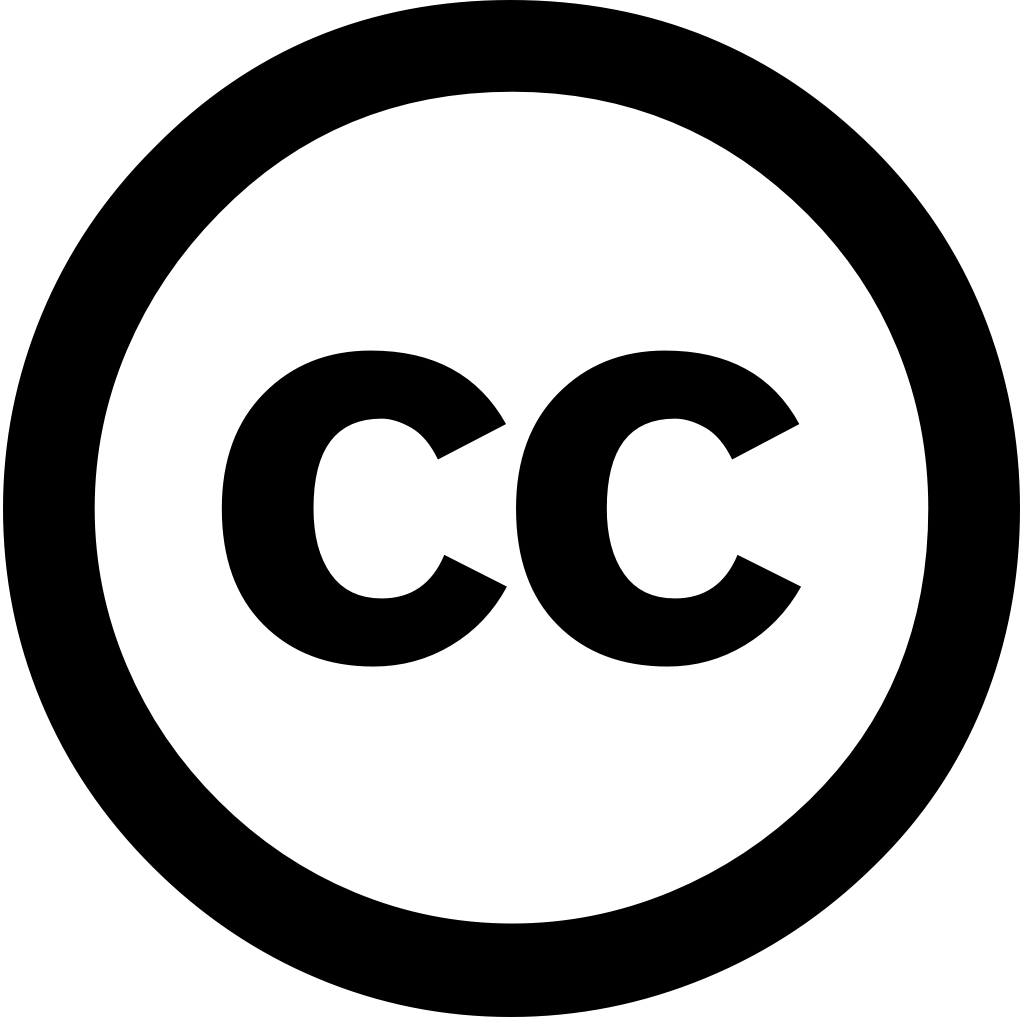
Cogent Food & Agriculture, Год журнала: 2025, Номер 11(1)
Опубликована: Фев. 12, 2025
Image classification poses a significant challenge in agriculture. However, the utilization of popular algorithms such as vision transformers and convolutional neural networks has often fallen short numerous agricultural tasks owing to scarcity extensively labelled data reliance on pretrained models trained generic datasets. To address this, our study details pretraining ViTs using 224,228 images, employing masked image modeling for preprocessing. The model was then fine-tuned three independent datasets performed better than state-of-the-art methods. For example, method achieved highest accuracy rates 76.18%, 98.49%, 88.56% IP102, DeepWeeds, Tsinghua Dogs datasets, respectively. This enhancement can be attributed robust strategy we have developed through extensive experimentation with MIM model. Our encompasses advanced models, leveraging histogram oriented gradient features reconstruction target, selecting an appropriate mask ratio. We hope that this research will prompt application self-supervised learning techniques, represented by model, wide range image-related future.
Язык: Английский