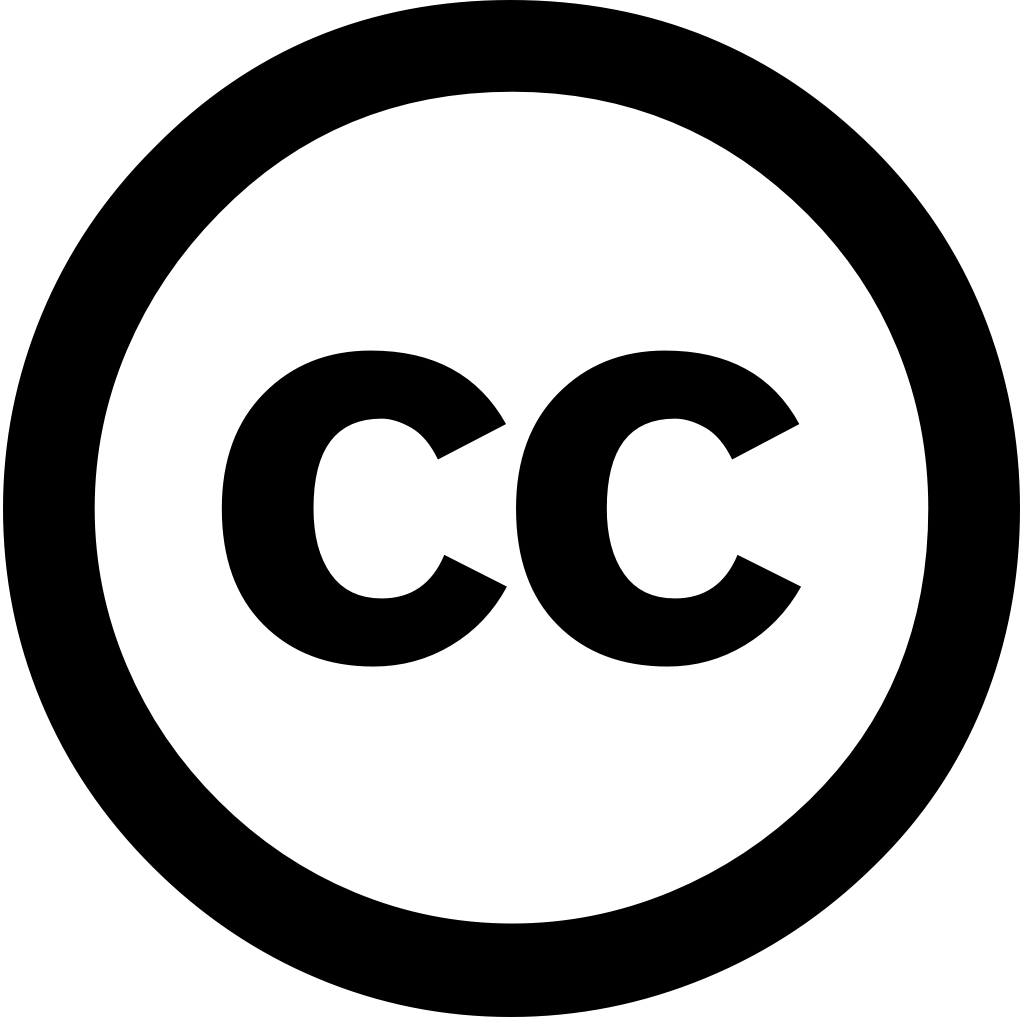
Scientific Reports, Год журнала: 2025, Номер 15(1)
Опубликована: Фев. 12, 2025
Язык: Английский
Scientific Reports, Год журнала: 2025, Номер 15(1)
Опубликована: Фев. 12, 2025
Язык: Английский
Seminars in Nuclear Medicine, Год журнала: 2025, Номер unknown
Опубликована: Март 1, 2025
Malignant lymphomas encompass a range of malignancies with incidence rising globally, particularly age. In younger populations, Hodgkin and Burkitt predominate, while older populations more commonly experience subtypes such as diffuse large B-cell, follicular, marginal zone, mantle cell lymphomas. Positron emission tomography/computed tomography (PET/CT) using [18F] fluorodeoxyglucose (FDG) is the gold standard for staging, treatment response assessment, prognostication in lymphoma. However, interpretation PET/CT complex, time-consuming, reliant on expert imaging specialists, exacerbating challenges associated workforce shortages worldwide. Artificial intelligence (AI) offers transformative potential across multiple aspects this setting. AI applications appointment planning have demonstrated utility reducing nonattendance rates improving departmental efficiency. Advanced reconstruction techniques leveraging convolutional neural networks (CNNs) enable reduced injected activities radiopharmaceutical patient dose whilst maintaining diagnostic accuracy, benefiting patients requiring scans. Automated segmentation tools, predominantly 3D U-Net architectures, improved quantification metrics total metabolic tumour volume (TMTV) lesion glycolysis (TLG), facilitating stratification. Despite these advancements, remain, including variability performance, impact Deauville Score interpretation, standardization TMTV/TLG measurements. Emerging language models (LLMs) also show promise enhancing reporting, converting free-text reports into structured formats, communication. Further research required to address limitations AI-induced errors, physiological uptake differentiation, integration clinical workflows. With robust validation harmonization, could significantly enhance lymphoma care, precision, workflow efficiency, outcomes.
Язык: Английский
Процитировано
1Journal of Clinical Medicine, Год журнала: 2025, Номер 14(2), С. 572 - 572
Опубликована: Янв. 17, 2025
Background: Several artificial intelligence systems based on large language models (LLMs) have been commercially developed, with recent interest in integrating them for clinical questions. Recent versions now include image analysis capacity, but their performance gastroenterology remains untested. This study assesses ChatGPT-4's interpreting images. Methods: A total of 740 images from five procedures-capsule endoscopy (CE), device-assisted enteroscopy (DAE), endoscopic ultrasound (EUS), digital single-operator cholangioscopy (DSOC), and high-resolution anoscopy (HRA)-were included analyzed by ChatGPT-4 using a predefined prompt each. predictions were compared to gold standard diagnoses. Statistical analyses accuracy, sensitivity, specificity, positive predictive value (PPV), negative (NPV), area under the curve (AUC). Results: For CE, demonstrated accuracies ranging 50.0% 90.0%, AUCs 0.50-0.90. DAE, model an accuracy 67.0% (AUC 0.670). EUS, system showed 0.488 0.550 differentiation between pancreatic cystic solid lesions, respectively. The LLM differentiated benign malignant biliary strictures AUC 0.550. HRA, overall 47.5% 67.5%. Conclusions: suboptimal diagnostic interpretation across several techniques, highlighting need continuous improvement before adoption.
Язык: Английский
Процитировано
0Scientific Reports, Год журнала: 2025, Номер 15(1)
Опубликована: Фев. 12, 2025
Язык: Английский
Процитировано
0