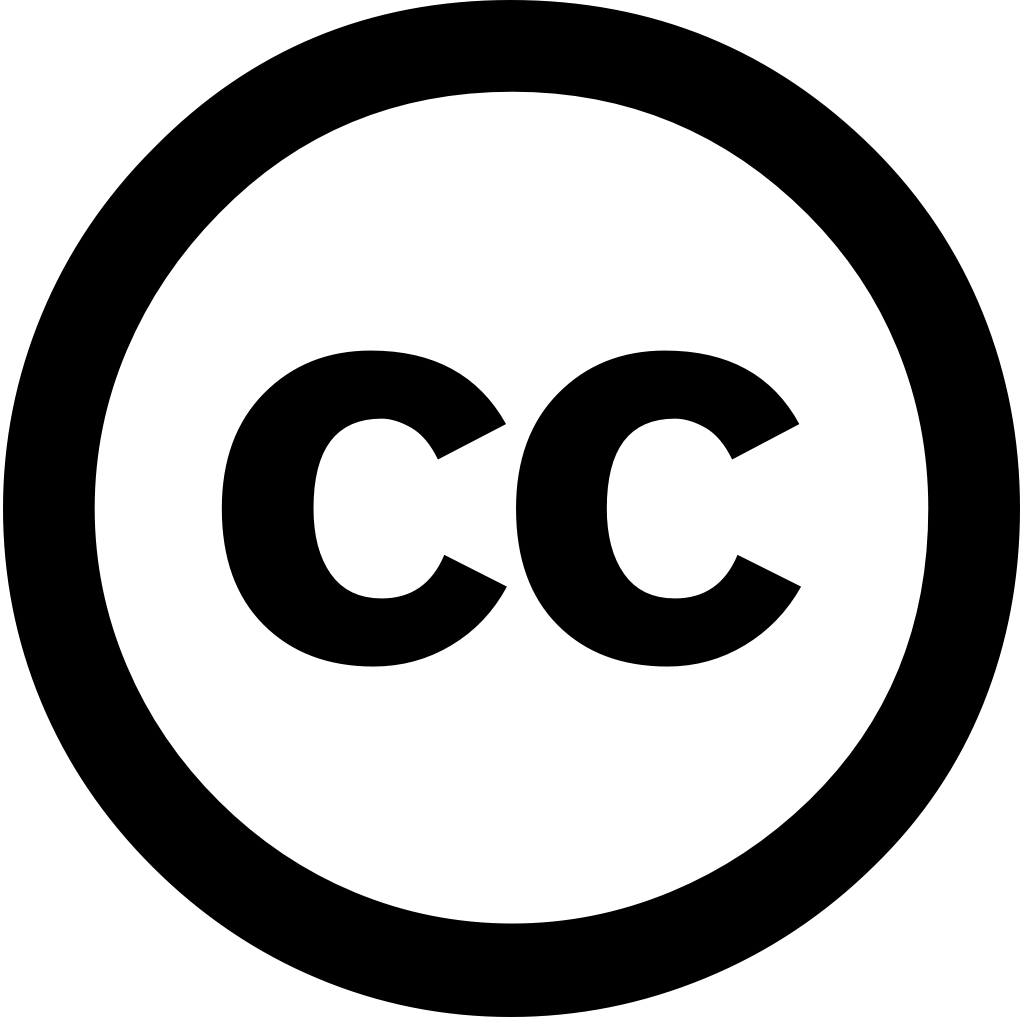
Computational and Structural Biotechnology Journal, Год журнала: 2024, Номер 23, С. 1886 - 1896
Опубликована: Апрель 25, 2024
Язык: Английский
Computational and Structural Biotechnology Journal, Год журнала: 2024, Номер 23, С. 1886 - 1896
Опубликована: Апрель 25, 2024
Язык: Английский
Nature Methods, Год журнала: 2024, Номер 21(8), С. 1481 - 1491
Опубликована: Июнь 6, 2024
Язык: Английский
Процитировано
81Nature Reviews Genetics, Год журнала: 2024, Номер 25(6), С. 381 - 400
Опубликована: Янв. 18, 2024
Язык: Английский
Процитировано
51Nucleic Acids Research, Год журнала: 2024, Номер 53(D1), С. D886 - D900
Опубликована: Ноя. 28, 2024
Hundreds of millions single cells have been analyzed using high-throughput transcriptomic methods. The cumulative knowledge within these datasets provides an exciting opportunity for unlocking insights into health and disease at the level cells. Meta-analyses that span diverse building on recent advances in large language models other machine-learning approaches pose new directions to model extract insight from single-cell data. Despite promise emerging analytical tools analyzing amounts data, sheer number datasets, data accessibility remains a challenge. Here, we present CZ CELLxGENE Discover (cellxgene.cziscience.com), platform curated interoperable Available via free-to-use online portal, hosts growing corpus community-contributed over 93 million unique Curated, standardized associated with consistent cell-level metadata, this collection is largest its kind rapidly community contributions. A suite features enables reusability both computational visual interfaces allow researchers explore individual perform cross-corpus analysis, run meta-analyses tens across studies tissues resolution
Язык: Английский
Процитировано
32Nature Reviews Molecular Cell Biology, Год журнала: 2024, Номер 26(1), С. 11 - 31
Опубликована: Авг. 21, 2024
Язык: Английский
Процитировано
31Nature Methods, Год журнала: 2024, Номер 21(8), С. 1430 - 1443
Опубликована: Авг. 1, 2024
Язык: Английский
Процитировано
27Cell, Год журнала: 2024, Номер 187(10), С. 2343 - 2358
Опубликована: Май 1, 2024
As the number of single-cell datasets continues to grow rapidly, workflows that map new data well-curated reference atlases offer enormous promise for biological community. In this perspective, we discuss key computational challenges and opportunities reference-mapping algorithms. We how mapping algorithms will enable integration diverse across disease states, molecular modalities, genetic perturbations, species eventually replace manual laborious unsupervised clustering pipelines.
Язык: Английский
Процитировано
25Biomolecules, Год журнала: 2024, Номер 14(3), С. 339 - 339
Опубликована: Март 12, 2024
Recent advancements in AI-driven technologies, particularly protein structure prediction, are significantly reshaping the landscape of drug discovery and development. This review focuses on question how these technological breakthroughs, exemplified by AlphaFold2, revolutionizing our understanding function changes underlying cancer improve approaches to counter them. By enhancing precision speed at which targets identified candidates can be designed optimized, technologies streamlining entire development process. We explore use AlphaFold2 development, scrutinizing its efficacy, limitations, potential challenges. also compare with other algorithms like ESMFold, explaining diverse methodologies employed this field practical effects differences for application specific algorithms. Additionally, we discuss broader applications including prediction complex structures generative design novel proteins.
Язык: Английский
Процитировано
24Cell, Год журнала: 2024, Номер 187(17), С. 4520 - 4545
Опубликована: Авг. 1, 2024
Язык: Английский
Процитировано
19IEEE Internet of Things Journal, Год журнала: 2024, Номер 11(21), С. 34749 - 34773
Опубликована: Июль 24, 2024
The Internet of Things (IoT) can significantly enhance the quality human life, specifically in healthcare, attracting extensive attentions to IoT healthcare services. Meanwhile, digital twin (HDT) is proposed as an innovative paradigm that comprehensively characterize replication individual body world and reflect its physical status real time. Naturally, HDT envisioned empower beyond application monitoring by acting a versatile vivid testbed, simulating outcomes guiding practical treatments. However, successfully establishing requires high-fidelity virtual modeling strong information interactions but possibly with scarce, biased, noisy data. Fortunately, recent popular technology called generative artificial intelligence (GAI) may be promising solution because it leverage advanced AI algorithms automatically create, manipulate, modify valuable while diverse This survey particularly focuses on implementation GAI-driven healthcare. We start introducing background potential HDT. Then, we delve into fundamental techniques present overall framework After that, explore realization detail, including GAI-enabled data acquisition, communication, management, modeling, analysis. Besides, discuss typical applications revolutionized HDT, namely, personalized health diagnosis, prescription, rehabilitation. Finally, conclude this highlighting some future research directions.
Язык: Английский
Процитировано
18Proceedings of the National Academy of Sciences, Год журнала: 2024, Номер 121(26)
Опубликована: Июнь 20, 2024
Proteomics has been revolutionized by large protein language models (PLMs), which learn unsupervised representations from corpora of sequences. These are typically fine-tuned in a supervised setting to adapt the model specific downstream tasks. However, computational and memory footprint fine-tuning (FT) PLMs presents barrier for many research groups with limited resources. Natural processing seen similar explosion size models, where these challenges have addressed methods parameter-efficient (PEFT). In this work, we introduce paradigm proteomics through leveraging method LoRA training new two important tasks: predicting protein–protein interactions (PPIs) symmetry homooligomer quaternary structures. We show that approaches competitive traditional FT while requiring reduced substantially fewer parameters. additionally PPI prediction task, only classification head also remains full FT, using five orders magnitude parameters, each outperform state-of-the-art compute. further perform comprehensive evaluation hyperparameter space, demonstrate PEFT is robust variations hyperparameters, elucidate best practices differ those natural processing. All our adaptation code available open-source at https://github.com/microsoft/peft_proteomics . Thus, provide blueprint democratize power PLM
Язык: Английский
Процитировано
16