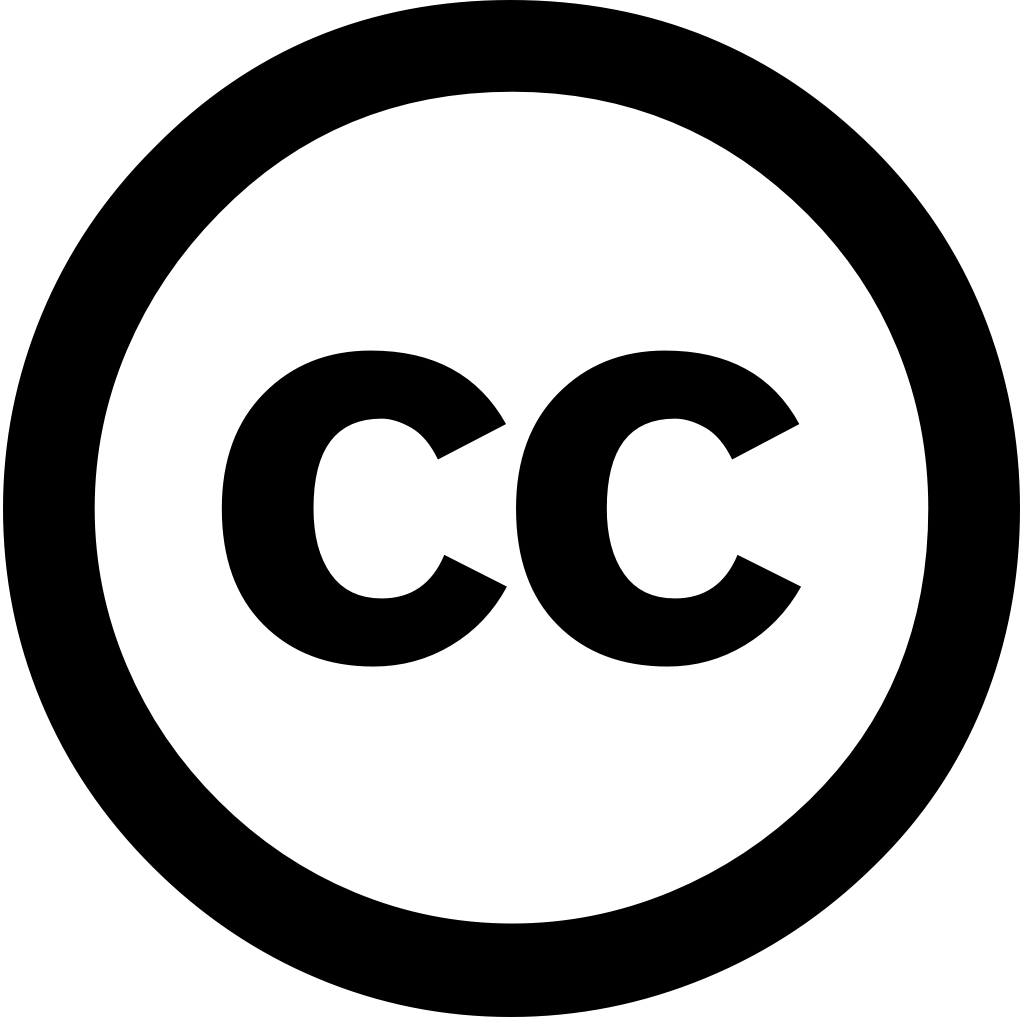
BMJ evidence-based medicine, Journal Year: 2024, Volume and Issue: unknown, P. bmjebm - 113199
Published: Dec. 20, 2024
Language: Английский
BMJ evidence-based medicine, Journal Year: 2024, Volume and Issue: unknown, P. bmjebm - 113199
Published: Dec. 20, 2024
Language: Английский
npj Digital Medicine, Journal Year: 2025, Volume and Issue: 8(1)
Published: Jan. 15, 2025
Can artificial intelligence improve clinical trial design? Despite their importance in medicine, over 40% of trials involve flawed protocols. We introduce and propose the development application-specific language models (ASLMs) for design across three phases: ASLM by regulatory agencies, customization Health Technology Assessment bodies, deployment to stakeholders. This strategy could enhance efficiency, inclusivity, safety, leading more representative, cost-effective trials.
Language: Английский
Citations
2Journal of Medical Internet Research, Journal Year: 2024, Volume and Issue: 26, P. e56780 - e56780
Published: May 31, 2024
Large language models (LLMs) such as ChatGPT have become widely applied in the field of medical research. In process conducting systematic reviews, similar tools can be used to expedite various steps, including defining clinical questions, performing literature search, document screening, information extraction, and refinement, thereby conserving resources enhancing efficiency. However, when using LLMs, attention should paid transparent reporting, distinguishing between genuine false content, avoiding academic misconduct. this viewpoint, we highlight potential roles LLMs creation reviews meta-analyses, elucidating their advantages, limitations, future research directions, aiming provide insights guidance for authors planning meta-analyses.
Language: Английский
Citations
9BMJ evidence-based medicine, Journal Year: 2025, Volume and Issue: unknown, P. bmjebm - 113320
Published: Jan. 9, 2025
Language: Английский
Citations
0npj Digital Medicine, Journal Year: 2025, Volume and Issue: 8(1)
Published: Jan. 31, 2025
Large language models (LLMs) have the potential to enhance evidence synthesis efficiency and accuracy. This study assessed LLM-only LLM-assisted methods in data extraction risk of bias assessment for 107 trials on complementary medicine. Moonshot-v1-128k Claude-3.5-sonnet achieved high accuracy (≥95%), with performing better (≥97%). significantly reduced processing time (14.7 5.9 min vs. 86.9 10.4 conventional methods). These findings highlight LLMs' when integrated human expertise.
Language: Английский
Citations
0Insights into Imaging, Journal Year: 2025, Volume and Issue: 16(1)
Published: Jan. 31, 2025
Language: Английский
Citations
0Clinical and Translational Science, Journal Year: 2025, Volume and Issue: 18(3)
Published: March 1, 2025
Despite interest in clinical trials with decentralized elements (DCTs), analysis of their trends trial registries is lacking due to heterogeneous designs and unstandardized terms. We explored Llama 3, an open-source large language model, efficiently evaluate these trends. Trial data were sourced from Aggregate Analysis ClinicalTrials.gov, focusing on drug conducted between 2018 2023. utilized three 3 models a different number parameters: 8b (model 1), fine-tuned 2) curated data, 70b 3). Prompt engineering enabled sophisticated tasks such as classification DCTs explanations extracting elements. Model performance, evaluated 3-month exploratory test dataset, demonstrated that sensitivity could be improved after fine-tuning 0.0357 0.5385. Low positive predictive value the model 2 by DCT-associated expressions 0.5385 0.9167. However, extraction was only properly performed which had larger parameters. Based results, we screened entire 6-year dataset applying expressions. After subsequent application identified 692 DCTs. found total 213 classified phase 2, followed 162 4 trials, 112 92 1 trials. In conclusion, our study potential for analyzing information not structured machine-readable format. Managing biases during crucial.
Language: Английский
Citations
0Research Synthesis Methods, Journal Year: 2025, Volume and Issue: unknown, P. 1 - 18
Published: March 12, 2025
Abstract Systematic reviews are essential for evidence-based health care, but conducting them is time- and resource-consuming. To date, efforts have been made to accelerate (semi-)automate various steps of systematic through the use artificial intelligence (AI) emergence large language models (LLMs) promises further opportunities. One crucial complex task within review conduct assessing risk bias (RoB) included studies. Therefore, aim this study was test LLM Claude 2 RoB assessment 100 randomized controlled trials, published in English from 2013 onwards, using revised Cochrane tool (‘RoB 2’; involving judgements five specific domains an overall judgement). We assessed agreement by with human reviews. The observed between authors ranged 41% judgement 71% domain 4 (‘outcome measurement’). Cohen’s κ lowest 5 (‘selective reporting’; 0.10 (95% confidence interval (CI): −0.10–0.31)) highest 3 (‘missing data’; 0.31 CI: 0.10–0.52)), indicating slight fair agreement. Fair found (Cohen’s κ: 0.22 0.06–0.38)). Sensitivity analyses alternative prompting techniques or more recent version did not result substantial changes. Currently, Claude’s cannot replace assessment. However, potential LLMs support should be explored.
Language: Английский
Citations
0Journal of Evidence-Based Medicine, Journal Year: 2025, Volume and Issue: 18(1)
Published: March 1, 2025
Language: Английский
Citations
0Journal of Evidence-Based Medicine, Journal Year: 2025, Volume and Issue: 18(1)
Published: March 1, 2025
ABSTRACT Objective To evaluate the methodological and reporting quality of clinical practice guidelines/expert consensus for ambulatory surgery centers published since 2000, combining manual assessment with large language model (LLM) analysis, while exploring LLMs' feasibility in evaluation. Methods We systematically searched Chinese/English databases guideline repositories. Two researchers independently screened literature extracted data. Quality assessments were conducted using AGREE II RIGHT tools through both evaluation GPT‐4o modeling. Results 54 eligible documents included. domains showed mean compliance: Scope purpose 25.00%, Stakeholder involvement 20.16%, Rigor development 17.28%, Clarity presentation 41.56%, Applicability 18.06%, Editorial independence 26.39%. items averaged: Basic information 44.44%, Background 36.11%, Evidence 14.07%, Recommendations 34.66%, Review assurance 3.70%, Funding declaration management interests 24.54%, Other 27.16%. LLMs'‐evaluated demonstrated significantly higher scores than tools. Subgroup analyses revealed superior evidence retrieval, conflict disclosure, funding support, LLM integration ( P <0.05). Conclusion Current guidelines related to day need improve their reporting. The study validates supplementary value emphasizing necessity maintaining as foundation.
Language: Английский
Citations
0BMJ evidence-based medicine, Journal Year: 2025, Volume and Issue: unknown, P. bmjebm - 113066
Published: April 8, 2025
Objective To assess custom GPT-4 performance in extracting and evaluating data from medical literature to assist the systematic review (SR) process. Design A proof-of-concept comparative study was conducted accuracy precision of models against human-performed reviews randomised controlled trials (RCTs). Setting Four were developed, each specialising one following areas: (1) extraction characteristics, (2) outcomes, (3) bias assessment domains (4) evaluation risk using results third model. Model outputs compared four SRs by human authors. The focused on extraction, replicating outcomes agreement levels assessments. Participants Among chosen, 43 studies retrieved for evaluation. Additionally, 17 RCTs selected comparison assessments, where both comparator an analogous SR provided assessments comparison. Intervention Custom deployed extract evaluate studies, their those generated reviewers. Main outcome measures Concordance rates between effect size comparability inter/intra-rater Results When comparing automatically extracted first table characteristics published review, showed 88.6% concordance with original <5% discrepancies due inaccuracies or omissions. It exceeded 2.5% instances. Study pooling comparable sizes SRs. fair-moderate but significant intra-rater (ICC=0.518, p<0.001) inter-rater agreements (weighted kappa=0.237) kappa=0.296). In contrast, there a poor two kappa=0.094). Conclusion Customized perform well precise potential utilization bias. While evaluated tasks are simpler than broader range methodologies, they provide important initial GPT-4's capabilities.
Language: Английский
Citations
0