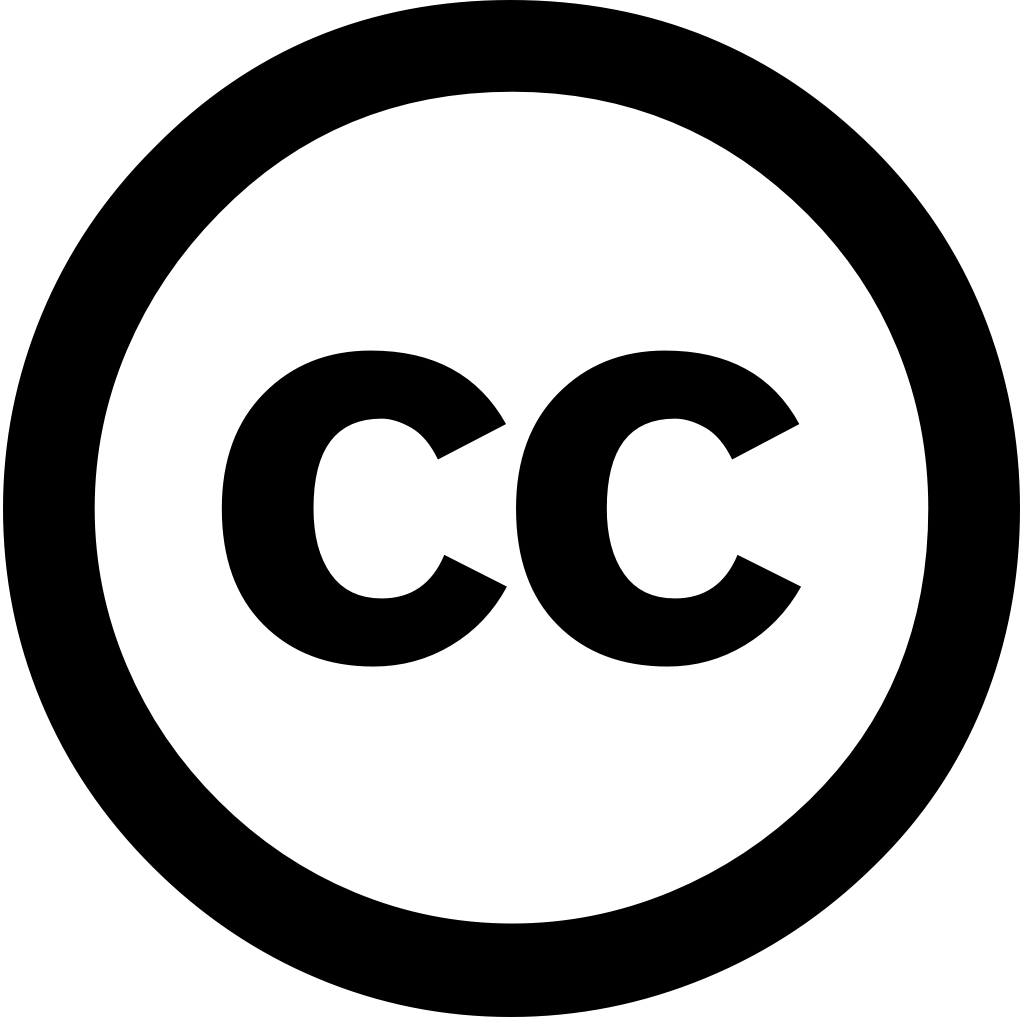
PeerJ Computer Science, Год журнала: 2024, Номер 10, С. e2409 - e2409
Опубликована: Окт. 18, 2024
Digital image processing is a constantly evolving field encompassing wide range of techniques and applications. Researchers worldwide are continually developing various algorithms across multiple fields to achieve accurate classification. Advanced computer vision crucial for architectural artistic analysis. The digitalization art has significantly enhanced the accessibility conservation fine-art paintings, yet risk theft remains significant challenge. Improving security necessitates precise identification paintings. Although current recognition systems have shown potential, there scope enhancing their efficiency. We developed an improved system categorizing paintings using convolutional transformers, specified by attention mechanism enhance focused learning on data. As part most advanced architectures in deep family, transformers empowered multi-head mechanism, thus improving To assess performance our model, we compared it with those four pre-trained networks: ResNet50, VGG16, AlexNet, ViT. Each network was integrated into corresponding state-of-the-art model as first blocks. These models were constructed under transfer strategy, one commonly used approaches this field. experimental results showed that proposed outperformed other models. Our study also highlighted effectiveness features.
Язык: Английский