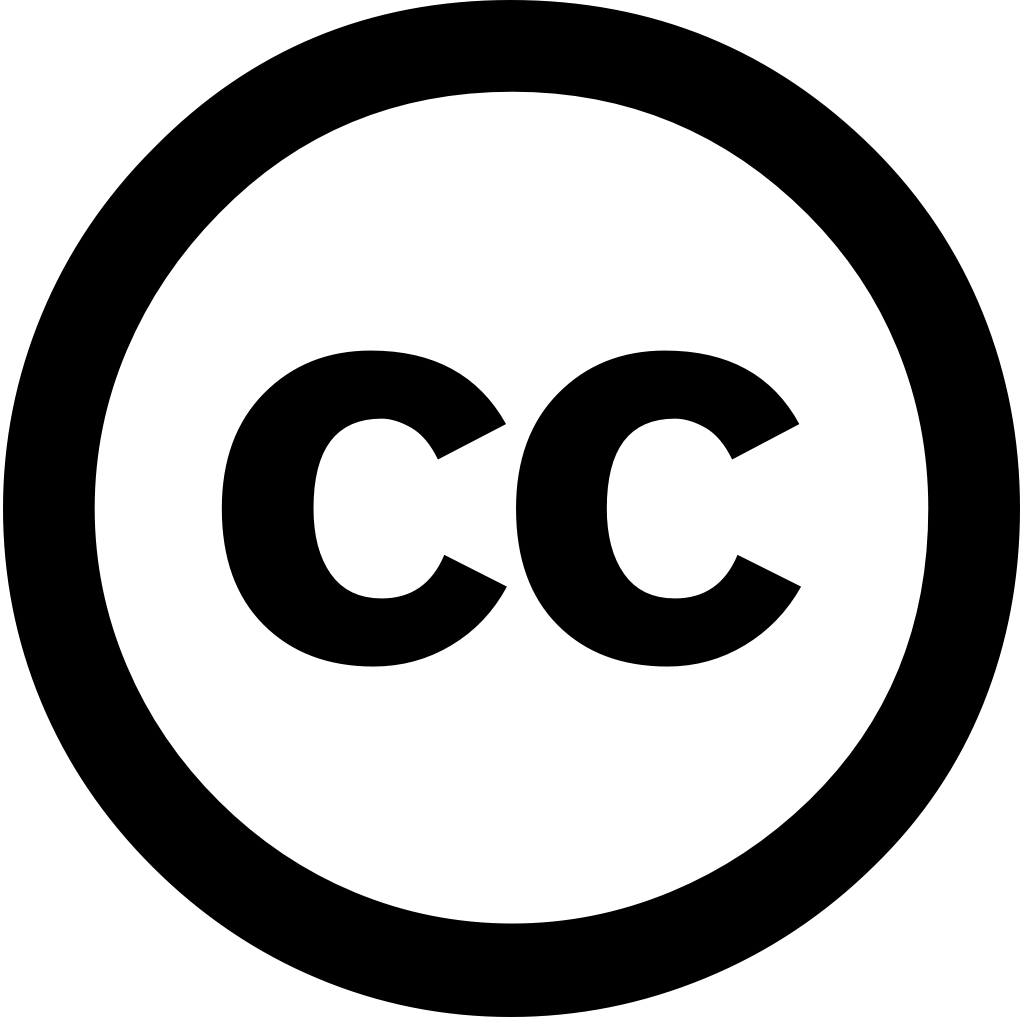
Materials Science and Engineering R Reports, Год журнала: 2020, Номер 144, С. 100595 - 100595
Опубликована: Дек. 26, 2020
Язык: Английский
Materials Science and Engineering R Reports, Год журнала: 2020, Номер 144, С. 100595 - 100595
Опубликована: Дек. 26, 2020
Язык: Английский
Journal of Medicinal Chemistry, Год журнала: 2020, Номер 63(16), С. 8683 - 8694
Опубликована: Июль 16, 2020
The data sets available to train models for in silico drug discovery efforts are often small. Indeed, the sparse availability of labeled is a major barrier artificial-intelligence-assisted discovery. One solution this problem develop algorithms that can cope with relatively heterogeneous and scarce data. Transfer learning type machine leverage existing, generalizable knowledge from other related tasks enable separate task small set Deep transfer most commonly used field This Perspective provides an overview applications date. Furthermore, it outlooks on future development
Язык: Английский
Процитировано
279Chemical Science, Год журнала: 2020, Номер 11(10), С. 2587 - 2605
Опубликована: Янв. 1, 2020
Enzymes are excellent catalysts that increasingly being used in industry and academia. This Perspective provides a general practical guide to enzymes their synthetic potential, primarily aimed at organic chemists.
Язык: Английский
Процитировано
261Nature Synthesis, Год журнала: 2023, Номер 2(6), С. 483 - 492
Опубликована: Янв. 30, 2023
Язык: Английский
Процитировано
259Advanced Materials, Год журнала: 2020, Номер 32(30)
Опубликована: Июнь 4, 2020
Abstract The optimal synthesis of advanced nanomaterials with numerous reaction parameters, stages, and routes, poses one the most complex challenges modern colloidal science, current strategies often fail to meet demands these combinatorially large systems. In response, an Artificial Chemist is presented: integration machine‐learning‐based experiment selection high‐efficiency autonomous flow chemistry. With self‐driving Chemist, made‐to‐measure inorganic perovskite quantum dots (QDs) in are autonomously synthesized, their yield composition polydispersity at target bandgaps, spanning 1.9 2.9 eV, simultaneously tuned. Utilizing eleven precision‐tailored QD compositions obtained without any prior knowledge, within 30 h, using less than 210 mL total starting solutions, user experiments. Using knowledge generated from studies, pre‐trained use a new batch precursors further accelerate synthetic path discovery compositions, by least twofold. knowledge‐transfer strategy enhances optoelectronic properties in‐flow synthesized QDs (within same resources as no‐prior‐knowledge experiments) mitigates issues batch‐to‐batch precursor variability, resulting averaging 1 meV peak emission energy.
Язык: Английский
Процитировано
258Matter, Год журнала: 2021, Номер 4(9), С. 2702 - 2726
Опубликована: Июль 26, 2021
Язык: Английский
Процитировано
258Nature, Год журнала: 2023, Номер 624(7992), С. 570 - 578
Опубликована: Дек. 20, 2023
Transformer-based large language models are making significant strides in various fields, such as natural processing
Язык: Английский
Процитировано
258Nature Reviews Materials, Год журнала: 2020, Номер 6(8), С. 655 - 678
Опубликована: Ноя. 9, 2020
Язык: Английский
Процитировано
254Journal of Cheminformatics, Год журнала: 2020, Номер 12(1)
Опубликована: Ноя. 17, 2020
We present the open-source AiZynthFinder software that can be readily used in retrosynthetic planning. The algorithm is based on a Monte Carlo tree search recursively breaks down molecule to purchasable precursors. guided by an artificial neural network policy suggests possible precursors utilizing library of known reaction templates. fast and typically find solution less than 10 s perform complete 1 min. Moreover, development code was range engineering principles such as automatic testing, system design continuous integration leading robust with high maintainability. Finally, well documented make it suitable for beginners. available at http://www.github.com/MolecularAI/aizynthfinder .
Язык: Английский
Процитировано
231Chemical Society Reviews, Год журнала: 2020, Номер 49(17), С. 6154 - 6168
Опубликована: Янв. 1, 2020
Chemists go ML! This tutorial review provides easy access to the fundamentals of machine learning from a synthetic chemist's perspective. Its diverse applications for molecular design, synthesis planning, or reactivity prediction are summarized.
Язык: Английский
Процитировано
226Drug Discovery Today, Год журнала: 2020, Номер 26(2), С. 511 - 524
Опубликована: Дек. 18, 2020
Although artificial intelligence (AI) has had a profound impact on areas such as image recognition, comparable advances in drug discovery are rare. This article quantifies the stages of which improvements time taken, success rate or affordability will have most overall bringing new drugs to market. Changes clinical rates improving discovery; other words, quality decisions regarding compound take forward (and how conduct trials) more important than speed cost. current AI focus make given compound, question make, using efficacy and safety-related end points, received significantly less attention. As consequence, proxy measures available data cannot fully utilize potential discovery, particular when it comes safety vivo. Thus, addressing questions generate points model be key clinically relevant decision-making future.
Язык: Английский
Процитировано
226