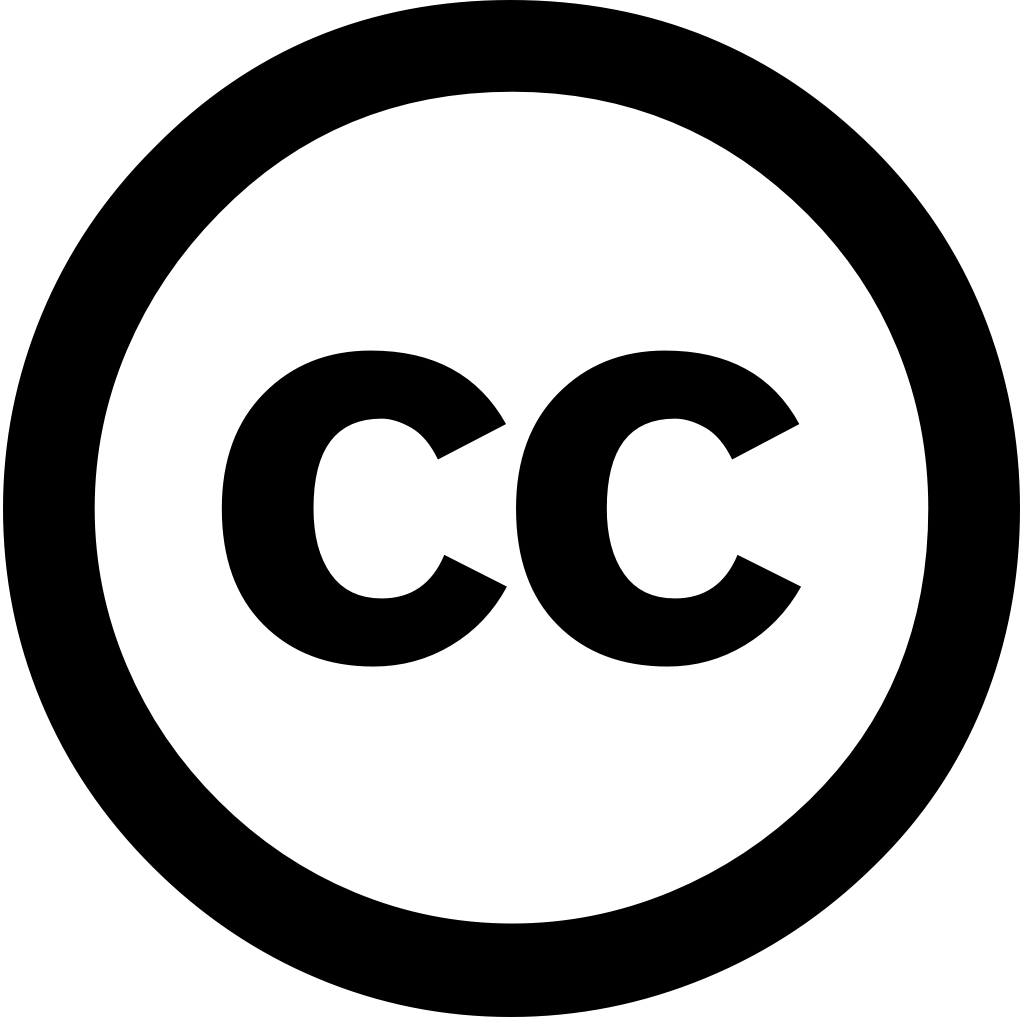
Smart Agricultural Technology, Год журнала: 2024, Номер unknown, С. 100677 - 100677
Опубликована: Ноя. 1, 2024
Язык: Английский
Smart Agricultural Technology, Год журнала: 2024, Номер unknown, С. 100677 - 100677
Опубликована: Ноя. 1, 2024
Язык: Английский
Comprehensive Reviews in Food Science and Food Safety, Год журнала: 2025, Номер 24(1)
Опубликована: Янв. 1, 2025
Abstract The food flavor science, traditionally reliant on experimental methods, is now entering a promising era with the help of artificial intelligence (AI). By integrating existing technologies AI, researchers can explore and develop new substances in digital environment, saving time resources. More more research will use AI big data to enhance product flavor, improve quality, meet consumer needs, drive industry toward smarter sustainable future. In this review, we elaborate mechanisms recognition their potential impact nutritional regulation. With increase accumulation development internet information technology, databases ingredient have made great progress. These provide detailed content, molecules, chemical properties various compounds, providing valuable support for rapid evaluation components construction screening technology. popularization fields, field has also ushered opportunities. This review explores role enhancing analysis through high‐throughput omics technologies. algorithms offer pathway scientifically formulations, thereby customized meals. Furthermore, it discusses safety challenges into industry.
Язык: Английский
Процитировано
3Computers and Electronics in Agriculture, Год журнала: 2024, Номер 222, С. 109032 - 109032
Опубликована: Май 29, 2024
Язык: Английский
Процитировано
13Computers and Electronics in Agriculture, Год журнала: 2024, Номер 221, С. 108924 - 108924
Опубликована: Апрель 16, 2024
Since the launch of "Generative Pre-trained Transformer 3.5", ChatGPT by Open, artificial intelligence (AI) has been a main discussion topic in public. Especially large language models (LLM), so called "intelligent" chatbots, and possibility to automatically generate highly professional technical texts get high attention. Companies, as well researchers, are evaluating possible applications how such powerful LLM can be integrated into daily work bring benefits, improve their business or make research outcome more efficient. In general, underlying trained on datasets, mainly sources from websites, online books articles. combination with information provided user, model give an impressively fast response. Even if range questions answers look unrestricted, there limits models. this paper, use cases for agricultural tasks elucidated. This includes textual preparation facts, consulting tasks, interpretation decision support plant disease management, guides tutorials integrate modern digital techniques work. Opportunities challenges described, limitations insufficiencies. The authors describe map easy-to-reach topics agriculture where integration LLMs seems very likely within next few years.
Язык: Английский
Процитировано
11Applied Sciences, Год журнала: 2025, Номер 15(2), С. 628 - 628
Опубликована: Янв. 10, 2025
In China, fruit tree diseases are a significant threat to the development of industry, and knowledge about is most needed professional for farmers other practitioners in industry. Research papers primary sources that represent cutting-edge progress disease research. Traditional engineering methods acquisition require extensive cumbersome preparatory work, they demand high level background information technology skills from handlers. This paper, perspective industry dissemination, aims at users such as farmers, experts, communicators, gatherers. It proposes fast, cost-effective, low-technical-barrier method extracting research paper abstracts—K-Extract, based on large language models (LLMs) prompt engineering. Under zero-shot conditions, K-Extract utilizes conversational LLMs automate extraction knowledge. The has constructed comprehensive classification system and, through series optimized questions, effectively overcomes deficiencies LLM providing factual accuracy. tests multiple available Chinese market, results show can seamlessly integrate with any model, DeepSeek model Kimi performing particularly well. experimental indicate have accuracy rate handling judgment tasks simple Q&A tasks. simple, efficient, accurate, serve convenient tool agricultural field.
Язык: Английский
Процитировано
1Smart Agricultural Technology, Год журнала: 2025, Номер unknown, С. 100824 - 100824
Опубликована: Фев. 1, 2025
Язык: Английский
Процитировано
1Environmental earth sciences, Год журнала: 2025, Номер unknown, С. 357 - 382
Опубликована: Янв. 1, 2025
Язык: Английский
Процитировано
1Heritage Science, Год журнала: 2024, Номер 12(1)
Опубликована: Апрель 9, 2024
Abstract Nanjing Yunjin, a traditional Chinese silk weaving craft, is celebrated globally for its unique local characteristics and exquisite workmanship, forming an integral part of the world's intangible cultural heritage. However, with advancement information technology, experiential knowledge Yunjin production process predominantly stored in text format. As highly specialized vertical domain, this not readily convert into usable data. Previous studies on graph-based Question-Answering System have partially addressed issue. graphs need to be constantly updated rely predefined entities relationship types. Faced ambiguous or complex natural language problems, graph retrieval faces some challenges. Therefore, study proposes that integrates Knowledge Graphs Retrieval Augmented Generation techniques. In system, ROBERTA model first utilized vectorize textual information, delving deep semantics unveil profound connotations. Additionally, FAISS vector database employed efficient storage achieving semantic match between questions answers. Ultimately, related results are fed Large Language Model enhanced generation, aiming more accurate generation outcomes improving interpretability logic System. This research merges technologies like embedding, vectorized retrieval, overcome limitations graphs-based terms updating, dependency types, understanding. implementation testing shown Intelligent System, constructed basis Generation, possesses broader base considers context, resolving issues polysemy, vague language, sentence ambiguity, efficiently accurately generates answers queries. significantly facilitates utilization knowledge, providing paradigm constructing other heritages, holds substantial theoretical practical significance exploration discovery structure human heritage, promoting inheritance protection.
Язык: Английский
Процитировано
6Agricultural Systems, Год журнала: 2024, Номер 220, С. 104099 - 104099
Опубликована: Авг. 29, 2024
Язык: Английский
Процитировано
6Frontiers in Artificial Intelligence, Год журнала: 2024, Номер 7
Опубликована: Окт. 25, 2024
Coverage of ChatGPT-style large language models (LLMs) in the media has focused on their eye-catching achievements, including solving advanced mathematical problems and reaching expert proficiency medical examinations. But gradual adoption LLMs agriculture, an industry which touches every human life, received much less public scrutiny. In this short perspective, we examine risks opportunities related to more widespread food production systems. While can potentially enhance agricultural efficiency, drive innovation, inform better policies, challenges like misinformation, collection vast amounts farmer data, threats jobs are important concerns. The rapid evolution LLM landscape underscores need for policymakers think carefully about frameworks guidelines that ensure responsible use before these technologies become so ingrained policy intervention becomes challenging.
Язык: Английский
Процитировано
6Scientific Reports, Год журнала: 2024, Номер 14(1)
Опубликована: Фев. 10, 2024
Abstract The limited number of agricultural extension agents (EAs) in sub-Saharan Africa limits farmers’ access to services. Artificial intelligence (AI) assistants could potentially aid providing answers questions. objective this study was evaluate the ability an AI chatbot assistant (ChatGPT) provide quality responses We compiled a list 32 questions related irrigated rice cultivation from farmers Kano State, Nigeria. Six EAs state were randomly selected answer these Their answers, along with those ChatGPT, assessed by four evaluators terms and local relevancy. Overall, rated significantly higher than EAs’ responses. Chatbot received best score nearly six times as often (40% vs. 7%). preferred 78% cases. topics for which poorer scores included planting time, seed rate, fertilizer application rate timing. In conclusion, while offer alternative source advisory services farmers, incorporating site-specific input rate-and-timing agronomic practices into is critical their direct use farmers.
Язык: Английский
Процитировано
5