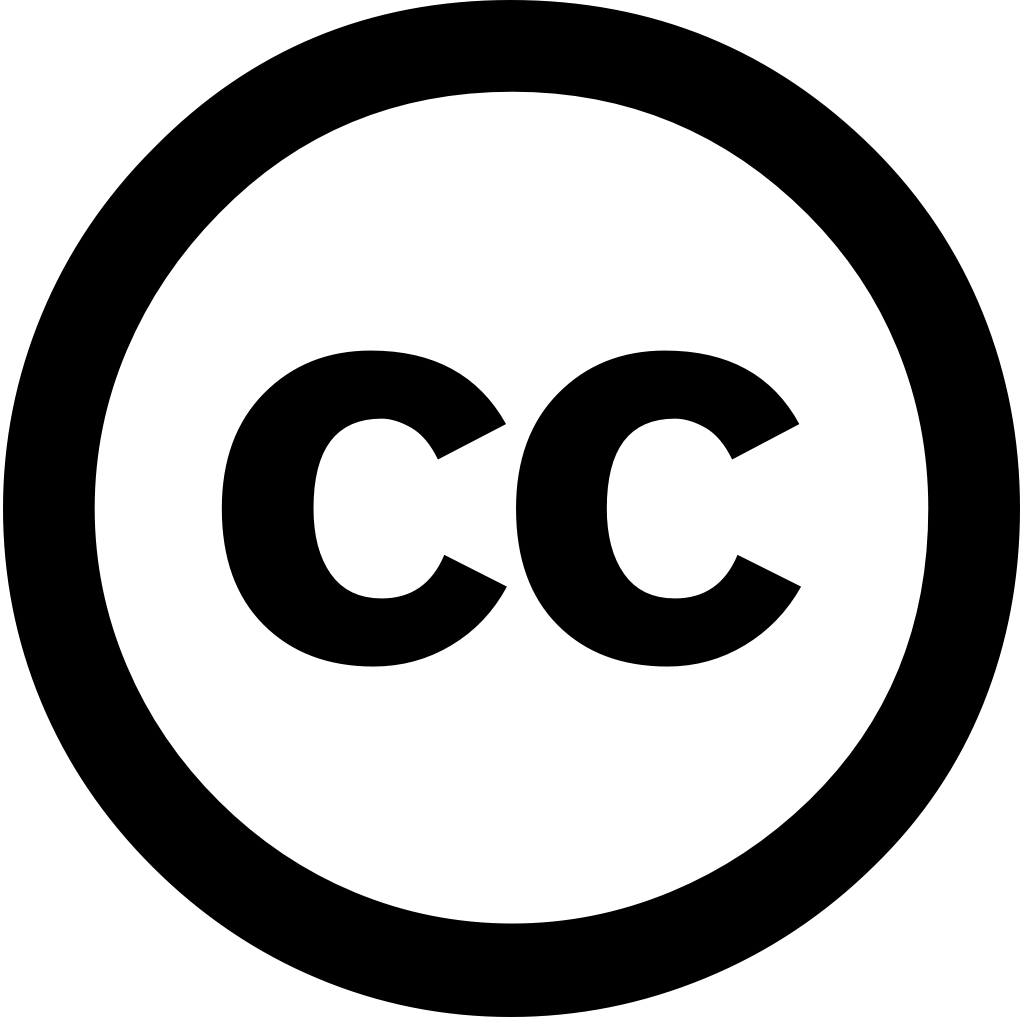
BMJ evidence-based medicine, Год журнала: 2024, Номер unknown, С. bmjebm - 113199
Опубликована: Дек. 20, 2024
Язык: Английский
BMJ evidence-based medicine, Год журнала: 2024, Номер unknown, С. bmjebm - 113199
Опубликована: Дек. 20, 2024
Язык: Английский
Mechanical Systems and Signal Processing, Год журнала: 2025, Номер 232, С. 112683 - 112683
Опубликована: Апрель 14, 2025
Язык: Английский
Процитировано
0Journal of Biomedical Informatics, Год журнала: 2025, Номер 166, С. 104819 - 104819
Опубликована: Апрель 16, 2025
Язык: Английский
Процитировано
0Surgical Endoscopy, Год журнала: 2025, Номер unknown
Опубликована: Апрель 18, 2025
Язык: Английский
Процитировано
0Research Synthesis Methods, Год журнала: 2025, Номер unknown, С. 1 - 19
Опубликована: Апрель 24, 2025
Abstract Introduction With the increasing accessibility of tools such as ChatGPT, Copilot, DeepSeek, Dall-E, and Gemini, generative artificial intelligence (GenAI) has been poised a potential, research timesaving tool, especially for synthesising evidence. Our objective was to determine whether GenAI can assist with evidence synthesis by assessing its performance using accuracy, error rates, time savings compared traditional expert-driven approach. Methods To systematically review evidence, we searched five databases on 17 January 2025, synthesised outcomes reporting or taken, appraised risk-of-bias modified version QUADAS-2. Results We identified 3,071 unique records, 19 which were included in our review. Most studies had high unclear Domain 1A: selection, 2A: conduct, 1B: applicability results. When used (1) searching missed 68% 96% (median = 91%) studies, (2) screening made incorrect inclusion decisions ranging from 0% 29% 10%); exclusion 1% 83% 28%), (3) data extractions 4% 31% 14%), (4) assessments 10% 56% 27%). Conclusion shows that current does not support use without human involvement oversight. However, most tasks other than searching, may have role assisting humans synthesis.
Язык: Английский
Процитировано
0medRxiv (Cold Spring Harbor Laboratory), Год журнала: 2024, Номер unknown
Опубликована: Июль 16, 2024
ABSTRACT Systematic reviews are essential for evidence based healthcare, but conducting them is time and resource consuming. To date, efforts have been made to accelerate (semi-) automate various steps of systematic through the use artificial intelligence emergence large language models (LLMs) promises further opportunities. One crucial complex task within review conduct assessing risk bias included studies. Therefore, aim this study was test LLM Claude 2 assessment 100 randomized controlled trials using revised Cochrane tool (“RoB 2”; involving judgements five specific domains an overall judgement). We assessed agreement by with human published in Reviews. The observed between authors ranged from 41% judgement 71% domain 4 (“outcome measurement”). Cohen’s κ lowest 5 (“selective reporting”; 0.10 (95% confidence interval (CI): −0.10-0.31)) highest 3 (“missing data”; 0.31 CI: 0.10-0.52)), indicating slight fair agreement. Fair found (Cohen’s κ: 0.22 0.06-0.38)). Sensitivity analyses alternative prompting techniques or more recent version did not result substantial changes. Currently, Claude’s RoB cannot replace assessment. However, potential LLMs support should be explored.
Язык: Английский
Процитировано
2Опубликована: Янв. 1, 2024
Язык: Английский
Процитировано
1Medical Teacher, Год журнала: 2024, Номер unknown, С. 1 - 3
Опубликована: Авг. 4, 2024
What is the educational challenge? The Medical Education Research Study Quality Instrument (MERSQI) widely used to evaluate quality of quantitative research in medical education. It has strong evidence validity and endorsed by guidelines. However, manual appraisal process time-consuming resource-intensive, highlighting need for more efficient methods. are proposed solutions? We propose use ChatGPT education with MERSQI compare its scoring those human evaluators. potential benefits a broader global audience? Using can decrease resources required appraisal. This allows faster summaries evidence, reducing workload researchers, editors, educators. Furthermore, ChatGPTs' capability extract supporting excerpts provides transparency may have data extraction training new researchers. next steps? plan continue evaluating using other instruments determine feasibility this realm. Moreover, we investigate which types studies performs best in.
Язык: Английский
Процитировано
0Опубликована: Сен. 2, 2024
Язык: Английский
Процитировано
0BMJ evidence-based medicine, Год журнала: 2024, Номер unknown, С. bmjebm - 113199
Опубликована: Дек. 20, 2024
Язык: Английский
Процитировано
0