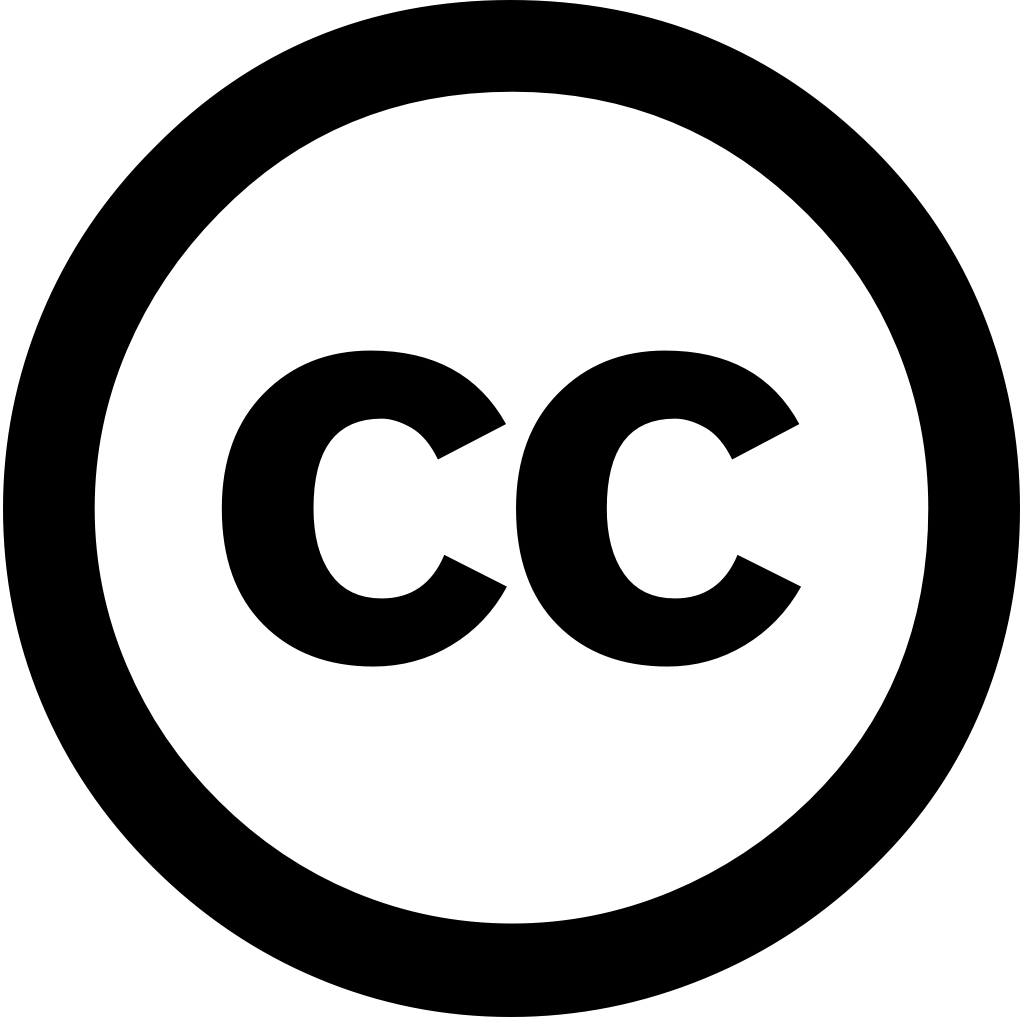
Environmental Science Advances, Год журнала: 2024, Номер 4(1), С. 125 - 132
Опубликована: Окт. 29, 2024
An ML model optimized the performance of an SBR WWTP when applied to OSL scenarios, ensuring regulatory compliance.
Язык: Английский
Environmental Science Advances, Год журнала: 2024, Номер 4(1), С. 125 - 132
Опубликована: Окт. 29, 2024
An ML model optimized the performance of an SBR WWTP when applied to OSL scenarios, ensuring regulatory compliance.
Язык: Английский
Engineering, Год журнала: 2024, Номер 36, С. 21 - 35
Опубликована: Май 1, 2024
The digital twins concept enhances modeling and simulation through the integration of real-time data feedback. This review elucidates foundational elements twins, covering their concept, entities, domains, key technologies. More specifically, we investigate transformative potential for wastewater treatment engineering sector. Our discussion highlights application to plants (WWTPs) sewage networks, hardware (i.e., facilities pipes, sensors water quality activated sludge, hydrodynamics, power consumption), software knowledge-based data-driven models, mechanistic hybrid control methods, Internet Things). Furthermore, two cases are provided, followed by an assessment current challenges in perspectives on WWTPs. serves as essential primer engineers navigating paradigm shift.
Язык: Английский
Процитировано
12Desalination and Water Treatment, Год журнала: 2024, Номер 319, С. 100524 - 100524
Опубликована: Июнь 11, 2024
This study investigates the use of developed machine learning techniques for modeling performance AlHayer, Saudi Arabia, wastewater treatment plant (ALWTP). Three physio-chemical characteristics were measured and predicted, including chemical oxygen demand (COD), biological (BOD), suspended solids (SS), at ALWTP. The pre-evaluation collected data revealed effective capabilities ALWTP removal solids, organic, nutrient pollutants. To estimate ALWATP, four evaluated compared. Logistic regression (LR), random forest (RF), gradient boosting (GB), support vector (SVR) designed. evaluation proposed models showed RF outperformed other estimating COD SS with accuracy 91 % 95 in terms coefficient determination (R2); however, GB was found best, 92 %, detecting BOD ALWATP. indicates ensemble models, GB, can be considered a superiority soft solution plant.
Язык: Английский
Процитировано
10Water, Год журнала: 2025, Номер 17(3), С. 310 - 310
Опубликована: Янв. 23, 2025
When confronted with different influent conditions, WWTPs often lack targeted and effective operational control strategies. For the three typical scenarios of low C/N, water temperature high temperature, 441 carbon source dosage DO concentration coordination strategies were designed under premise ensuring effluent quality meets standard. The purpose was to provide clear guidance for efficient operation in scenarios. To determine optimal strategy, prediction model based on LSTM GRU constructed testing. results showed that: (1) LSTM-GRU is better than SVR RF predicting COD TN; (2) In C/N scenario, should be controlled between 0.23 t/h 0.26 t/h, ranging from 2.0 mg/L 2.6 mg/L; (3) 0.25 0.27 2.8 (4) 0.20 2.5 mg/L.
Язык: Английский
Процитировано
1Desalination, Год журнала: 2024, Номер 586, С. 117849 - 117849
Опубликована: Июнь 15, 2024
Язык: Английский
Процитировано
7Chemosphere, Год журнала: 2024, Номер 352, С. 141472 - 141472
Опубликована: Фев. 19, 2024
Язык: Английский
Процитировано
6E3S Web of Conferences, Год журнала: 2025, Номер 605, С. 03006 - 03006
Опубликована: Янв. 1, 2025
There are some challenges firms the wastewater treatment, numerous hurdles concerning enhancement of energy efficiency, compliance with increasingly stringent water quality regulations, and maximizing resource recovery opportunities. In recent years, computational models have garnered acknowledgment as potent instruments for tackling these various challenges, bolstering operational economic effectiveness treatment plants (“WWTPs”). Also, review discusses application (AI) algorithms on (WWTPs), predicting (“WWTP”) effluent properties, inflows, anomaly detecting, optimization. The critical gaps future directions in including explain ability data-driven or transfer Learning processes reinforcement learning, also addressed.
Язык: Английский
Процитировано
0Chemical Engineering Journal, Год журнала: 2025, Номер unknown, С. 160872 - 160872
Опубликована: Фев. 1, 2025
Язык: Английский
Процитировано
0Waste Management, Год журнала: 2024, Номер 188, С. 95 - 106
Опубликована: Авг. 10, 2024
Язык: Английский
Процитировано
3Scientific Reports, Год журнала: 2024, Номер 14(1)
Опубликована: Дек. 28, 2024
With the accelerated urbanization and economic development in Northwest China, efficiency of urban wastewater treatment importance water quality management have become increasingly significant. This work aims to explore carbon reduction mechanisms China alleviate resource pressure. By utilizing online monitoring data from pilot systems, it conducts an in-depth analysis impacts different processes on parameters. pays particular attention their impact key indicators such as Chemical Oxygen Demand (COD), NH4+-N, Total Phosphorus (TP), Nitrogen (TN), application predictive models. The first establishes a Random Forest Regression (RFR) model. RFR algorithm integrates Bagging ensemble learning random subspace theory construct multiple decision trees aggregate predictions, thereby enhancing model's prediction accuracy stability. Using bootstrap sampling, model generates training subsets original randomly selects variables regression trees. Its performance predicting various is then evaluated. results show that exhibits excellent performance, achieving high levels stability for all indicators. For example, R2 COD 0.99954, while values TP, TN predictions reach 0.99989. Compared five other models, demonstrates best across indicator predictions. provides critical support optimizing technologies developing policies. These findings also offer essential theoretical empirical insights future improvement decision-making.
Язык: Английский
Процитировано
3Cleaner Water, Год журнала: 2024, Номер unknown, С. 100058 - 100058
Опубликована: Дек. 1, 2024
Язык: Английский
Процитировано
2