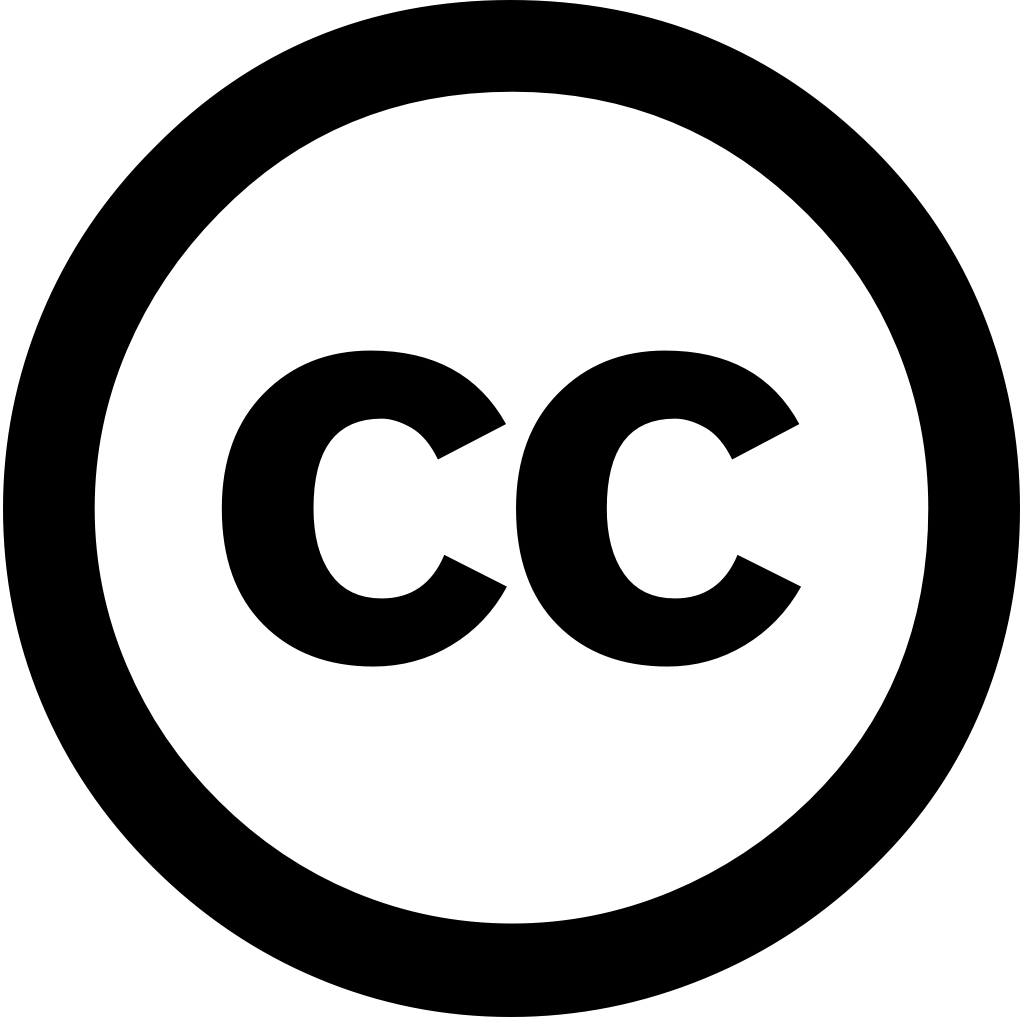
Heliyon, Год журнала: 2024, Номер 11(1), С. e41262 - e41262
Опубликована: Дек. 24, 2024
Язык: Английский
Heliyon, Год журнала: 2024, Номер 11(1), С. e41262 - e41262
Опубликована: Дек. 24, 2024
Язык: Английский
Buildings, Год журнала: 2024, Номер 14(3), С. 591 - 591
Опубликована: Фев. 22, 2024
Landscape geopolymer concrete (GePoCo) with environmentally friendly production methods not only has a stable structure but can also effectively reduce environmental damage. Nevertheless, GePoCo poses challenges its intricate cementitious matrix and vague mix design, where the components their relative amounts influence compressive strength. In response to these challenges, application of accurate applicable soft computing techniques becomes imperative for predicting strength such composite matrix. This research aimed predict using waste resources through novel ensemble ML algorithm. The dataset comprised 156 statistical samples, 15 variables were selected prediction. model employed combination RF, GWO algorithm, XGBoost. A stacking strategy was implemented by developing multiple RF models different hyperparameters, combining outcome predictions into new dataset, subsequently XGBoost model, termed RF–XGBoost model. To enhance accuracy errors, algorithm optimized hyperparameters resulting in RF–GWO–XGBoost proposed compared stand-alone models, hybrid GWO–XGBoost system. results demonstrated significant performance improvement strategies, particularly assistance exhibited better effectiveness, an RMSE 1.712 3.485, R2 0.983 0.981. contrast, (RF XGBoost) lower performance.
Язык: Английский
Процитировано
24Buildings, Год журнала: 2024, Номер 14(3), С. 615 - 615
Опубликована: Фев. 26, 2024
The design of geopolymer concrete must meet more stringent requirements for the landscape, so understanding and designing with a higher compressive strength challenging. In performance prediction strength, machine learning models have advantage being accurate faster. However, only single model is usually used at present, there are few applications ensemble models, optimization processes lacking. Therefore, this paper proposes to use Firefly Algorithm (AF) as an tool perform hyperparameter tuning on Logistic Regression (LR), Multiple (MLR), decision tree (DT), Random Forest (RF) models. At same time, reliability efficiency four integrated were analyzed. was analyze influencing factors determine their ability. According experimental data, RF-AF had lowest RMSE value. value training set test 4.0364 8.7202, respectively. R 0.9774 0.8915, compared other three has stronger generalization ability accuracy. addition, molar concentration NaOH most important factors, its influence far greater than possible including content. it necessary pay attention molarity when concrete.
Язык: Английский
Процитировано
13REVIEWS ON ADVANCED MATERIALS SCIENCE, Год журнала: 2024, Номер 63(1)
Опубликована: Янв. 1, 2024
Abstract Using supplementary cementitious materials in concrete production makes it eco-friendly by decreasing cement usage and the corresponding CO 2 emissions. One key measure of concrete’s durability performance is its porosity. An empirical prediction porosity high-performance with added elements goal this work, which employs machine learning approaches. Binder, water/cement ratio, slag, aggregate content, superplasticizer (SP), fly ash, curing conditions were considered as inputs database. The aim study to create ML models that could evaluate Gene expression programming (GEP) multi-expression (MEP) used develop these models. Statistical tests, Taylor’s diagram, R values, difference between experimental predicted readings metrics With = 0.971, mean absolute error (MAE) 0.348%, root square (RMSE) 0.460%, Nash–Sutcliffe efficiency (NSE) MEP provided a slightly better-fitted model improved when contrasted GEP, had 0.925, MAE 0.591%, RMSE 0.745%, NSE 0.923. water/binder conditions, content direct (positive) relationship concrete, while SP, slag an indirect (negative) association, according SHapley Additive exPlanations study.
Язык: Английский
Процитировано
7Journal of Composites Science, Год журнала: 2024, Номер 8(8), С. 287 - 287
Опубликована: Июль 26, 2024
High-Performance Concrete (HPC) and Ultra-High-Performance (UHPC) have many applications in civil engineering industries. These two types of concrete as similarities they differences with each other, such the mix design additive powders like silica fume, metakaolin, various fibers, however, optimal percentages mixture properties element these concretes are completely different. This study investigated between to find better mechanical behavior through parameters concrete. In addition, this paper studied correlation matrix machine learning method predict relationship elements properties. way, Linear, Ridge, Lasso, Random Forest, K-Nearest Neighbors (KNN), Decision tree, Partial least squares (PLS) regressions been chosen best regression types. To accuracy, coefficient determination (R2), mean absolute error (MAE), root-mean-square (RMSE) were selected. Finally, PLS, Lasso had results than other regressions, R2 greater 93%, 92%, respectively. general, present shows that HPC UHPC different designs for predicting
Язык: Английский
Процитировано
7Buildings, Год журнала: 2024, Номер 14(4), С. 1173 - 1173
Опубликована: Апрель 21, 2024
Permeable concrete is a type of porous with the special function water permeability, but permeability permeable will decrease gradually due to clogging behavior arising from surrounding environment. To reliably characterize concrete, particle swarm optimization (PSO) and random forest (RF) hybrid artificial intelligence techniques were developed in this study predict coefficient optimize aggregate mix ratio concrete. Firstly, reliable database was collected established input output variables for machine learning. Then, PSO 10-fold cross-validation used hyperparameters RF model using training testing datasets. Finally, accuracy verified by comparing predicted value actual coefficients (R = 0.978 RMSE 1.3638 dataset; R 0.9734 2.3246 dataset). The proposed can provide predictions that may face trend its development.
Язык: Английский
Процитировано
6REVIEWS ON ADVANCED MATERIALS SCIENCE, Год журнала: 2024, Номер 63(1)
Опубликована: Янв. 1, 2024
Abstract Marble cement (MC) is a new binding material for concrete, and the strength assessment of resulting materials subject this investigation. MC was tested in combination with rice husk ash (RHA) fly (FA) to uncover its full potential. Machine learning (ML) algorithms can help formulation better MC-based concrete. ML models that could predict compressive (CS) concrete contained FA RHA were built. Gene expression programming (GEP) multi-expression (MEP) used build these models. Additionally, evaluated by calculating R 2 values, carrying out statistical tests, creating Taylor’s diagram, comparing theoretical experimental readings. When MEP GEP models, yielded slightly better-fitted model prediction performance ( = 0.96, mean absolute error 0.646, root square 0.900, Nash–Sutcliffe efficiency 0.960). According sensitivity analysis, CS most affected curing age content, then contents. Incorporating waste such as marble powder, RHA, into building reduce environmental impacts encourage sustainable development.
Язык: Английский
Процитировано
6International Journal of Medical Informatics, Год журнала: 2025, Номер 196, С. 105793 - 105793
Опубликована: Янв. 21, 2025
Язык: Английский
Процитировано
0Proceedings of the Institution of Mechanical Engineers Part E Journal of Process Mechanical Engineering, Год журнала: 2025, Номер unknown
Опубликована: Фев. 26, 2025
This experimental study examines how cutting parameters influence surface roughness (Ra), kerf width (KW), heat-affected zone (HAZ), and material removal rate (MRR) during CO 2 laser of polyethylene (PE) material. The aim is to determine the optimal conditions by integrating fuzzy analytic hierarchy process (AHP) multi-criteria decision-making (MCDM) methods. Control factors (i.e. focal length, power, speed) were varied in a full factorial design. Criterion weights for responses calculated with help AHP method environment, resulting 0.442 Ra, 0.102 KW, 0.287 HAZ, 0.169 MRR. These integrated into MCDM methods combined compromise solution [CoCoSo], technique order preference similarity ideal [TOPSIS], Vlsekriterijumska Optimizacija I Kompromisno Resenje [VIKOR]) rank experiments identify parameters. condition was found be length 8 mm, power 90 W, speed 12 mm/s. Spearman's correlation coefficients applied analysis consistency among hybrid approaches, results indicating very strong positive relationship between them. comprehensive approach ensures precise efficient PE, contributing valuable insights field manufacturing materials processing.
Язык: Английский
Процитировано
0Symmetry, Год журнала: 2025, Номер 17(3), С. 443 - 443
Опубликована: Март 16, 2025
Modern digital media requires digitalization to protect cultural traditions, languages, and artistic expressions meant for future generations. Implementing the best strategy remains difficult because of unpredictable technological advances, changing preservation standards, financial constraints. This study deals with these intricate challenges through establishment picture fuzzy combined compromise ideal solution (PF-COCOFISO) decision-making approach. The proposed framework employs sets (PFSs) develop symmetrical assessment tools that better manage systems operating in uncertain settings. practical research analyzes heritage archive optimization by assessing various approaches regarding important criteria, including adaptability levels accessibility, maintenance, security systems, sustainability initiatives. Multiple conflicting criteria can be optimally managed PF-COCOFISO selection process, which improves reliability. establishes an operational method allows organizations archivists policymakers achieve intangible accessibility symmetry while preserving structured methods during unstable times.
Язык: Английский
Процитировано
0Frontiers in Built Environment, Год журнала: 2025, Номер 11
Опубликована: Апрель 8, 2025
A novel form of high-tech concrete known basalt fiber-reinforced high-performance (BFHPC) has been developed using traditional materials that require extra admixtures to improve its mechanical properties. Machine learning (ML) techniques provide a more flexible and economical way predict the property chopped minibar based on material properties processing parameters, enabling durable environmentally friendly construction. Predicting BFHPC precisely is crucial since it reduces design costs time, also minimizes waste from several mixing experiments. In this study, compressive strength flexural are predicted via different types machine models. Experiments carried out in laboratory under standard controlled settings at 7, 14, 28-day curing periods yielded sample data for analysis model development. The characteristics have combination decision tree, partial least squares, lasso, rigid, random forest regressor, K Neighbours, linear regressions. According results, all regression best results except KNeighbors Regressor, Random Forest Lasso Regression, with correlation coefficient R 2 93%. Each model’s performance application examined thoroughly, leading identification useful suggestions, existing knowledge gaps, areas need further study.
Язык: Английский
Процитировано
0