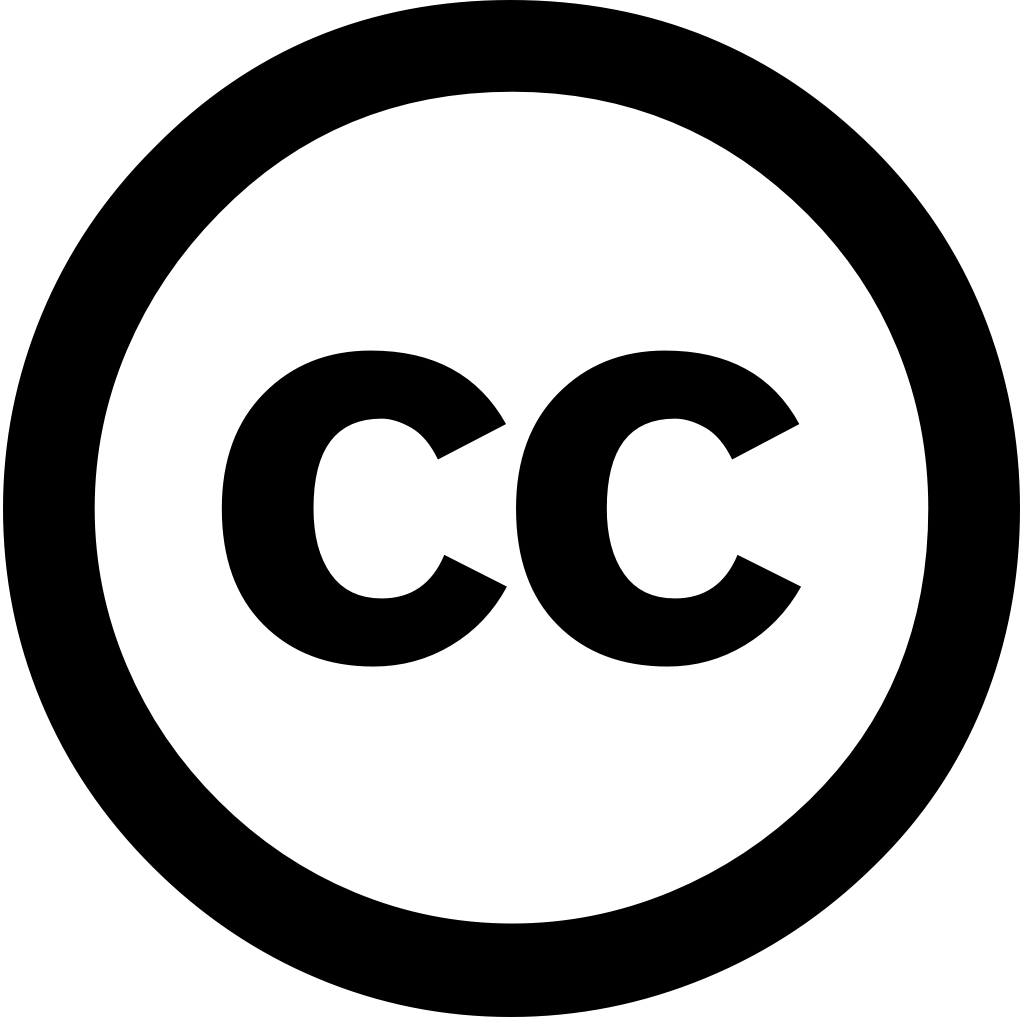
PLoS ONE, Год журнала: 2025, Номер 20(1), С. e0305561 - e0305561
Опубликована: Янв. 16, 2025
This paper presents a novel method for improving semantic segmentation performance in computer vision tasks. Our approach utilizes an enhanced UNet architecture that leverages improved ResNet50 backbone. We replace the last layer of with deformable convolution to enhance feature representation. Additionally, we incorporate attention mechanism, specifically ECA-ASPP (Attention Spatial Pyramid Pooling), encoding path capture multi-scale contextual information effectively. In decoding UNet, explore use mechanisms after concatenating low-level features high-level features. Specifically, investigate two types mechanisms: ECA (Efficient Channel Attention) and LKA (Large Kernel Attention). experiments demonstrate incorporating concatenation improves accuracy. Furthermore, compare modules decoder path. The results indicate module outperforms module. finding highlights importance exploring different their impact on performance. To evaluate effectiveness proposed method, conduct benchmark datasets, including Stanford Cityscapes, as well newly introduced WildPASS DensPASS datasets. Based our experiments, achieved state-of-the-art mIoU 85.79 82.25 dataset, Cityscapes respectively. performs these achieving high
Язык: Английский