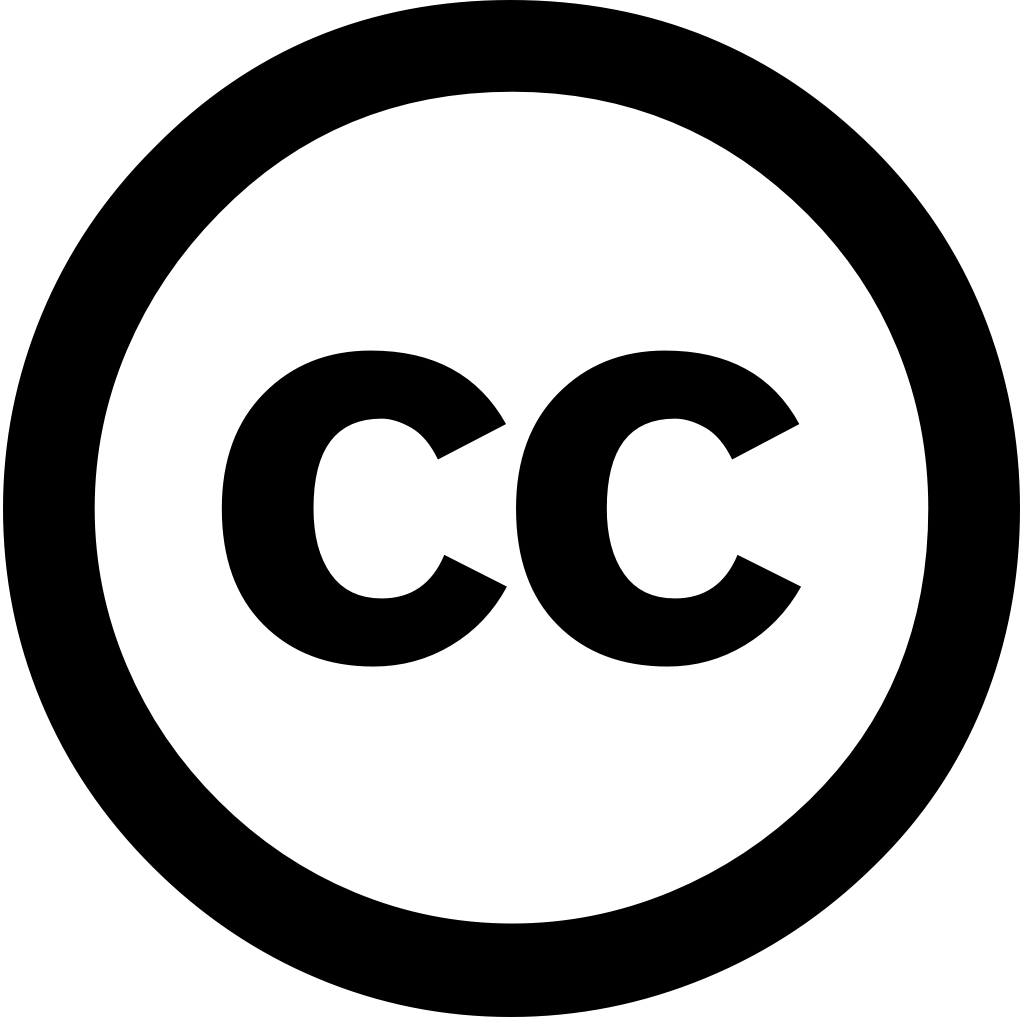
Water Research X, Год журнала: 2024, Номер 26, С. 100280 - 100280
Опубликована: Ноя. 22, 2024
Язык: Английский
Water Research X, Год журнала: 2024, Номер 26, С. 100280 - 100280
Опубликована: Ноя. 22, 2024
Язык: Английский
International Journal of Hydrogen Energy, Год журнала: 2024, Номер 67, С. 136 - 149
Опубликована: Апрель 19, 2024
This review article provides a comprehensive overview of the fundamentals, modelling approaches, experimental studies, and challenges associated with hydrogen gas flow in pipelines. It elucidates key aspects flow, including density, compressibility factor, other relevant properties crucial for understanding its behavior Equations state are discussed detail, highlighting their importance accurately modeling flow. In subsequent sections, one-dimensional three-dimensional techniques distribution networks localized involving critical components explored. Emphasis is placed on transient friction losses, leakage characteristics, shedding light complexities pipeline transportation. Experimental studies investigating transportation dynamics outlined, focusing impact surrounding environments safety parameters. The solutions repurposing natural pipelines transport discussed, along influence material Identified research gaps underscore need further investigation into areas such as behavior, mitigation strategies, development advanced techniques. Future perspectives address growing demand clean energy carrier evolving landscape hydrogen-based systems.
Язык: Английский
Процитировано
24Applied Energy, Год журнала: 2023, Номер 353, С. 122155 - 122155
Опубликована: Окт. 27, 2023
Язык: Английский
Процитировано
40International Journal of Hydrogen Energy, Год журнала: 2023, Номер 53, С. 535 - 549
Опубликована: Дек. 18, 2023
Язык: Английский
Процитировано
23Process Safety and Environmental Protection, Год журнала: 2024, Номер 188, С. 363 - 373
Опубликована: Май 28, 2024
Язык: Английский
Процитировано
11Process Safety and Environmental Protection, Год журнала: 2024, Номер 189, С. 549 - 560
Опубликована: Июнь 27, 2024
Язык: Английский
Процитировано
9Measurement, Год журнала: 2025, Номер unknown, С. 116857 - 116857
Опубликована: Янв. 1, 2025
Язык: Английский
Процитировано
1Ocean Engineering, Год журнала: 2024, Номер 294, С. 116658 - 116658
Опубликована: Янв. 6, 2024
Язык: Английский
Процитировано
8Renewable and Sustainable Energy Reviews, Год журнала: 2024, Номер 207, С. 114898 - 114898
Опубликована: Сен. 6, 2024
Язык: Английский
Процитировано
8Expert Systems with Applications, Год журнала: 2024, Номер 246, С. 123200 - 123200
Опубликована: Янв. 10, 2024
In this paper, we introduce an Adaptable and Interpretable Framework for Anomaly Detection (AID) designed industrial systems utilizing IoT data streams on top of well-established SCADA systems. AID leverages dynamic conditional probability distribution modeling to capture the normal operation isolate root causes anomalies at level individual inputs. The self-supervised framework dynamically updates parameters underlying model, allowing it adapt non-stationarity. interprets as significant deviations from probability, encompassing interactions well both spatial temporal irregularities by exposing them features. Crucially, provides operating limits integrate with existing alarm handling mechanisms in SCADA-based Two industrial-scale case studies demonstrate AID's capabilities. first study showcases effectiveness energy storage system, adapting changes, setting context-aware SCADA, ability leverage a physics-based model. second monitors battery module temperatures, where identifies hardware faults, emphasizing its relevance safety. A benchmark evaluation real shows that delivers comparable performance other self-learning adaptable anomaly detection methods, advancement diagnostic capabilities improved system reliability performance.
Язык: Английский
Процитировано
6Reliability Engineering & System Safety, Год журнала: 2024, Номер 252, С. 110465 - 110465
Опубликована: Авг. 28, 2024
This study introduces an unsupervised machine learning framework for damage detection and localization in Structural Health Monitoring (SHM), leveraging dynamic graph convolutional neural networks Transformer networks. approach is specifically tailored to overcome the challenge of limited labeled data SHM, enabling precise analysis feature synthesis from sensor-derived time series accurate identification. Incorporating a novel 'localization score' enhances framework's precision pinpointing structural damages by integrating data-driven insights with physics-informed understanding dynamics. Extensive validations on diverse structures, including benchmark steel structure real-world cable-stayed bridge, underscore effectiveness anomaly localization, showcasing its potential safeguard critical infrastructure through advanced data-effective techniques.
Язык: Английский
Процитировано
5