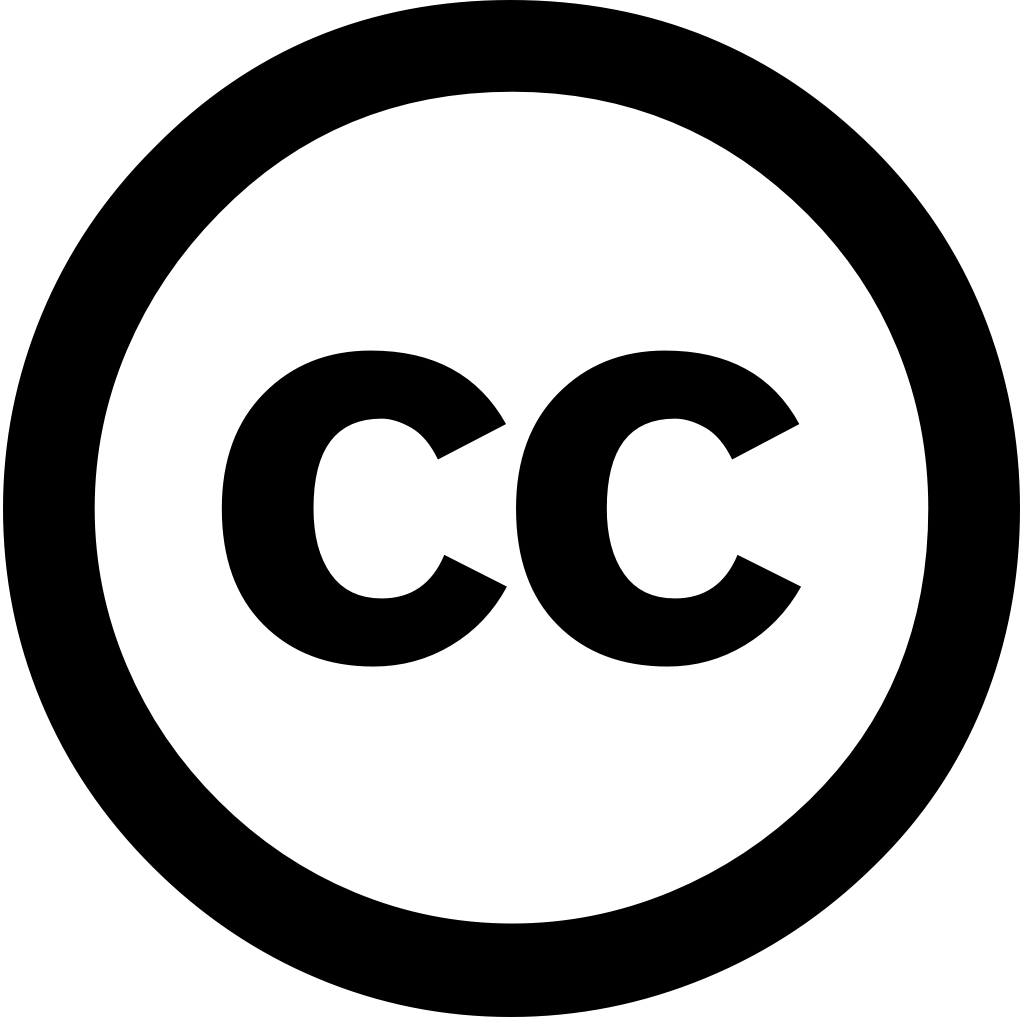
Heart Lung and Circulation, Год журнала: 2023, Номер 32(10), С. 1178 - 1188
Опубликована: Сен. 22, 2023
Язык: Английский
Heart Lung and Circulation, Год журнала: 2023, Номер 32(10), С. 1178 - 1188
Опубликована: Сен. 22, 2023
Язык: Английский
Sensors, Год журнала: 2022, Номер 22(19), С. 7227 - 7227
Опубликована: Сен. 23, 2022
Coronary heart disease is one of the major causes deaths around globe. Predicating a most challenging tasks in field clinical data analysis. Machine learning (ML) useful diagnostic assistance terms decision making and prediction on basis produced by healthcare sector globally. We have also perceived ML techniques employed medical prediction. In this regard, numerous research studies been shown using an classifier. paper, we used eleven classifiers to identify key features, which improved predictability disease. To introduce model, various feature combinations well-known classification algorithms were used. achieved 95% accuracy with gradient boosted trees multilayer perceptron model. The Random Forest gives better performance level prediction, 96%.
Язык: Английский
Процитировано
63European Heart Journal - Digital Health, Год журнала: 2022, Номер 3(2), С. 323 - 337
Опубликована: Май 2, 2022
Abstract Aims High blood pressure (BP) is the commonest modifiable cardiovascular risk factor, yet its monitoring remains problematic. Wearable cuffless BP devices offer potential solutions; however, little known about their validity and utility. We aimed to systematically review validity, features clinical use of wearable devices. Methods results searched MEDLINE, Embase, IEEE Xplore Cochrane Database till December 2019 for studies that reported validating extracted information study characteristics, device features, validation processes, applications. Devices were classified according functions features. defined with a mean systolic (SBP) diastolic (DBP) biases <5 mmHg as valid consensus. Our definition did not include assessment measurement precision, which assessed by standard deviation difference—a critical component ISO protocol criteria. Study quality was using Quality Assessment Diagnostic Accuracy Studies version 2 tool. A random-effects model meta-analysis performed summarise SBP DBP across studies. Of 430 identified, 16 (15 devices, 974 participants) selected. The majority (81.3%) used photoplethysmography estimate against reference device; other technologies included tonometry, auscultation electrocardiogram. In addition heart rate, some also measured night-time (n = 5), sleep 3), oxygen saturation temperature 2) electrocardiogram 3). Eight showed compared three commercially available. no statistically significant differences between (pooled difference 3.42 mmHg, 95% CI: −2.17, 9.01, I2 95.4%) 1.16 −1.26, 3.58, 87.1%). Conclusion Several are currently available different technologies, offering continuous monitoring. variation in standards protocols limited comparability findings identification most accurate device. Challenges such real-life settings must be overcome before they can recommended uptake into practice.
Язык: Английский
Процитировано
40International Journal of Medical Informatics, Год журнала: 2023, Номер 175, С. 105088 - 105088
Опубликована: Май 3, 2023
Disease comorbidity is a major challenge in healthcare affecting the patient's quality of life and costs. AI-based prediction comorbidities can overcome this issue by improving precision medicine providing holistic care. The objective systematic literature review was to identify summarise existing machine learning (ML) methods for evaluate interpretability explainability models.The Preferred Reporting Items Systematic Reviews Meta-Analyses (PRISMA) framework used articles three databases: Ovid Medline, Web Science PubMed. search covered broad range terms disease ML, including traditional predictive modelling.Of 829 unique articles, 58 full-text papers were assessed eligibility. A final set 22 with 61 ML models included review. Of identified models, 33 achieved relatively high accuracy (80-95%) AUC (0.80-0.89). Overall, 72% studies had or unclear concerns regarding risk bias.This first examine use explainable artificial intelligence (XAI) prediction. chosen focused on limited scope ranging from 1 34 (mean = 6), no novel found due phenotypic genetic data. lack standard evaluation XAI hinders fair comparisons.A has been predict various disorders. With further development capacity field prediction, there significant possibility identifying unmet health needs highlighting patient groups that not previously recognised be at particular comorbidities.
Язык: Английский
Процитировано
32Scientific Reports, Год журнала: 2023, Номер 13(1)
Опубликована: Авг. 21, 2023
Abstract Heart disease is a significant global cause of mortality, and predicting it through clinical data analysis poses challenges. Machine learning (ML) has emerged as valuable tool for diagnosing heart by analyzing healthcare data. Previous studies have extensively employed ML techniques in medical research prediction. In this study, eight classifiers were utilized to identify crucial features that enhance the accuracy Various combinations well-known classification algorithms develop prediction model. Neural network models, such Naïve Bayes Radial Basis Functions, implemented, achieving accuracies 94.78% 90.78% respectively Among state-of-the-art methods cardiovascular problem prediction, Learning Vector Quantization exhibited highest rate 98.7%. The motivation behind Cardiovascular Disease lies its potential save lives, improves health outcomes, allocates resources efficiently. key contributions encompass early intervention, personalized medicine, technological advancements, impact on public health, ongoing research, all which collectively work toward reducing burden CHD both individual patients society whole.
Язык: Английский
Процитировано
31Pharmaceuticals, Год журнала: 2024, Номер 17(6), С. 757 - 757
Опубликована: Июнь 8, 2024
Diabetes, a multifactorial metabolic disorder, demands the discovery of multi-targeting drugs with minimal side effects. This study investigated antidiabetic potential quercetin and kaempferol. The druggability binding affinities both compounds towards multiple targets were explored using pharmacokinetic docking software (AutoDock Vina 1.1.2). Our findings showed that kaempferol obey Lipinski’s rule five exhibit desirable ADMET (absorption, distribution, metabolism excretion, toxicity) profiles. Both higher C-reactive protein (CRP), interleukin-1 (IL-1), dipeptidyl peptidase-4 (DPP-IV), peroxisome proliferator-activated receptor gamma (PPARG), tyrosine phosphatase (PTP), sodium–glucose co-transporter-1 (SGLT-1) compared to metformin (the positive control). inhibited α-amylase activity (in vitro) up 20.30 ± 0.49 37.43 0.42%, respectively. Their oral supplementation significantly reduced blood glucose levels (p < 0.001), improved lipid profile enhanced total antioxidant status 0.01) in streptozotocin–nicotinamide (STZ-NA)-induced diabetic mice. Additionally, proliferation Huh-7 HepG2 (cancer cells) 0.0001) no effect on viability Vero cell line (non-cancer). In conclusion, demonstrated than metformin. vitro vivo along anticancer activities suggest promise for further development diabetes management. combination did not show synergistic effect, possibly due their same target receptors.
Язык: Английский
Процитировано
9International Research Journal on Advanced Science Hub, Год журнала: 2022, Номер 5(01), С. 15 - 21
Опубликована: Дек. 28, 2022
Heart disease is one of the leading killers that are widely recognized through- out globe. Large volumes clinical data stored in a variety sys- tems and biological equipment at hospitals. It essential to grasp facts heart order improve forecast accuracy. In this paper, experimental evaluations have been conducted assess effectiveness models created utilizing classification algorithms relevant attributes selected using Extra Tree feature selection procedures. Several people suffer originated globally. necessary use mining machine learning techniques extract new insights data. Analyzing medical sets diagnostic issues, including disease, involved number categorization approaches. However, these methods were only per- formed on small, balanced data; then, features must be derived trial error. Additionally, several sectors made substantial enhance performance. This paper aims propose comprehensive approach prediction such as Bagging, Support Vec- tor Machine, Multilayer Perception Gradient Boost with extra tree. The results showed improvements prediction. Bagging received scores training model 80% sample 99.08, 73.19, 67.20, 69.20 80.66 accuracy, precision, recall, F1-score roc respectively. experiment, we tested 20% sam- ple for each classifier find perform higher score 92.62, 48.44, 39.63, 41.89, 66.82
Язык: Английский
Процитировано
29Nutrients, Год журнала: 2023, Номер 15(18), С. 3937 - 3937
Опубликована: Сен. 11, 2023
Cardiovascular disease (CVD) is one of the primary causes death around world. This study aimed to identify risk factors associated with CVD mortality using data from National Health and Nutrition Examination Survey (NHANES). We created three models focusing on dietary data, non-diet-related health a combination both. Machine learning (ML) models, particularly random forest algorithm, demonstrated robust consistency across health, nutrition, mixed categories in predicting CVD. Shapley additive explanation (SHAP) values showed age, systolic blood pressure, several other as crucial variables, while fiber, calcium, vitamin E, among others, were significant nutritional variables. Our research emphasizes importance comprehensive evaluation intake mortality. The inclusion nutrition variables improved performance our underscoring utility ML-based analysis. Further investigation large datasets recurring recalls necessary enhance effectiveness interpretability such models.
Язык: Английский
Процитировано
19JMIR Diabetes, Год журнала: 2022, Номер 7(2), С. e33264 - e33264
Опубликована: Апрель 8, 2022
Diabetes is one of the leading noncommunicable chronic diseases globally. In people with diabetes, blood glucose levels need to be monitored regularly and managed adequately through healthy lifestyles medications. However, various factors contribute poor medication adherence. Smartphone apps can improve adherence in but it not clear which app features are most beneficial.This study aims systematically review evaluate high-quality for diabetes adherence, freely available public Android Apple stores present technical apps.We searched App Store Google Play that assist using predefined selection criteria. We assessed Mobile Rating Scale (MARS) calculated mean app-specific score (MASS) by taking average scores on 6 dimensions, namely, awareness, knowledge, attitudes, intention change, help-seeking, behavior change rated a 5-point scale (1=strongly disagree 5=strongly agree). used app's performance these dimensions calculate MASS. Apps achieved total MASS quality greater than 4 out 5 were considered high our study. formulated task-technology fit matrix adherence.We identified 8 (MASS score≥4) presented findings under 3 main categories: characteristics included apps, features, Our framework smartphone promoting physiological influencing features. On evaluation, we observed 25% promoted another moderate Finally, found 50% provided low medication.Our show almost half publicly free did achieve could have positive implications future design development patients diabetes. Additionally, evaluated standardized framework, only those higher should prescribed better health outcomes.
Язык: Английский
Процитировано
27IEEE Access, Год журнала: 2023, Номер 11, С. 60254 - 60266
Опубликована: Янв. 1, 2023
In medical informatics, deep learning-based models are being used to predict and diagnose cardiovascular diseases (CVDs). These can detect clinical signs, recognize phenotypes, pick treatment methods for complicated illnesses. One approach predicting CVDs is collect a large dataset of patient records use it train learning model. This study investigated early prediction using regression analysis on 2621 from UAE hospitals, including age, symptoms, CVD information. We propose long short-term memory-based neural network by leveraging the analysis. It be seen that accuracy level increased when they were simulated in pairs one disease with another due overlapping symptoms. The study's results suggest coronary heart has been predicted an 71.5% level, 84.4% Dyspnea; measured combination three conditions was 86.7%, Dyspnea, Chest Pain, Cyanosis, up 88.9%. Weakness, Fatigue, Emptysis showed value 89.8%. our proposed work, combinations Weakness Emptysis, discomfort pressure chest have shown ideal 90.6%, Fever, 91%. show effectiveness method several evaluation benchmarks.
Язык: Английский
Процитировано
15IEEE Access, Год журнала: 2023, Номер 11, С. 136758 - 136769
Опубликована: Янв. 1, 2023
Detecting cardiovascular irregularities in a timely manner is crucial for preventing any fatal risks. This research aims to devise an efficient forecasting algorithm the prognosis of Coronary Heart Disease (CHD). The study includes diverse sample individuals from Framingham, Massachusetts, with varying demographic, clinical, and co-morbidity parameters. We aim achieve this two-step ensemble Machine Learning model. Firstly, feature importance integrated conventional classifiers build Feature Weighted Meta-Models Forward selection algorithm. Subsequently, top-performing are combined design Hybrid Voting Models predict risk CHD ten-year timeframe by minimizing misclassification rate. proposed models undergo vetting using multiple metrics, including F1 score, Matthew's Correlation Coefficient (MCC), Misclassification Ratio (MCR), Accuracy. Given high cost associated healthcare domain, these metrics carefully considered. resulting model demonstrated strong predictive capability risk, achieving overall accuracy rate 95.87%. score calculated be 0.91, MCC 0.83, MCR 0.041. Notably, achieved impressive results only seven features, reducing time complexity prediction. In comparison classifiers, our 23.94% improvement accuracy, 17.23% over average Meta-models highlighting its effectiveness predicting risk.
Язык: Английский
Процитировано
14