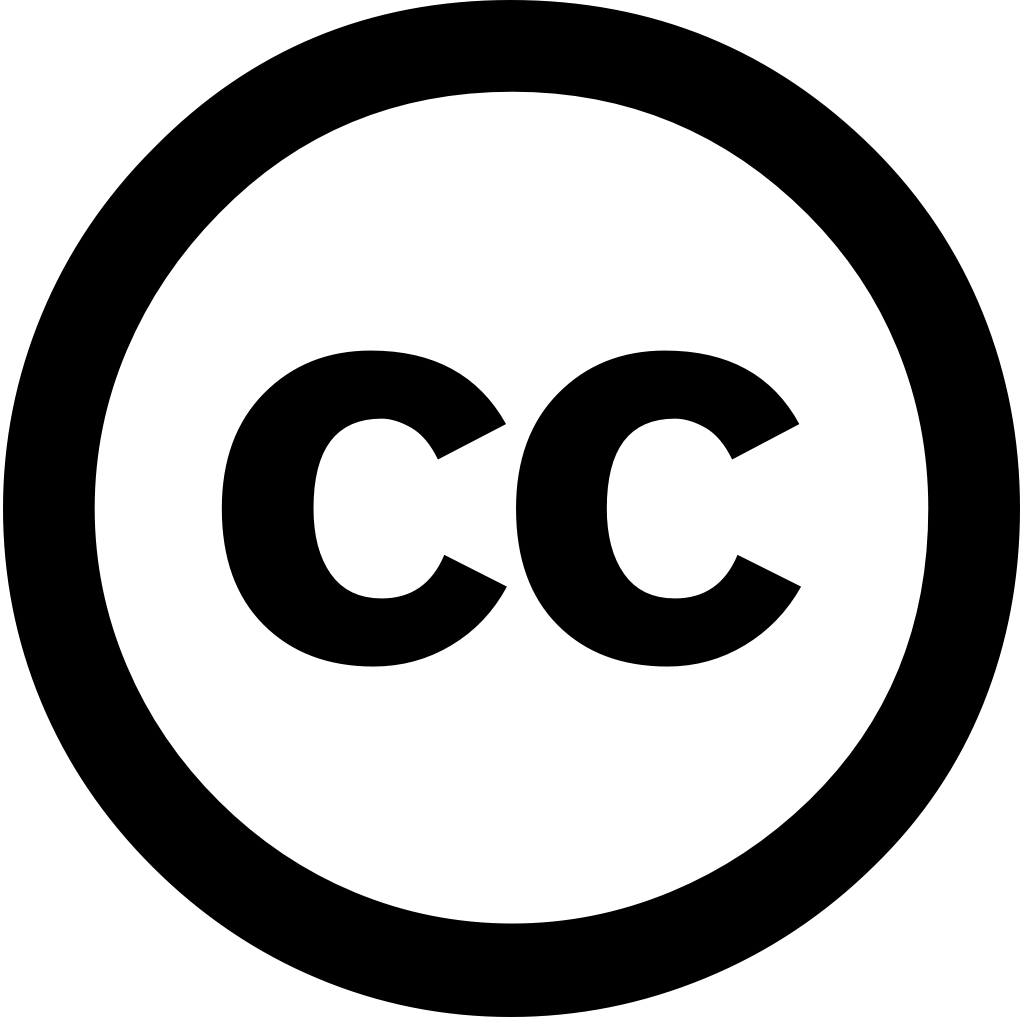
Research Square (Research Square), Год журнала: 2024, Номер unknown
Опубликована: Ноя. 11, 2024
Язык: Английский
Research Square (Research Square), Год журнала: 2024, Номер unknown
Опубликована: Ноя. 11, 2024
Язык: Английский
Aging Clinical and Experimental Research, Год журнала: 2025, Номер 37(1)
Опубликована: Фев. 22, 2025
Abstract Background A variety of unsupervised learning algorithms have been used to phenotype older patients, enabling directed care and personalised treatment plans. However, the ability clusters accurately discriminate for risk may vary depending on methods employed. Aims To compare seven clustering in their develop patient phenotypes that predict health outcomes. Methods Data was collected N = 737 medical inpatients during hospital stay five different types data (ICD-10 codes, ATC drug laboratory, clinic frailty data). We trialled (K-means, K-modes, hierarchical clustering, latent class analysis (LCA), DBSCAN) two graph-based approaches create separate each method datatype. These were as input a random forest classifier eleven outcomes: mortality at one, three, six 12 months, in-hospital falls delirium, length-of-stay, outpatient visits, readmissions three months. Results The overall median area-under-the-curve (AUC) across outcomes (from highest lowest) 0.758 (hierarchical), 0.739 (K-means), 0.722 (KG-Louvain), 0.704 (KNN-Louvain), 0.698 0.694 (DBSCAN) 0.656 (K-modes). Overall, most important type predicting mortality, ICD-10 disease codes readmissions, laboratory falls. Conclusions Clusters created using hierarchical, K-means Louvain community detection identified well-separated consistently associated with age-related adverse Frailty valuable
Язык: Английский
Процитировано
0Aging Clinical and Experimental Research, Год журнала: 2025, Номер 37(1)
Опубликована: Апрель 2, 2025
Fragility fractures occur on porotic bones due to minor trauma and are associated with high rates of disability mortality. To evaluate the ability handgrip strength predict one-year mortality in elderly patients fragility hip fracture. We enrolled aged 65 years older admitted an Italian orthopedic unit. They underwent a comprehensive geriatric assessment, including measurement, all received surgical intervention. Among 322 (median age: 84 years; 75.2% women), rate was 15.5%. According European Working Group Sarcopenia Older People 2 guidelines, 235 subjects (73.0%) exhibited low strength. This group revealed HR: 2.36 (95%CI: 1.06-5.24) for compared adequate (p = 0.036). After adjusting age risk adverse event, through Multidimensional Prognostic Index, HR decreased 1.31 0.56-3.07), lower validity. Our study found slightly than other studies similar samples, probably co-management teams. As main outcome, significantly However, significance diminished when considering possible confounding variables, despite precision model. Low predicts people fractures. Further needed explore influence confounders.
Язык: Английский
Процитировано
0Research Square (Research Square), Год журнала: 2024, Номер unknown
Опубликована: Ноя. 11, 2024
Язык: Английский
Процитировано
0