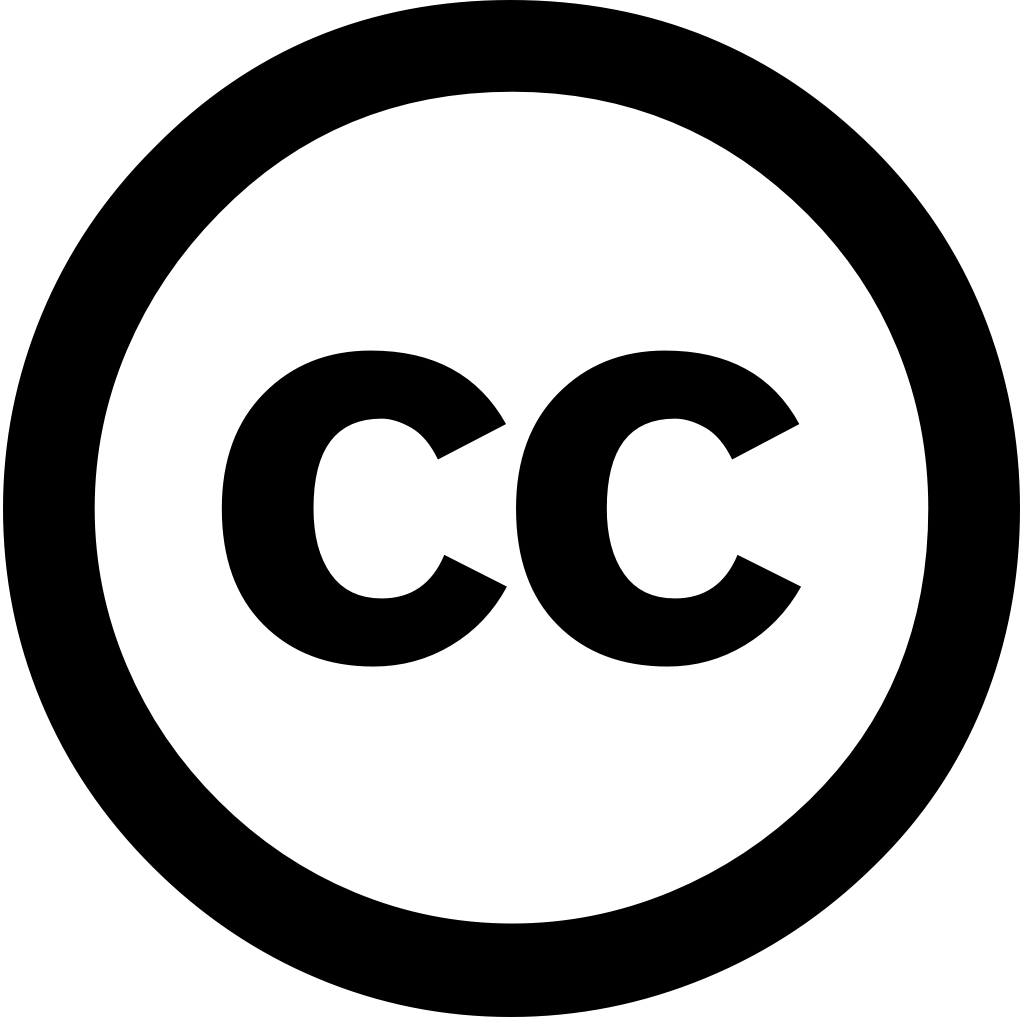
Engineering Applications of Computational Fluid Mechanics, Год журнала: 2024, Номер 18(1)
Опубликована: Ноя. 6, 2024
In recent decades, securing drinkable water sources has become a pressing concern for populations in various regions worldwide. Therefore, to address the growing need potable water, contemporary purification technologies can be employed convert saline into supplies. prediction of important parameters desalination plants is key task designing and implementing these facilities. this regard, artificial intelligence techniques have proven powerful assets field. These methods offer an expedited effective means estimating parameters, thus catalyzing their implementation real-world scenarios. study, predictive accuracy six different machine learning models, including Natural Gradient-based Boosting (NGBoost), Adaptive (AdaBoost), Categorical (CatBoost), Support vector regression (SVR), Gaussian Process Regression (GPR), Extremely Randomized Tree (ERT) was evaluated modelling parameter permeate flow as element system efficiency, energy consumption, quality using input combinations feed salt concentration, condenser inlet temperature, rate, evaporator temperature. The next phase research SHAP interpretability method illustrate impact individual variables on model's output. Moreover, performance developed frameworks set five dependable statistical measures: RMSE, NS, MAE, MAPE R2. indicators were utilized provide robust gauging precision forecasts. A comparative analysis outcomes, measured by RMSE criteria, revealed that SVR technique (RMSE = 0.125 L/(h·m2)) exhibited superior compared NGBoost 0.163 L/(h·m2)), AdaBoost 0.219 CatBoost 0.149 GPR 0.156 ERT 0.167 methodologies predicting rates. outcomes obtained during evaluation stage demonstrated efficacy algorithm enhancing forecasts, utilizing relevant variables.
Язык: Английский