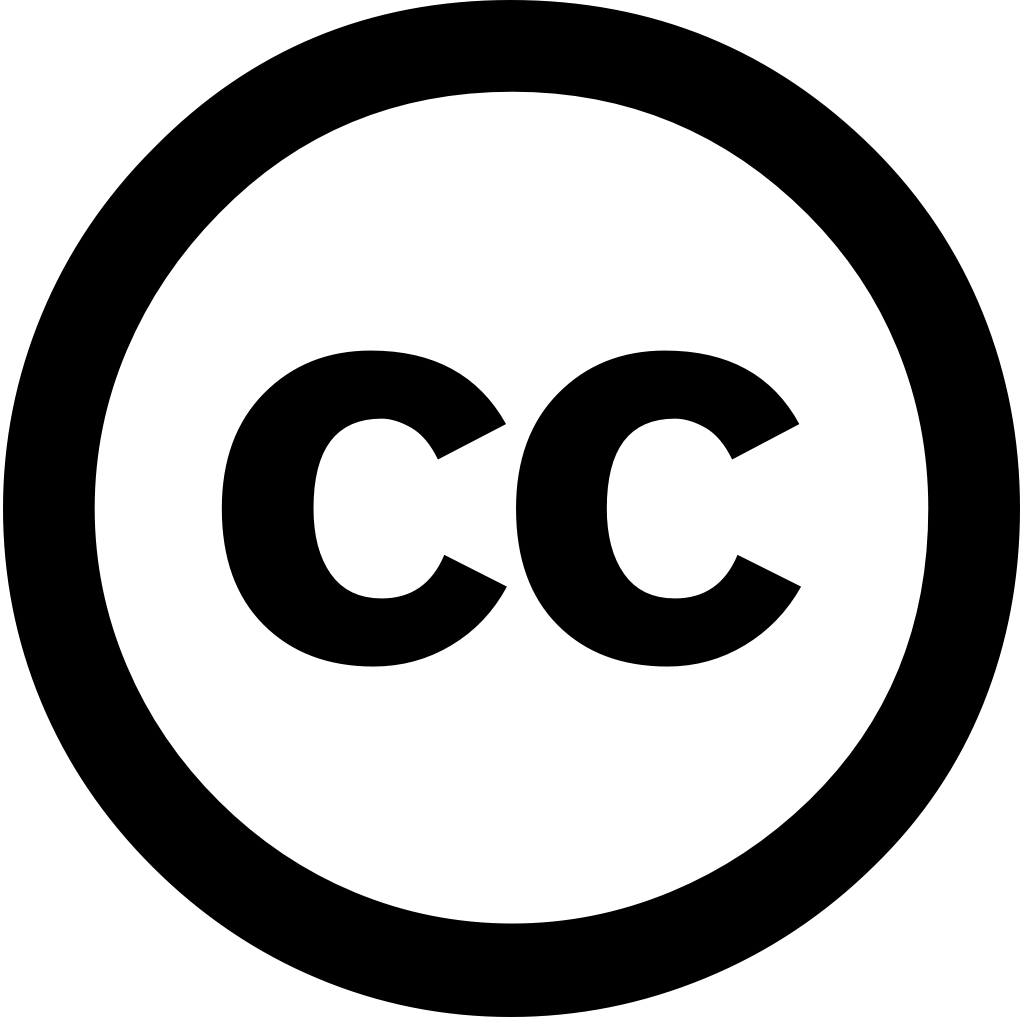
Petroleum Science, Год журнала: 2025, Номер unknown
Опубликована: Фев. 1, 2025
Язык: Английский
Petroleum Science, Год журнала: 2025, Номер unknown
Опубликована: Фев. 1, 2025
Язык: Английский
Fuel, Год журнала: 2023, Номер 351, С. 128913 - 128913
Опубликована: Июнь 10, 2023
Язык: Английский
Процитировано
112Energy Reports, Год журнала: 2022, Номер 8, С. 15595 - 15616
Опубликована: Ноя. 1, 2022
While carbon capture and sequestration (CCS) has garnered many headlines in the recent past, its wider applicability on an industrial scale is yet to be explored due a plethora of challenges, primarily involving short-term financial attractiveness CCS projects. However, represents crucial technology for hydrocarbon industry extend utility this age where environmental, social governance (ESG) criteria applied by institutes restrict access capital industry. opportunities are varied require more detailed understanding various processes them successful their desired operational parameters. Alternatively, captured dioxide (CO2) may utilized enhanced oil recovery (EOR) or methane production, which referred as capture, utilization storage (CCUS). Hence, primary purpose review introduce research audience process CCUS providing brief introduction potential. The article discusses multiple technologies studies Technology Readiness Level (TRL) values each these order give better outlook about being employed contemporary world. Also, viability subsurface mobilization applications would adverse conditions. Finally, highlight implementation proposed methods transition towards CO2 gas been elaborated upon. Apart from reviewing literature related technologies, authors have upon economic aspects technologies. There also studied impact different industries like chemical industry, cement etc. thus, paper intended act guide policy makers future course action with regards reducing footprint oil, till equitable sources energy replace it.
Язык: Английский
Процитировано
82International Journal of Pressure Vessels and Piping, Год журнала: 2023, Номер 206, С. 105033 - 105033
Опубликована: Июль 22, 2023
Язык: Английский
Процитировано
81Sensors, Год журнала: 2023, Номер 23(6), С. 3226 - 3226
Опубликована: Март 17, 2023
Pipelines play a significant role in liquid and gas resource distribution. Pipeline leaks, however, result severe consequences, such as wasted resources, risks to community health, distribution downtime, economic loss. An efficient autonomous leakage detection system is clearly required. The recent leak diagnosis capability of acoustic emission (AE) technology has been well demonstrated. This article proposes machine learning-based platform for various pinhole-sized leaks using the AE sensor channel information. Statistical measures, kurtosis, skewness, mean value, square, root square (RMS), peak standard deviation, entropy, frequency spectrum features, were extracted from signal features train learning models. adaptive threshold-based sliding window approach was used retain properties both bursts continuous-type emissions. First, we collected three datasets 11 time domain 14 one-second each data category. measurements their associated statistics transformed into feature vectors. Subsequently, these utilized training evaluating supervised models detect leaks. Several widely known classifiers, neural networks, decision trees, random forests, k-nearest neighbors, evaluated four regarding water leakages at different pressures pinhole sizes. We achieved an exceptional overall classification accuracy 99%, providing reliable effective results that are suitable implementation proposed platform.
Язык: Английский
Процитировано
54Process Safety and Environmental Protection, Год журнала: 2023, Номер 174, С. 460 - 472
Опубликована: Апрель 12, 2023
Язык: Английский
Процитировано
44Applied Energy, Год журнала: 2024, Номер 359, С. 122656 - 122656
Опубликована: Янв. 18, 2024
Язык: Английский
Процитировано
21Sensors, Год журнала: 2025, Номер 25(4), С. 1112 - 1112
Опубликована: Фев. 12, 2025
Effective leak detection and size identification are essential for maintaining the operational safety, integrity, longevity of industrial pipelines. Traditional methods often suffer from high noise sensitivity, limited adaptability to non-stationary signals, excessive computational costs, which limits their feasibility real-time monitoring applications. This study presents a novel acoustic emission (AE)-based pipeline approach, integrating Empirical Wavelet Transform (EWT) adaptive frequency decomposition with customized one-dimensional DenseNet architecture achieve precise classification. The methodology begins EWT-based signal segmentation, isolates meaningful bands enhance leak-related feature extraction. To further improve quality, thresholding denoising techniques applied, filtering out low-amplitude while preserving critical diagnostic information. denoised signals processed using DenseNet-based deep learning model, combines convolutional layers densely connected propagation extract fine-grained temporal dependencies, ensuring accurate classification presence severity. Experimental validation was conducted on real-world AE data collected under controlled non-leak conditions at varying pressure levels. proposed model achieved an exceptional accuracy 99.76%, demonstrating its ability reliably differentiate between normal operation multiple severities. method effectively reduces costs robust performance across diverse operating environments.
Язык: Английский
Процитировано
3Engineering Applications of Computational Fluid Mechanics, Год журнала: 2023, Номер 17(1)
Опубликована: Янв. 17, 2023
This paper proposes a leak detection and size identification technique in fluid pipelines based on new leak-sensitive feature called the vulnerability index (VI) 1-D convolutional neural network (1D-CNN). The acoustic emission hit (AEH) features can differentiate between normal operating conditions of pipeline. However, multiple sources hits, such as pressure joints, interference noises, flange vibrations, leaks pipeline, make less sensitive toward To address this issue, are first extracted from (AE) signal using sliding window with an adaptive threshold. Since distribution changes according to pipeline working conditions, newly developed multiscale Mann–Whitney test (MMU-Test) is applied obtain feature, which shows pipeline's susceptibility conditions. Finally, provided input 1-D-CNN for identification, whose experimental results show higher accuracy compared reference state-of-the-art methods under variable
Язык: Английский
Процитировано
38Expert Systems with Applications, Год журнала: 2023, Номер 231, С. 120542 - 120542
Опубликована: Май 31, 2023
Язык: Английский
Процитировано
31Journal of Cleaner Production, Год журнала: 2024, Номер 438, С. 140467 - 140467
Опубликована: Янв. 1, 2024
Язык: Английский
Процитировано
15