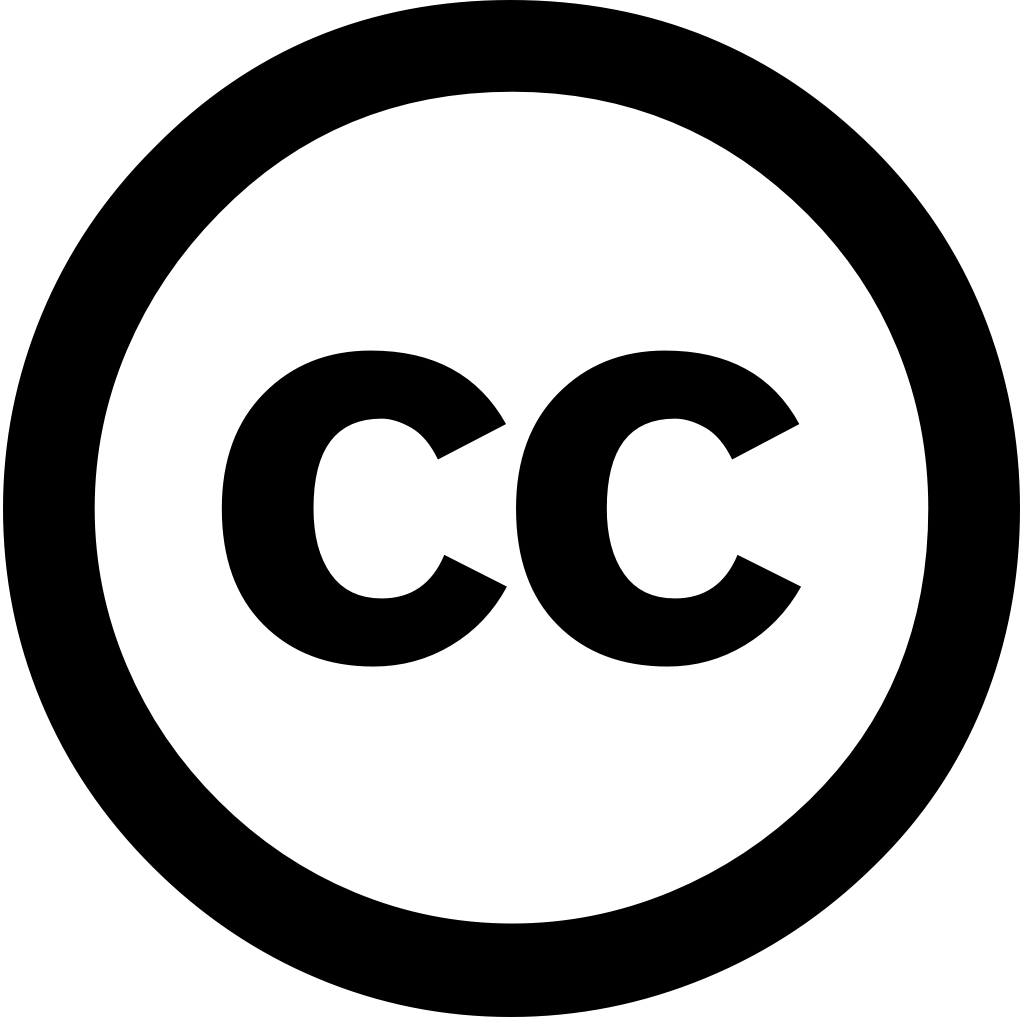
Alexandria Engineering Journal, Год журнала: 2024, Номер 115, С. 65 - 82
Опубликована: Дек. 12, 2024
Язык: Английский
Alexandria Engineering Journal, Год журнала: 2024, Номер 115, С. 65 - 82
Опубликована: Дек. 12, 2024
Язык: Английский
PLoS ONE, Год журнала: 2024, Номер 19(3), С. e0296352 - e0296352
Опубликована: Март 12, 2024
Chest disease refers to a wide range of conditions affecting the lungs, such as COVID-19, lung cancer (LC), consolidation (COL), and many more. When diagnosing chest disorders medical professionals may be thrown off by overlapping symptoms (such fever, cough, sore throat, etc.). Additionally, researchers make use X-rays (CXR), cough sounds, computed tomography (CT) scans diagnose disorders. The present study aims classify nine different disorders, including LC, COL, atelectasis (ATE), tuberculosis (TB), pneumothorax (PNEUTH), edema (EDE), pneumonia (PNEU). Thus, we suggested four novel convolutional neural network (CNN) models that train distinct image-level representations for classifications extracting features from images. Furthermore, proposed CNN employed several new approaches max-pooling layer, batch normalization layers (BANL), dropout, rank-based average pooling (RBAP), multiple-way data generation (MWDG). scalogram method is utilized transform sounds coughing into visual representation. Before beginning model has been developed, SMOTE approach used calibrate CXR CT well sound images (CSI) CXR, scan, CSI training evaluating come 24 publicly available benchmark illness datasets. classification performance compared with seven baseline models, namely Vgg-19, ResNet-101, ResNet-50, DenseNet-121, EfficientNetB0, DenseNet-201, Inception-V3, in addition state-of-the-art (SOTA) classifiers. effectiveness further demonstrated results ablation experiments. was successful achieving an accuracy 99.01%, making it superior both SOTA As result, capable offering significant support radiologists other professionals.
Язык: Английский
Процитировано
10Decision Analytics Journal, Год журнала: 2024, Номер 12, С. 100499 - 100499
Опубликована: Июль 2, 2024
Traditional deep learning models are often considered "black boxes" due to their lack of interpretability, which limits therapeutic use despite success in classification tasks. This study aims improve the interpretability diagnoses for COVID-19, pneumonia, and tuberculosis from X-ray images using an enhanced DenseNet201 model within a transfer framework. We incorporated Explainable Artificial Intelligence (XAI) techniques, including SHAP, LIME, Grad-CAM, Grad-CAM++, make model's decisions more understandable. To enhance image clarity detail, we applied preprocessing methods such as Denoising Autoencoder, Contrast Limited Adaptive Histogram Equalization (CLAHE), Gamma Correction. An ablation was conducted identify optimal parameters proposed approach. Our performance compared with other learning-based like EfficientNetB0, InceptionV3, LeNet evaluation metrics. The that included data augmentation techniques achieved best results, accuracy 99.20%, precision recall 99%. demonstrates critical role improving performance. SHAP LIME provided significant insights into decision-making process, while Grad-CAM Grad-CAM++ highlighted specific features regions influencing classifications. These transparency trust AI-assisted diagnoses. Finally, developed Android-based system most effective support medical specialists process.
Язык: Английский
Процитировано
9Biomedical Signal Processing and Control, Год журнала: 2024, Номер 100, С. 106959 - 106959
Опубликована: Сен. 30, 2024
Язык: Английский
Процитировано
8Journal of X-Ray Science and Technology, Год журнала: 2025, Номер unknown
Опубликована: Фев. 20, 2025
Background Lung disease is the crucial that affects breathing conditions and even causes death. There are various approaches for lung classification; still inefficiency in accurate detection, computational complexity over-fitting issues limits performance of model. To overcome challenges, a deep learning model proposed this research. Initially, input acquired pre-processed using three techniques like data augmentation, filtering image re-sizing. Then, threshold based segmentation employed obtaining required region. Objective From segmented image, categories diseases COVID, Opacity, Pneumonia normal identified Optimal Cross Stage Partial Bidirectional Long short term memory (OCBiNet). Methods The OCBiNet designed short-term (BiNet) with connection its hidden state. Besides, adjustable parameters modified Improved Mother Optimization (ImMO) algorithm. Results ImMO algorithm by integrating Logistic Chaotic Mapping within conventional enhancing convergence rate global best solution. Conclusions evaluated on Accuracy, Recall, Precision, F-Score values 99.11%, 98.98%, 99.18%, 99.08% respectively.
Язык: Английский
Процитировано
0Algorithms for intelligent systems, Год журнала: 2025, Номер unknown, С. 43 - 52
Опубликована: Янв. 1, 2025
Язык: Английский
Процитировано
0Medical Review, Год журнала: 2025, Номер unknown
Опубликована: Апрель 11, 2025
Abstract The integration of artificial intelligence (AI) in medical diagnostics represents a transformative advancement healthcare, with projected market growth reaching $188 billion by 2030. This comprehensive review examines the latest developments AI-driven diagnostic technologies across multiple disease domains, particularly focusing on cancer, Alzheimer’s (AD), and diabetes. Through systematic bibliometric analysis using GraphRAG methodology, we analyzed research publications from 2022 to 2024, revealing distribution impact AI applications various fields. In cancer diagnostics, systems have achieved breakthrough performances analyzing imaging molecular data, notable advances early detection capabilities 19 different types. For AD diagnosis, AI-powered tools demonstrated up 90 % accuracy risk through non-invasive methods, including speech pattern blood-based biomarkers. diabetes care, AI-integrated incorporating deep neural networks electronic nose technology shown remarkable predicting onset before clinical manifestation. These collectively indicate paradigm shift toward more precise, efficient, accessible approaches. However, challenges remain standardization, data quality, implementation. synthesizes current progress while highlighting potential for revolutionize enhanced accuracy, detection, personalized patient care.
Язык: Английский
Процитировано
0Algorithms, Год журнала: 2024, Номер 17(7), С. 302 - 302
Опубликована: Июль 8, 2024
A common and natural physiological response of the human body is cough, which tries to push air other wastage thoroughly from airways. Due environmental factors, allergic responses, pollution or some diseases, cough occurs. can be either dry wet depending on amount mucus produced. characteristic feature sound, a quacking sound mostly. Human sounds monitored continuously, so, classification has attracted lot interest in research community last decade. In this research, three systematic conglomerated models (SCMs) are proposed for audio signal classification. The first technique utilizes concept robust like Cross-Correlation Function (CCF) Partial (PCCF) model, Least Absolute Shrinkage Selection Operator (LASSO) elastic net regularization model with Gabor dictionary analysis efficient ensemble machine learning techniques, second stacked conditional autoencoders (SAEs) third using extraction schemes Tunable Q Wavelet Transform (TQWT), sparse TQWT, Maximal Information Coefficient (MIC), Distance Correlation (DCC) selection techniques Binary Tunicate Swarm Algorithm (BTSA), aggregation functions (AFs), factor (FA), explanatory (EFA) classified classifiers, kernel extreme (KELM), arc-cosine ELM, Rat Optimization (RSO)-based KELM, etc. utilized publicly available datasets, results show that highest accuracy 98.99% was obtained when TQWT AF implemented an ELM classifier.
Язык: Английский
Процитировано
1Big Data and Cognitive Computing, Год журнала: 2024, Номер 8(7), С. 77 - 77
Опубликована: Июль 9, 2024
Since early 2020, coronavirus has spread extensively throughout the globe. It was first detected in Wuhan, a province China. Many researchers have proposed various models to solve problems related COVID-19 detection. As traditional medical approaches take lot of time detect virus and require specific laboratory tests, adoption artificial intelligence (AI), including machine learning, might play an important role handling problem. A great deal research seen AI succeed detection using X-ray images. Unfortunately, majority deep learning for shortcomings high error computation costs. In this study, we employed hybrid model auto-encoder (AE) convolutional neural network (CNN) (named AMIKOMNET) with small number layers parameters. We implemented ensemble mechanism AMIKOMNET Adaboost aim reducing classification tasks. The experimental results binary class show that our achieved effectiveness, 96.90% accuracy, 95.06% recall, 94.67% F1-score, 96.03% precision. result multiclass 95.13% 94.93% 95.75% 96.19% increased effectiveness 98.45% 96.16% 95.70% 96.87% task also saw increase performance, accuracy 96.65%, recall 94.93%, F1-score 95.76%, precision 96.19%. implementation AE handle image feature extraction combined CNN used dimensional reduction outstanding performance when compared previous work platform. Exploiting detecting COVID-19.
Язык: Английский
Процитировано
0Journal of Clinical Medicine, Год журнала: 2024, Номер 13(22), С. 6913 - 6913
Опубликована: Ноя. 16, 2024
Chronic respiratory diseases (CRDs), including asthma and chronic obstructive pulmonary disease (COPD), represent significant global health challenges, contributing to substantial morbidity mortality. As the prevalence of CRDs continues rise, particularly in low-income countries, there is a pressing need for more efficient personalized approaches diagnosis treatment. This article explores impact emerging technologies, artificial intelligence (AI), on management CRDs. AI applications, machine learning (ML), deep (DL), large language models (LLMs), are transforming landscape CRD care, enabling earlier diagnosis, treatment, enhanced remote patient monitoring. The integration with telehealth wearable technologies further supports proactive interventions improved outcomes. However, challenges remain, issues related data quality, algorithmic bias, ethical concerns such as privacy transparency. paper evaluates effectiveness, accessibility, implications AI-driven tools management, offering insights into their potential shape future healthcare. advanced managing like COPD holds enhancing early monitoring, though remain regarding considerations, regulatory oversight.
Язык: Английский
Процитировано
0Alexandria Engineering Journal, Год журнала: 2024, Номер 115, С. 65 - 82
Опубликована: Дек. 12, 2024
Язык: Английский
Процитировано
0