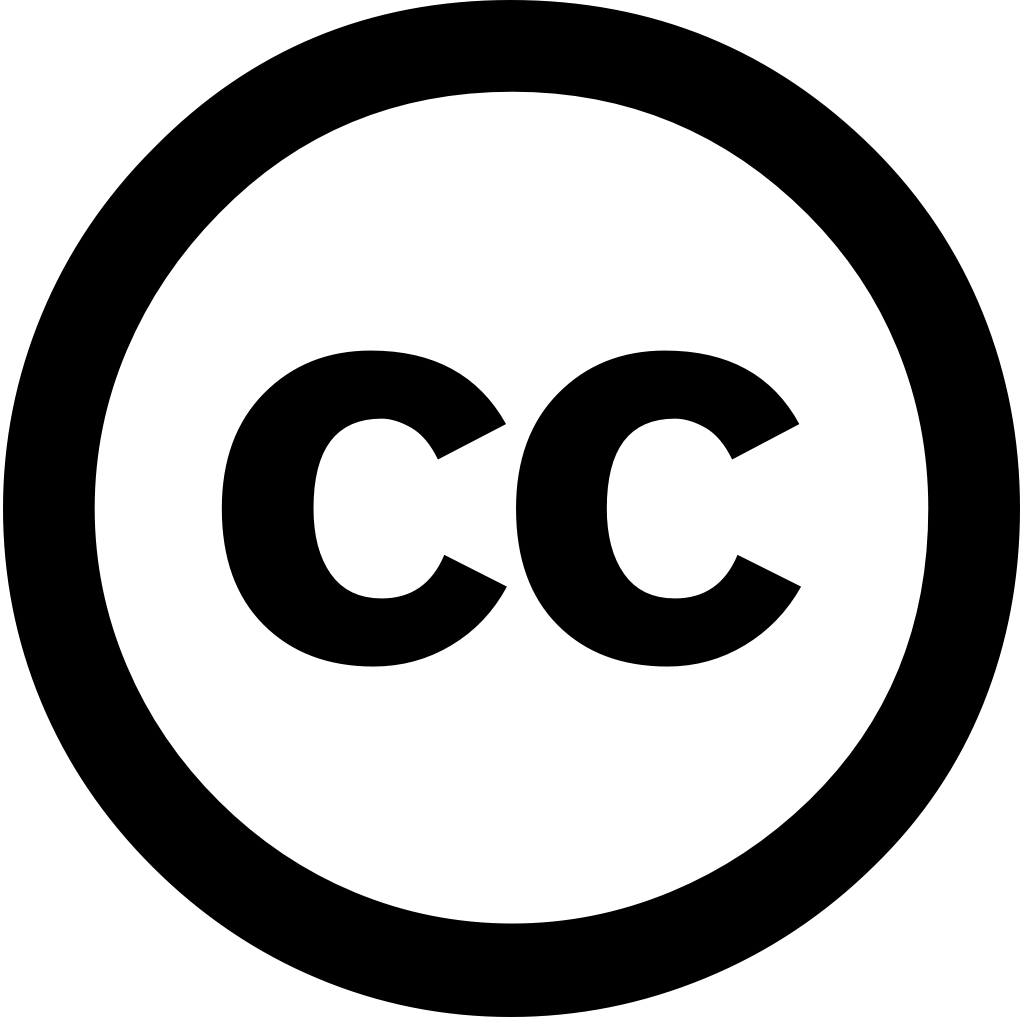
Computers in Biology and Medicine, Год журнала: 2025, Номер 191, С. 110121 - 110121
Опубликована: Апрель 14, 2025
Cardiac arrhythmias are irregular heart rhythms that, if undetected, can lead to severe cardiovascular conditions. Detecting these anomalies early through electrocardiogram (ECG) signal analysis is critical for preventive healthcare and effective treatment. However, the automatic classification of poses significant challenges, including class imbalance noise interference in ECG signals. This paper introduces Multi-Scale Convolutional LSTM Dense Network (MS-CLDNet) model, an advanced deep-learning model specifically designed address issues improve arrhythmia accuracy other relevant metrics. aims develop efficient MS-CLDNet, accurately classifying cardiac from Addressing challenges like interference, integrates bidirectional long short-term memory (LSTM) networks temporal pattern recognition, Blocks feature refinement, Neural Networks (CNNs) robust extraction. To achieve accurate different types arrhythmias, Classification Head refines extracted features even further. Utilizing MIT-BIH dataset, key pre-processing techniques such as wavelet-based denoising were employed enhance clarity. Results indicate that MS-CLDNet achieves a 98.22 %, outperforming baseline models with low average loss values (0.084). research highlights how crucial it combine sophisticated neural network architectures precision automated diagnostic systems, which could have important applications detection.
Язык: Английский