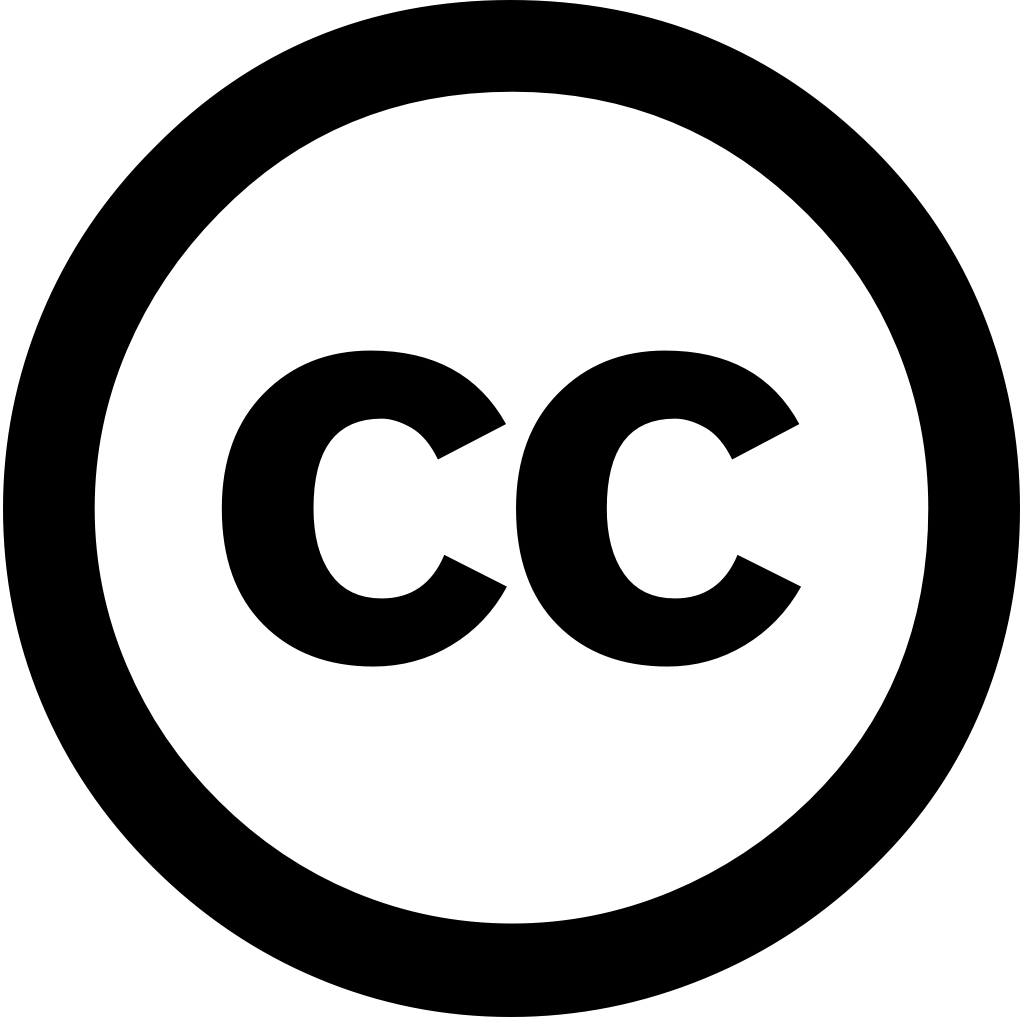
Research Square (Research Square), Год журнала: 2024, Номер unknown
Опубликована: Ноя. 25, 2024
Язык: Английский
Research Square (Research Square), Год журнала: 2024, Номер unknown
Опубликована: Ноя. 25, 2024
Язык: Английский
International Journal of Mechanical Sciences, Год журнала: 2024, Номер 279, С. 109472 - 109472
Опубликована: Окт. 1, 2024
Язык: Английский
Процитировано
8International Journal of Mechanical Sciences, Год журнала: 2025, Номер unknown, С. 110136 - 110136
Опубликована: Март 1, 2025
Язык: Английский
Процитировано
1Applied Physics Reviews, Год журнала: 2024, Номер 11(2)
Опубликована: Апрель 24, 2024
State-of-the-art biomedical applications such as targeted drug delivery and laparoscopic surgery are extremely challenging because of the small length scales, requirements wireless manipulation, operational accuracy, precise localization. In this regard, miniaturized magnetic soft robotic swimmers (MSRS) attractive candidates since they offer a contactless mode operation for path maneuvering. Inspired by nature, researchers have designed these small-scale intelligent machines to demonstrate enhanced swimming performance through viscous fluidic media using different modes propulsion. review paper, we identify classify nature-inspired basic that been optimized over large evolutionary timescales. For example, ciliary like Paramecium Coleps covered with tiny hairlike filaments (cilia) beat rhythmically coordinated wave movements propulsion gather food. Undulatory spermatozoa midge larvae use traveling body waves push surrounding fluid effective highly environments. Helical bacteria rotate their slender whiskers (flagella) locomotion stagnant viscid fluids. Essentially, all three employ nonreciprocal motion achieve spatial asymmetry. We provide mechanistic understanding magnetic-field-induced spatiotemporal symmetry-breaking principles adopted MSRS at scales. Furthermore, theoretical computational tools can precisely predict magnetically driven deformation fluid–structure interaction discussed. Here, present holistic descriptive recent developments in smart material systems covering wide spectrum fabrication techniques, design, applications, strategies, actuation, modeling approaches. Finally, future prospects promising systems. Specifically, synchronous tracking noninvasive imaging external agents during vivo clinical still remains daunting task. experimental demonstrations mostly limited vitro ex phantom models where dynamics testing conditions quite compared conditions. Additionally, multi-shape morphing multi-stimuli-responsive modalities active structures demand further advancements 4D printing avenues. Their multi-state configuration an solid-fluid continuum would require development multi-scale models. Eventually, adding multiple levels intelligence enhance adaptivity, functionalities, reliability critical applications.
Язык: Английский
Процитировано
6Acta Mechanica Sinica, Год журнала: 2024, Номер 41(1)
Опубликована: Авг. 22, 2024
Язык: Английский
Процитировано
4Materials Science and Engineering A, Год журнала: 2025, Номер 924, С. 147792 - 147792
Опубликована: Янв. 5, 2025
Язык: Английский
Процитировано
0Опубликована: Янв. 1, 2025
Язык: Английский
Процитировано
0Scientific Reports, Год журнала: 2025, Номер 15(1)
Опубликована: Фев. 12, 2025
Aiming at the problem that it is difficult to accurately predict wellbore trajectory under complex geological conditions, NOA-LSTM-FCNN prediction method for steering drilling proposed by combining NOA, LSTM and FCNN. This adopts layer receive input data capture long-term dependencies within data, extracting important information. The FCNN performs nonlinear mapping on output of further extracts relevant features enhance accuracy. NOA employed hyperparameter optimization LSTM-FCNN model. experimental results show effect better than other methods. Taking well deviation angle H21 as an example, compared with traditional machine learning methods (LR, SVM BP) deep (CNN, GRU), evaluation index R² this was improved 0.17887, 0.03129, 0.0259, 0.00054, 0.00032 0.00031 respectively, showing significant accuracy advantages strong adaptability. In addition, applies various types effectively enhancing capabilities conditions.
Язык: Английский
Процитировано
0International Journal of Mechanical Sciences, Год журнала: 2024, Номер unknown, С. 109840 - 109840
Опубликована: Ноя. 1, 2024
Язык: Английский
Процитировано
1Applied Mathematical Modelling, Год журнала: 2024, Номер unknown, С. 115922 - 115922
Опубликована: Дек. 1, 2024
Язык: Английский
Процитировано
1Research Square (Research Square), Год журнала: 2024, Номер unknown
Опубликована: Ноя. 25, 2024
Язык: Английский
Процитировано
0