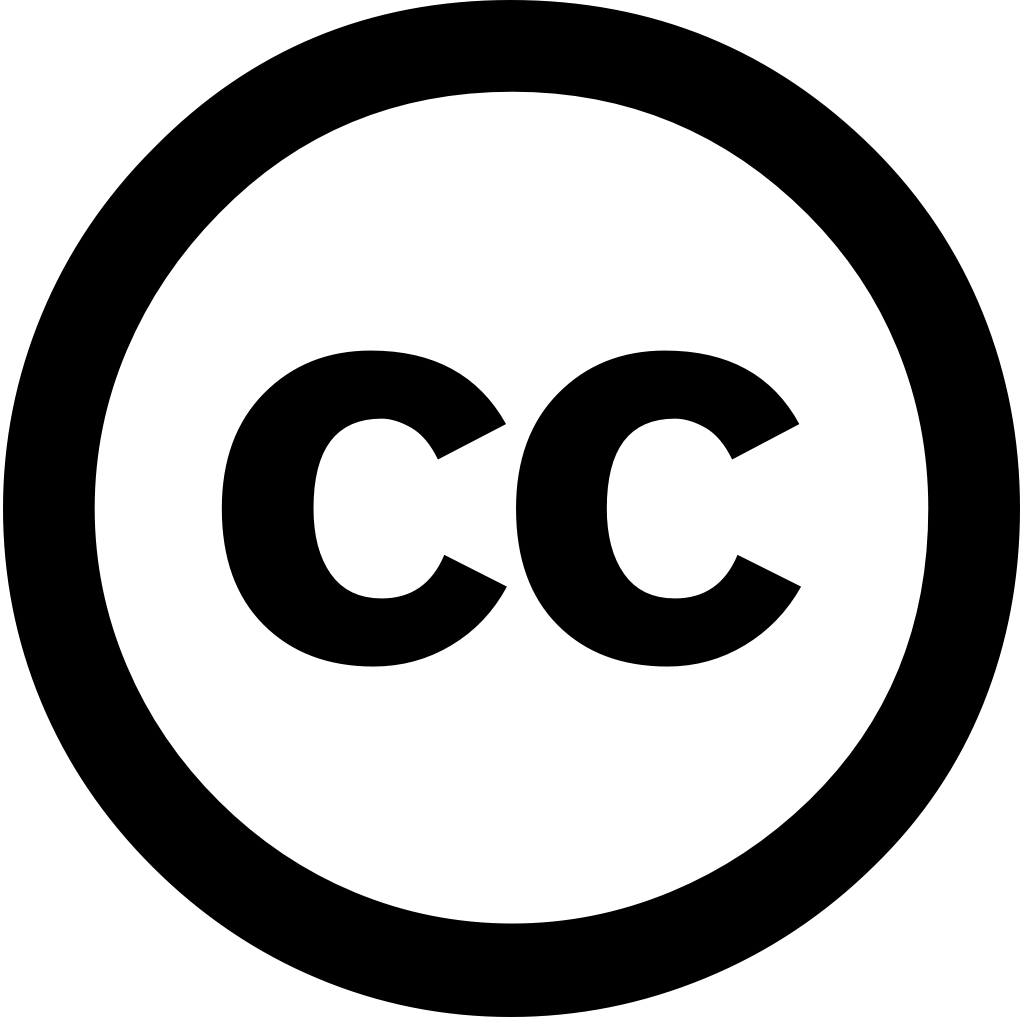
Опубликована: Ноя. 20, 2024
Recently, the primary reason for blindness in adults has been diabetic retinopathy (DR) disease. Therefore, there is an increasing demand a real-time efficient classification and detection system to overcome fast-growing disease (DR). We introduced novel deep hybrid model auto-mated recognition classification. Our leverages power of CNN architectures: Inception V3 VGG16 models by combining their strengths cater exact requirements. efficiently captures fine features wide-ranging such as textures edges, crucial classifying initial signs DR. Similarly, V3’s architecture proficient at detecting multiscale patterns, providing extensive setting shaping occurrence more complex DR severity stages. allows extraction various appearance retinal images, which can better assist proposed evaluated on diverse datasets, including EyePACS1 APTOS2019, demonstrating confident performance 99.63% accuracy levels dataset, while 98.70% APTOS2019 indicating that our well distinguished different stages highly detection. This helps clinicians medical experts classify identify early. automatic manage treat patient effectively introduces timely treatment.
Язык: Английский