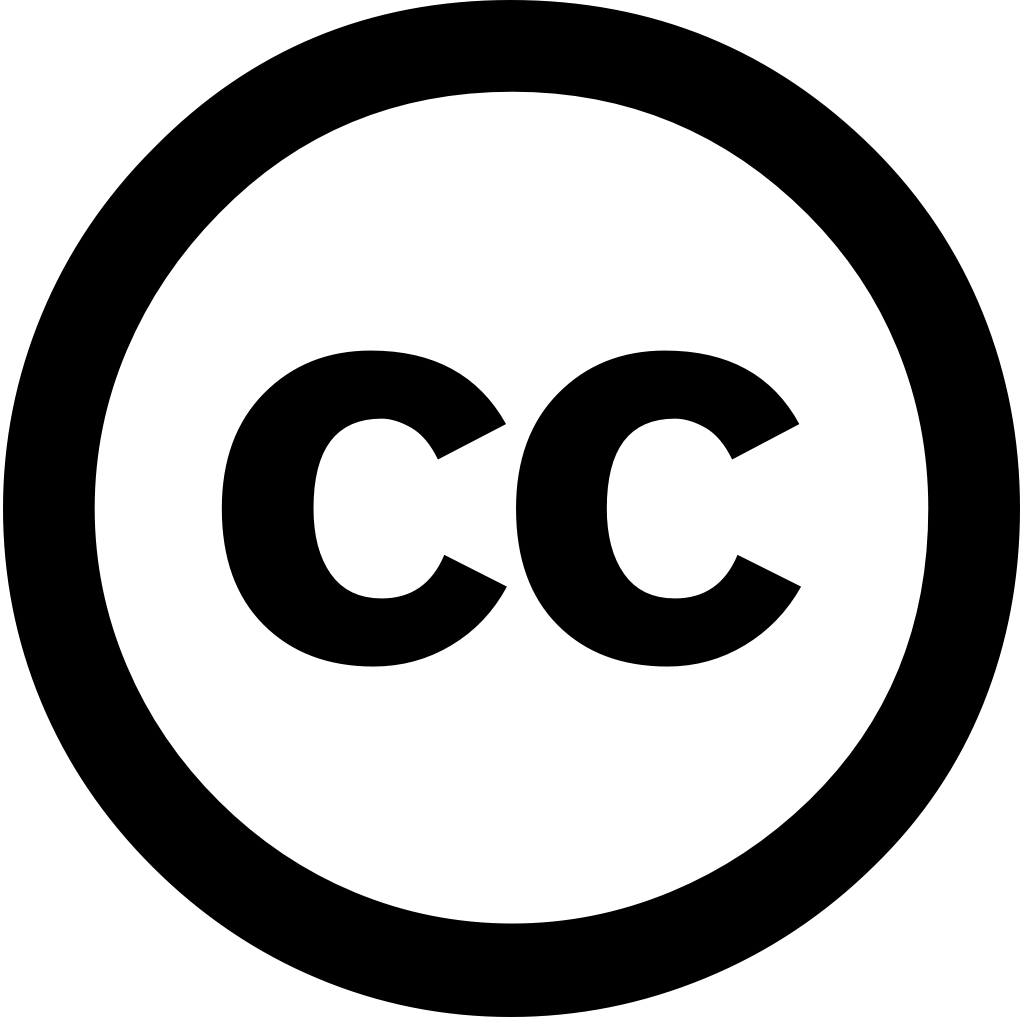
Energy and Buildings, Год журнала: 2024, Номер 307, С. 113964 - 113964
Опубликована: Фев. 2, 2024
Язык: Английский
Energy and Buildings, Год журнала: 2024, Номер 307, С. 113964 - 113964
Опубликована: Фев. 2, 2024
Язык: Английский
Systems, Год журнала: 2023, Номер 11(8), С. 400 - 400
Опубликована: Авг. 2, 2023
The growing interest in unmanned aerial vehicles (UAVs) from both the scientific and industrial sectors has attracted a wave of new researchers substantial investments this expansive field. However, due to wide range topics subdomains within UAV research, newcomers may find themselves overwhelmed by numerous options available. It is therefore crucial for those involved research recognize its interdisciplinary nature connections with other disciplines. This paper presents comprehensive overview field, highlighting recent trends advancements. Drawing on literature reviews surveys, review begins classifying UAVs based their flight characteristics. then provides an current UAVs, utilizing data Scopus database quantify number documents associated each direction interconnections. also explores potential areas further development including communication, artificial intelligence, remote sensing, miniaturization, swarming cooperative control, transformability. Additionally, it discusses aircraft commonly used control techniques, appropriate algorithms research. Furthermore, addresses general hardware software architecture applications, key issues them. open source projects By presenting view aims enhance our understanding rapidly evolving highly area
Язык: Английский
Процитировано
129Knowledge-Based Systems, Год журнала: 2023, Номер 277, С. 110851 - 110851
Опубликована: Июль 29, 2023
Язык: Английский
Процитировано
63IEEE Access, Год журнала: 2024, Номер 12, С. 3768 - 3789
Опубликована: Янв. 1, 2024
Automating the monitoring of industrial processes has potential to enhance efficiency and optimize quality by promptly detecting abnormal events thus facilitating timely interventions. Deep learning, with its capacity discern non-trivial patterns within large datasets, plays a pivotal role in this process. Standard deep learning methods are suitable solve specific task given type data. During training, demands volumes labeled However, due dynamic nature environment, it is impractical acquire large-scale data for standard training every slightly different case anew. transfer offers solution problem. By leveraging knowledge from related tasks accounting variations distributions, framework solves new little or even no additional The approach bypasses need retrain model scratch setup dramatically reduces requirement. This survey first provides an in-depth review examining problem settings classifying prevailing methods. Moreover, we delve into applications context broad spectrum time series anomaly detection prevalent primary domains, e.g., manufacturing process monitoring, predictive maintenance, energy management, infrastructure facility monitoring. We discuss challenges limitations contexts conclude practical directions actionable suggestions address leverage diverse increasingly production environment.
Язык: Английский
Процитировано
43Energy and Buildings, Год журнала: 2024, Номер 305, С. 113903 - 113903
Опубликована: Янв. 11, 2024
Язык: Английский
Процитировано
41Information Fusion, Год журнала: 2024, Номер 113, С. 102601 - 102601
Опубликована: Июль 27, 2024
Язык: Английский
Процитировано
16Image and Vision Computing, Год журнала: 2023, Номер 135, С. 104710 - 104710
Опубликована: Май 18, 2023
Язык: Английский
Процитировано
32Engineering Applications of Artificial Intelligence, Год журнала: 2023, Номер 126, С. 107063 - 107063
Опубликована: Сен. 1, 2023
Язык: Английский
Процитировано
31Internet of Things, Год журнала: 2023, Номер 25, С. 101035 - 101035
Опубликована: Дек. 15, 2023
Язык: Английский
Процитировано
29Journal of Energy Storage, Год журнала: 2023, Номер 76, С. 109891 - 109891
Опубликована: Ноя. 29, 2023
Язык: Английский
Процитировано
28Environmental Science & Technology, Год журнала: 2024, Номер 58(15), С. 6457 - 6474
Опубликована: Апрель 3, 2024
The circular economy (CE) aims to decouple the growth of from consumption finite resources through strategies, such as eliminating waste, circulating materials in use, and regenerating natural systems. Due rapid development data science (DS), promising progress has been made transition toward CE past decade. DS offers various methods achieve accurate predictions, accelerate product sustainable design, prolong asset life, optimize infrastructure needed circulate materials, provide evidence-based insights. Despite exciting scientific advances this field, there still lacks a comprehensive review on topic summarize achievements, synthesize knowledge gained, navigate future research directions. In paper, we try how accelerated CE. We conducted critical where helped with focus four areas including (1) characterizing socioeconomic metabolism, (2) reducing unnecessary waste generation by enhancing material efficiency optimizing (3) extending lifetime repair, (4) facilitating reuse recycling. also introduced limitations challenges current applications discussed opportunities clear roadmap for field.
Язык: Английский
Процитировано
12