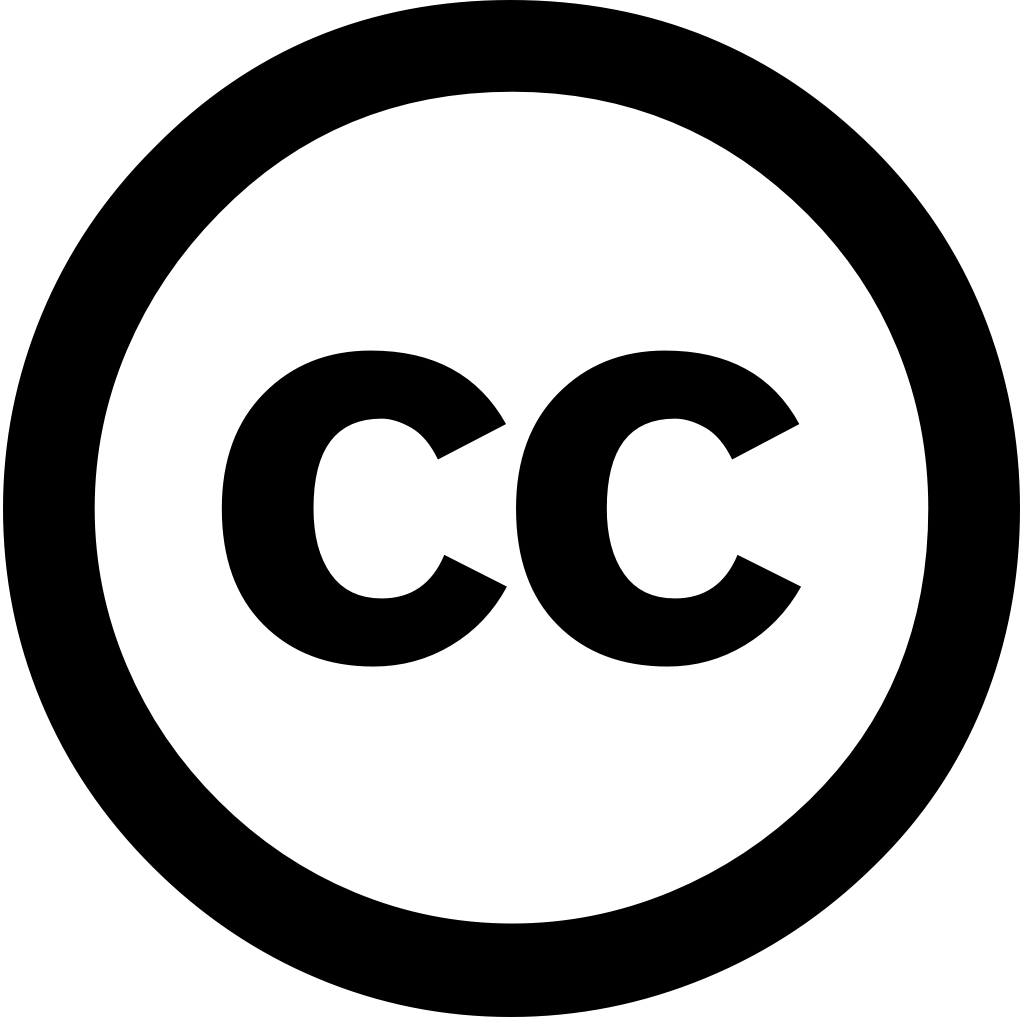
ICST Transactions on Scalable Information Systems, Год журнала: 2024, Номер 11
Опубликована: Июль 31, 2024
INTRODUCTION: Insider threats are a major issue for cyber security. In contrast to external attackers, insiders have more privileges and authorized access data resources, which can cause an organization great harm. To completely understand insider's activities throughout the organization, sophisticated method is needed. OBJECTIVES: Based on organization's login activity, this study proposes novel conceptual insider threat detection. Behavioural such as HTTP, Email Login details collected create dataset further processed pre-processing using transformation Trimmed Score Regression (TSR). METHODS: These pre-data given feature extraction process Deep Feature Synthesis (DFS) extraction. The extracted fed Physics Informed Neural Networks (PINN) RESULTS: prediction of PINN was improved through optimally choosing parameters learning rate weight Hunter-prey Optimization (HPO). proposed model offers 68% detection rate, 98.4% accuracy, 5% FDR, 95% F1_score 0.7005 sec execution time. CONCLUSION: Observed outcomes compared other traditional approaches validation. with shows that provides better than in models therefore good fit real-time prediction.
Язык: Английский