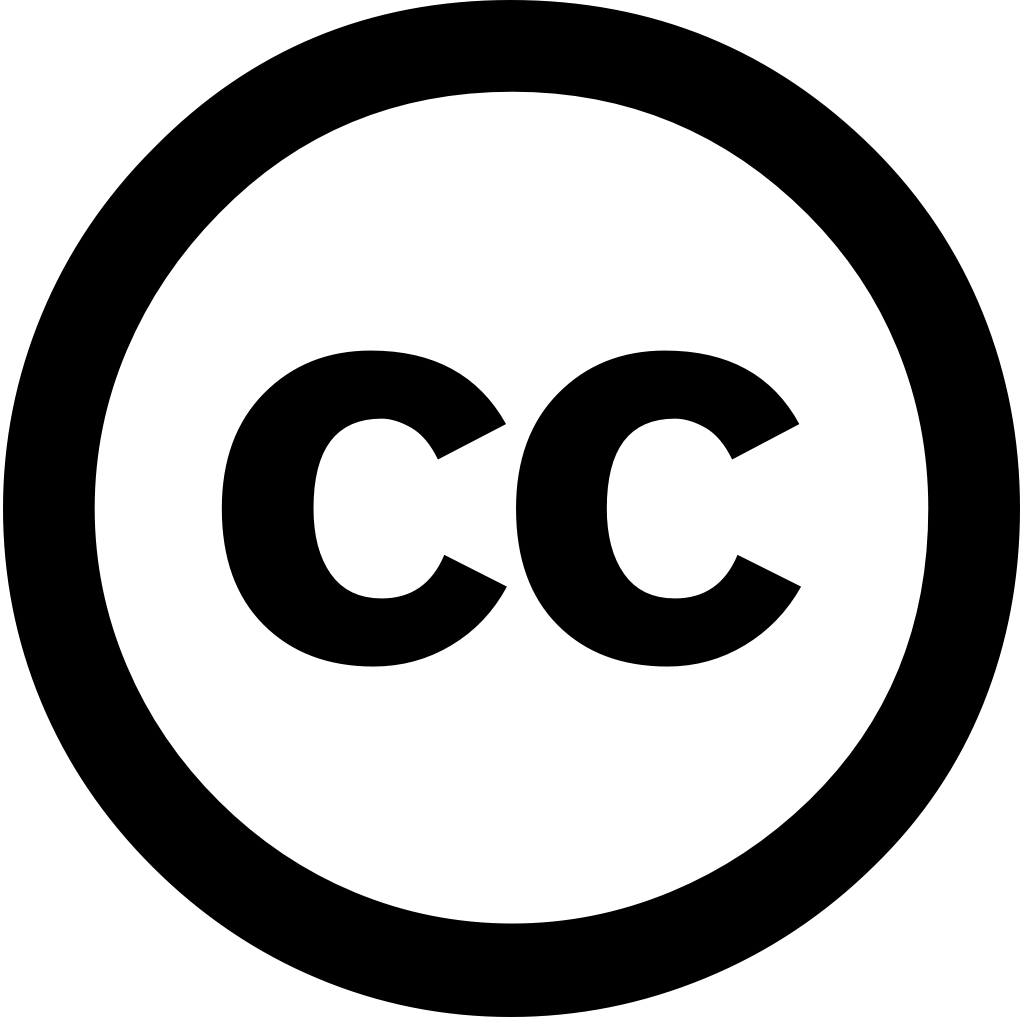
Archives of Computational Methods in Engineering, Год журнала: 2025, Номер unknown
Опубликована: Март 18, 2025
Язык: Английский
Archives of Computational Methods in Engineering, Год журнала: 2025, Номер unknown
Опубликована: Март 18, 2025
Язык: Английский
Measurement, Год журнала: 2024, Номер 235, С. 114912 - 114912
Опубликована: Май 14, 2024
Язык: Английский
Процитировано
24Journal of Materials Science, Год журнала: 2024, Номер 59(31), С. 14095 - 14140
Опубликована: Июль 30, 2024
Abstract Electrospun nanofibers have gained prominence as a versatile material, with applications spanning tissue engineering, drug delivery, energy storage, filtration, sensors, and textiles. Their unique properties, including high surface area, permeability, tunable porosity, low basic weight, mechanical flexibility, alongside adjustable fiber diameter distribution modifiable wettability, make them highly desirable across diverse fields. However, optimizing the properties of electrospun to meet specific requirements has proven be challenging endeavor. The electrospinning process is inherently complex influenced by numerous variables, applied voltage, polymer concentration, solution flow rate, molecular weight polymer, needle-to-collector distance. This complexity often results in variations nanofibers, making it difficult achieve desired characteristics consistently. Traditional trial-and-error approaches parameter optimization been time-consuming costly, they lack precision necessary address these challenges effectively. In recent years, convergence materials science machine learning (ML) offered transformative approach electrospinning. By harnessing power ML algorithms, scientists researchers can navigate intricate space more efficiently, bypassing need for extensive experimentation. holds potential significantly reduce time resources invested producing wide range applications. Herein, we provide an in-depth analysis current work that leverages obtain target nanofibers. examining work, explore intersection ML, shedding light on advancements, challenges, future directions. comprehensive not only highlights processes but also provides valuable insights into evolving landscape, paving way innovative precisely engineered various Graphical abstract
Язык: Английский
Процитировано
17Hybrid Advances, Год журнала: 2024, Номер 6, С. 100242 - 100242
Опубликована: Июль 4, 2024
This systematic review explores the integration of 4D/3D printing technologies with machine learning, shaping a new era manufacturing innovation. The analysis covers wide range research papers, articles, and patents, presenting multidimensional perspective on advancements in additive manufacturing. underscores learning's pivotal role optimizing printing, addressing aspects like design customization, material selection, process control, quality assurance. examination reveals novel techniques enabling fabrication intelligent, self-adaptive structures capable transformation over time. Additionally, investigates use predictive algorithms to enhance efficiency, reliability, sustainability processes. Applications span aerospace, healthcare, architecture, consumer goods, showcasing potential create intricate, personalized, once-unattainable functional products. synergy between learning is poised unlock horizons, rapid responses market demands challenges. In summary, this provides comprehensive overview current state optimization through highlighting transformative interdisciplinary fusion offering roadmap for future development. It aims inspire innovators, researchers, industries harness powerful combination accelerated evolution processes into 21st century beyond.
Язык: Английский
Процитировано
16Results in Engineering, Год журнала: 2024, Номер 23, С. 102576 - 102576
Опубликована: Июль 17, 2024
Fabric-layered composites play a crucial role in safety and surveillance applications, making it imperative to accurately predict their impact behavior. This research focuses on creating machine-learning model the behavior of fabric-stacked composites, specifically carbon Kevlar fabrics. Low-velocity tests were performed with varying parameters, energy laminate thickness information used train models properties such as force, displacement, absorbed energy. It was observed that force increased by 118.5 % carbon-laminated fibers 175.8 Kevlar-laminated fibers, while hybrid layer showed 101.4 increase upon from 16J. Displacement can affect stability layered structure; thus, similar stacking sequence is less stable than laminated structure. In terms energy, layers increase, fiber absorbs 4.8 times more structures absorb 3 at higher Furthermore, four machine learning investigate identical mixed-layered composites. The displacement predicted accuracy using polynomial regression model, achieving 80 89 accuracy, respectively. support vector approximately 96 accuracy. continuing, experimental results closely matched predictions made other utilized this study. Additionally, importance distinctive features influence performance learning-based interpreted transposed dependency plots. Various failure modes fabric also identified, providing insights enhance stacked materials.
Язык: Английский
Процитировано
11Composite Structures, Год журнала: 2024, Номер 337, С. 118099 - 118099
Опубликована: Апрель 2, 2024
Язык: Английский
Процитировано
10Fibers and Polymers, Год журнала: 2024, Номер 25(8), С. 3115 - 3133
Опубликована: Июль 19, 2024
Язык: Английский
Процитировано
10Sustainable materials and technologies, Год журнала: 2025, Номер unknown, С. e01259 - e01259
Опубликована: Янв. 1, 2025
Язык: Английский
Процитировано
1Journal of Materials Engineering and Performance, Год журнала: 2025, Номер unknown
Опубликована: Март 5, 2025
Язык: Английский
Процитировано
1Journal of Materials Research and Technology, Год журнала: 2023, Номер 27, С. 4074 - 4087
Опубликована: Ноя. 1, 2023
Currently, the research efforts on machining indices such as tool wear, surface roughness, power consumption etc. is well reported in literature, but energy analysis based material removal methods and machine learning has received comparatively little attention. Therefore, present work deals with simultaneous reduction of specific cutting sustainable Inconel 601 alloy different models. The studies were conducted using dry, minimum quantity lubrication (MQL), nano-MQL, cryogenic, hybrid cooling (cryo-nano-MQL). (SCE) values calculated data obtained from rate. Subsequently, SCE employed to construct crucial maps, which are then utilized several sophisticated models, including Multiple Linear Regression, Lasso Bayesian Ridge Voting Regressor, facilitate predictive modeling outcomes. findings study indicate that model exhibits a reduced error rate closely aligned R2 value when compared other prediction Moreover, novelty, nanoparticles addition into (cryo + nano MQL) also showed better performance 0.3 % less than only cryo method previously used former studies.
Язык: Английский
Процитировано
17Food and Bioprocess Technology, Год журнала: 2024, Номер unknown
Опубликована: Май 30, 2024
Язык: Английский
Процитировано
7