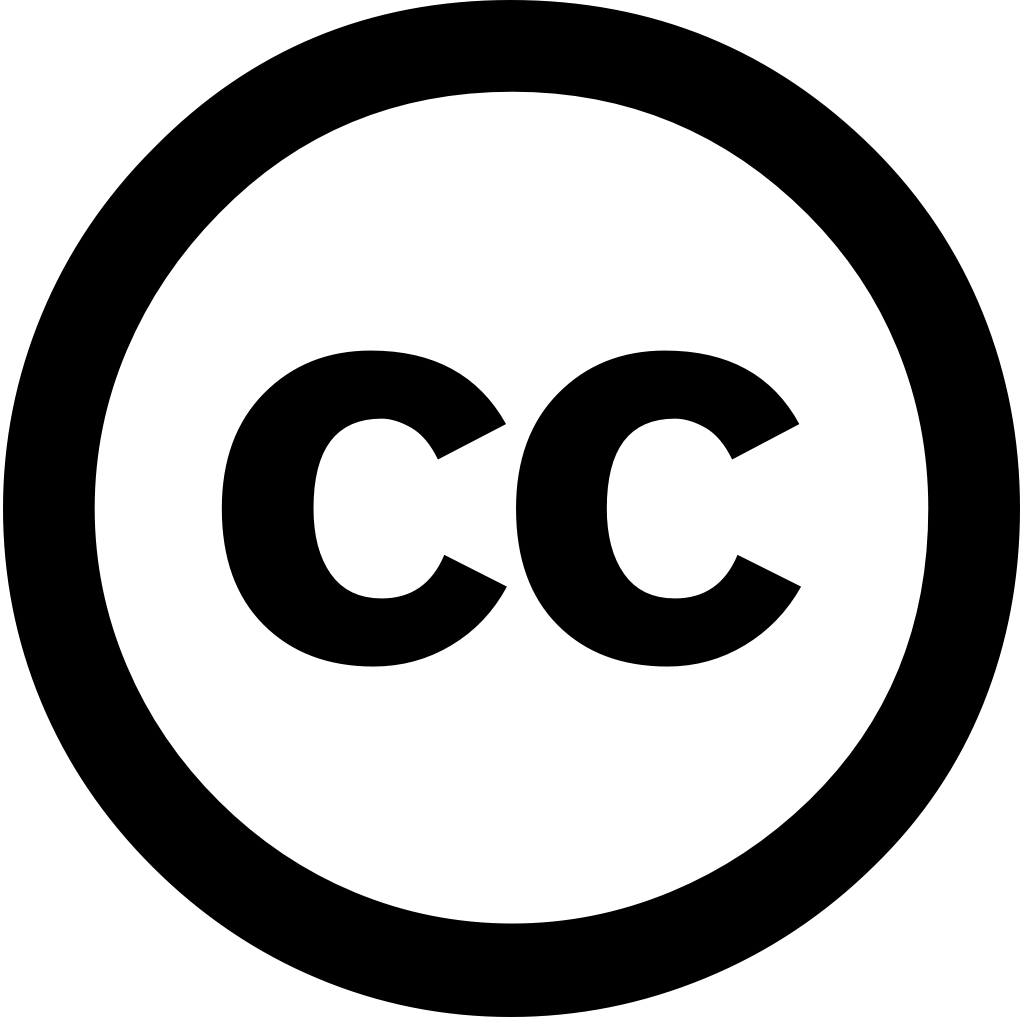
Research Square (Research Square), Год журнала: 2024, Номер unknown
Опубликована: Дек. 20, 2024
Язык: Английский
Research Square (Research Square), Год журнала: 2024, Номер unknown
Опубликована: Дек. 20, 2024
Язык: Английский
PeerJ Computer Science, Год журнала: 2024, Номер 10, С. e2128 - e2128
Опубликована: Июнь 17, 2024
Fog computing has emerged as a prospective paradigm to address the computational requirements of IoT applications, extending capabilities cloud network edge. Task scheduling is pivotal in enhancing energy efficiency, optimizing resource utilization and ensuring timely execution tasks within fog environments. This article presents comprehensive review advancements task methodologies for systems, covering priority-based, greedy heuristics, metaheuristics, learning-based, hybrid nature-inspired heuristic approaches. Through systematic analysis relevant literature, we highlight strengths limitations each approach identify key challenges facing scheduling, including dynamic environments, heterogeneity, scalability, constraints, security concerns, algorithm transparency. Furthermore, propose future research directions these challenges, integration machine learning techniques real-time adaptation, leveraging federated collaborative developing resource-aware energy-efficient algorithms, incorporating security-aware techniques, advancing explainable AI methodologies. By addressing pursuing directions, aim facilitate development more robust, adaptable, efficient task-scheduling solutions ultimately fostering trust, security, sustainability systems facilitating their widespread adoption across diverse applications domains.
Язык: Английский
Процитировано
8Engineering Technology & Applied Science Research, Год журнала: 2025, Номер 15(1), С. 20372 - 20378
Опубликована: Фев. 2, 2025
Efficient resource allocation in fog computing environments is essential to address the increasing demand for high-performance and adaptable network services. Traditional methods lack granular differentiation based on traffic characteristics often resulting suboptimal bandwidth utilization elevated latency. To enhance efficiency, this study applies a community-based approach leveraging Louvain algorithm dynamically cluster nodes with similar demands. By forming communities latency needs, enables targeted distribution, aligning each community optimized pathways that specific requirements. The results indicate notable performance gains, including 14% increase affecting download reduction by an average of 23% time-sensitive applications. These improvements highlight effectiveness proposed managing diverse demands, improving data flow stability, enhancing overall infrastructures. findings underscore potential support scalable, adaptable, secure management, positioning it as viable solution meet complex needs IoT other distributed systems.
Язык: Английский
Процитировано
0Technologies, Год журнала: 2025, Номер 13(2), С. 72 - 72
Опубликована: Фев. 8, 2025
Surgical waiting lists present significant challenges to healthcare systems, particularly in resource-constrained settings where equitable prioritization and efficient resource allocation are critical. We aim address these issues by developing a novel, dynamic, interpretable framework for prioritizing surgical patients. Our methodology integrates machine learning (ML), stochastic simulations, explainable AI (XAI) capture the temporal evolution of dynamic scores, qp(t), while ensuring transparency decision making. Specifically, we employ Light Gradient Boosting Machine (LightGBM) predictive modeling, simulations account variables competitive interactions, SHapley Additive Explanations (SHAPs) interpret model outputs at both global patient-specific levels. hybrid approach demonstrates strong performance using dataset 205 patients from an otorhinolaryngology (ENT) unit high-complexity hospital Chile. The LightGBM achieved mean squared error (MSE) 0.00018 coefficient determination (R2) value 0.96282, underscoring its high accuracy estimating qp(t). Stochastic effectively captured changes, illustrating that Patient 1’s qp(t) increased 0.50 (at t=0) 1.026 t=10) due growth such as severity urgency. SHAP analyses identified (Sever) most influential variable, contributing substantially non-clinical factors, capacity participate family activities (Lfam), exerted moderating influence. Additionally, our achieves reduction times up 26%, demonstrating effectiveness optimizing prioritization. Finally, strategy combines adaptability interpretability, transparent aligns with evolving patient needs constraints.
Язык: Английский
Процитировано
0Archives of Computational Methods in Engineering, Год журнала: 2025, Номер unknown
Опубликована: Фев. 20, 2025
Язык: Английский
Процитировано
0Expert Systems with Applications, Год журнала: 2025, Номер unknown, С. 127214 - 127214
Опубликована: Март 1, 2025
Язык: Английский
Процитировано
0Information Sciences, Год журнала: 2025, Номер unknown, С. 122238 - 122238
Опубликована: Апрель 1, 2025
Язык: Английский
Процитировано
0Multimedia Tools and Applications, Год журнала: 2024, Номер 83(25), С. 66841 - 66868
Опубликована: Янв. 25, 2024
Язык: Английский
Процитировано
2Journal of Grid Computing, Год журнала: 2024, Номер 22(4)
Опубликована: Сен. 23, 2024
Язык: Английский
Процитировано
2IEEE Access, Год журнала: 2024, Номер 12, С. 74444 - 74455
Опубликована: Янв. 1, 2024
This research presents a novel game theory based model for femtocell power management, engineered to significantly enhance the Signal-to-Interference-plus-Noise Ratio (SINR) while optimizing energy consumption across wireless communication networks. Femtocells, as solution increasing demand high-quality indoor network coverage, face challenges in management and interference mitigation. Our proposed addresses these issues, providing sophisticated algorithmic approach that ensures high SINR levels without proportional increase usage. Through series of simulations, model's performance was evaluated against existing techniques. The results, delineated several tables, revealed consistently achieved often surpassed targeted with modest increments, even at targets. Notably, target 20, sustained 23.62 maintaining reasonable profile. Additionally, exhibited exceptional operational efficiency, characterized by low execution times rapid convergence rates, varying conditions. responsiveness is essential adapting user mobility traffic patterns, particularly dense urban settings during peak usage periods.
Язык: Английский
Процитировано
1EAI Endorsed Transactions on Energy Web, Год журнала: 2024, Номер 11
Опубликована: Июнь 5, 2024
INTRODUCTION: Cloud computing, a still emerging technology, allows customers to pay for services based on usage. It provides internet-based services, whilst virtualization optimizes PC’s available resources. OBJECTIVES: The foundation of cloud computing is the data center, comprising networked computers, cables, electricity components, and various other elements that host store corporate data. In centres, high performance has always been critical concern, but this often comes at cost increased energy consumption. METHODS: most problematic factor reducing power consumption while maintaining service quality balance system efficiency use. Our proposed approach requires comprehensive understanding usage patterns within environment. RESULTS: We examined trends demonstrate with application right optimization principles models, significant savings can be made in centers. During prediction phase, tablet optimization, its 97 % accuracy rate, enables more accurate future forecasts. CONCLUSION: Energy major concern To handle incoming requests fewest resources possible, given increasing demand widespread adoption it essential maintain effective efficient center strategies.
Язык: Английский
Процитировано
1