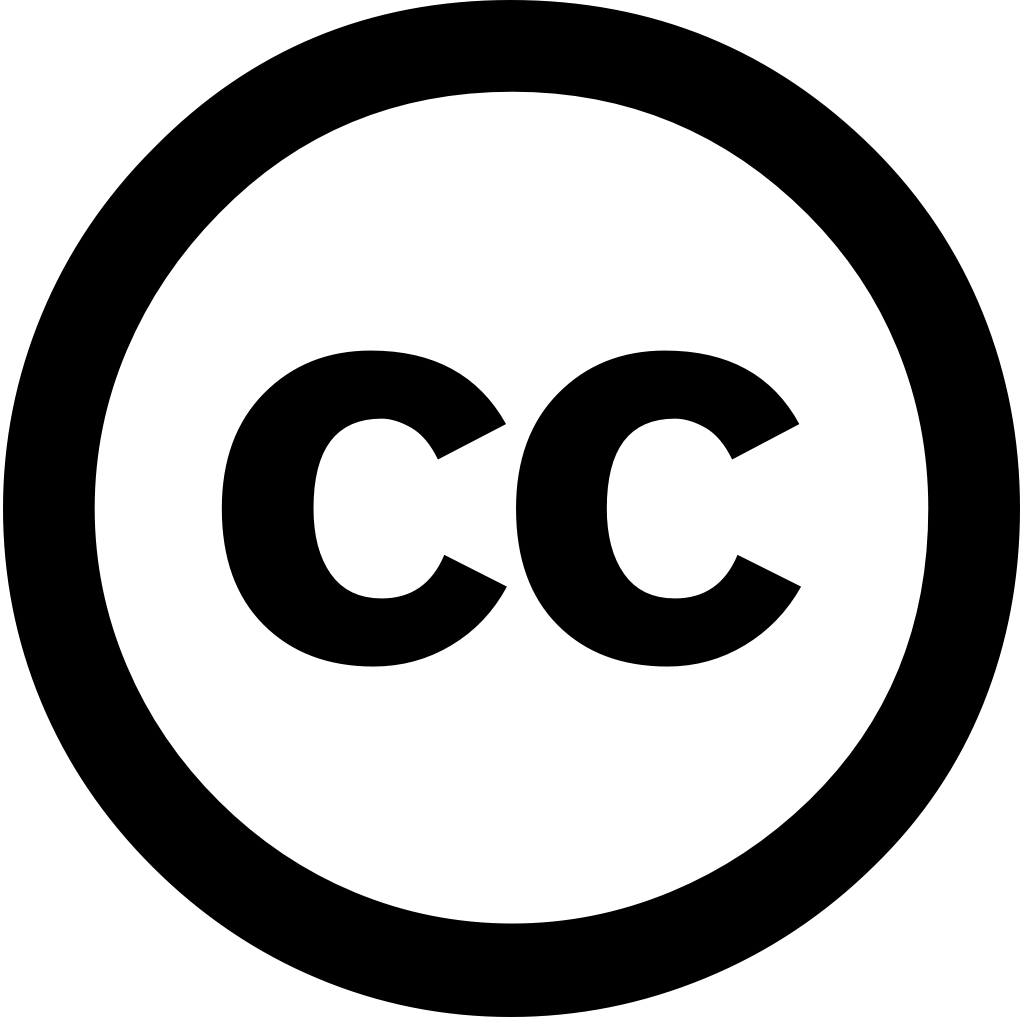
Research Square (Research Square), Год журнала: 2024, Номер unknown
Опубликована: Дек. 18, 2024
Язык: Английский
Research Square (Research Square), Год журнала: 2024, Номер unknown
Опубликована: Дек. 18, 2024
Язык: Английский
Heliyon, Год журнала: 2024, Номер 10(13), С. e33695 - e33695
Опубликована: Июнь 28, 2024
The water quality index (WQI) is a widely used tool for comprehensive assessment of river environments. However, its calculation involves numerous parameters, making sample collection and laboratory analysis time-consuming costly. This study aimed to identify key parameters the most reliable prediction models that could provide maximum accuracy using minimal indicators. Water from 2020 2023 were collected including nine biophysical chemical indicators in seventeen rivers Yancheng Nantong, two coastal cities Jiangsu Province, China, adjacent Yellow Sea. Linear regression seven machine learning (Artificial Neural Network (ANN), Self-Organizing Maps (SOM), K-Nearest Neighbor (KNN), Support Vector Machines (SVM), Random Forest (RF), Extreme Gradient Boosting (XGB) Stochastic (SGB)) developed predict WQI different groups input variables based on correlation analysis. results indicated improved 2022 but deteriorated 2023, with inland stations exhibiting better conditions than ones, particularly terms turbidity nutrients. environment was comparatively Nantong Yancheng, mean values approximately 55.3–72.0 56.4–67.3, respectively. classifications "Good" "Medium" accounted 80 % records, no instances "Excellent" 2 classified as "Bad". performance all models, except SOM, addition variables, achieving R2 higher 0.99 such SVM, RF, XGB, SGB. RF XGB total phosphorus (TP), ammonia nitrogen (AN), dissolved oxygen (DO) (R2 = 0.98 0.91 training testing phase) predicting values, TP AN (accuracy 85 %) grades. "Low" grades highest at 90 %, followed by level 70 %. model contribute efficient evaluation identifying facilitating effective management basins.
Язык: Английский
Процитировано
5Environmental Monitoring and Assessment, Год журнала: 2024, Номер 196(11)
Опубликована: Окт. 3, 2024
Язык: Английский
Процитировано
4Environmental Science and Pollution Research, Год журнала: 2025, Номер unknown
Опубликована: Янв. 20, 2025
Язык: Английский
Процитировано
0Journal of Contaminant Hydrology, Год журнала: 2025, Номер 270, С. 104518 - 104518
Опубликована: Фев. 16, 2025
Язык: Английский
Процитировано
0Ecological Indicators, Год журнала: 2025, Номер 172, С. 113299 - 113299
Опубликована: Март 1, 2025
Язык: Английский
Процитировано
0Remote Sensing, Год журнала: 2025, Номер 17(6), С. 988 - 988
Опубликована: Март 12, 2025
Groundwater systems are important for maintaining ecological balance and ensuring water supplies. However, under the combined pressures of shifting climate patterns human activities, their responses to extreme events have become increasingly complex. As China’s largest freshwater lake, Poyang Lake supports critical resources, health, adaptation efforts. Yet, relationship between groundwater storage (GWS) hydrological in this region remains insufficiently studied, hindering effective management. This study investigates GWS response by downscaling Gravity Recovery Climate Experiment (GRACE) data validating it with five years observed daily levels. Using GRACE, Global Land Data Assimilation System (GLDAS), ERA5 data, a convolutional neural network (CNN)–attention mechanism (A)–long short-term memory (LSTM) model was selected downscale high resolution (0.1° × 0.1°) estimate recovery times return baseline. Our analysis revealed seasonal fluctuations that phase precipitation, evapotranspiration, runoff. durations flood (2020) drought (2022) ranged from 0.8 3.1 months 0.2 4.8 months, respectively. A strong correlation meteorological droughts, while agricultural significantly weaker. These results indicate precipitation runoff more sensitive than evapotranspiration influencing changes. findings highlight significant sensitivity GWS, despite improved management
Язык: Английский
Процитировано
0Environmental Geochemistry and Health, Год журнала: 2025, Номер 47(5)
Опубликована: Апрель 5, 2025
Язык: Английский
Процитировано
0Applied Water Science, Год журнала: 2025, Номер 15(5)
Опубликована: Апрель 7, 2025
Язык: Английский
Процитировано
0Ecological Indicators, Год журнала: 2024, Номер 166, С. 112543 - 112543
Опубликована: Авг. 29, 2024
Язык: Английский
Процитировано
3Water, Год журнала: 2024, Номер 16(21), С. 3080 - 3080
Опубликована: Окт. 28, 2024
Water quality degradation and eutrophication of lakes are global ecological environmental concerns, especially shallow lakes. This study collected hydrochemical data from 2935 samples the Chinese part Xingkai (Khanka) Lake, based on 40 published papers spanning period 2001 to 2023. Using water index (WQI), improved geo-accumulation (Igeo), redundancy analysis (RDA), we analyzed overall contamination characteristics environment in Lake. Additionally, explored impact climate change human activities lake’s quality. The results showed that annual WQI for Lake ranged 47.3 72, with a general downward trend, indicating improving Notably, average May total nitrogen (TN) content decreased significantly, signaling further improvement concentration TN sediments was 1401.3 mg/kg, reflecting mild contamination. Igeo values heavy metals Hg Cr were greater than 1, moderate contamination, while Cd Pb between 0 which is range uncontaminated moderately contaminated. Land use (average temperature precipitation) key factors influencing quality, cumulative explanatory ratios 67.3% 50.1%. utilized land-use as metric activities, highlighting potential impacts It offers vital insights sustainable management provides valuable references into similar transboundary
Язык: Английский
Процитировано
3