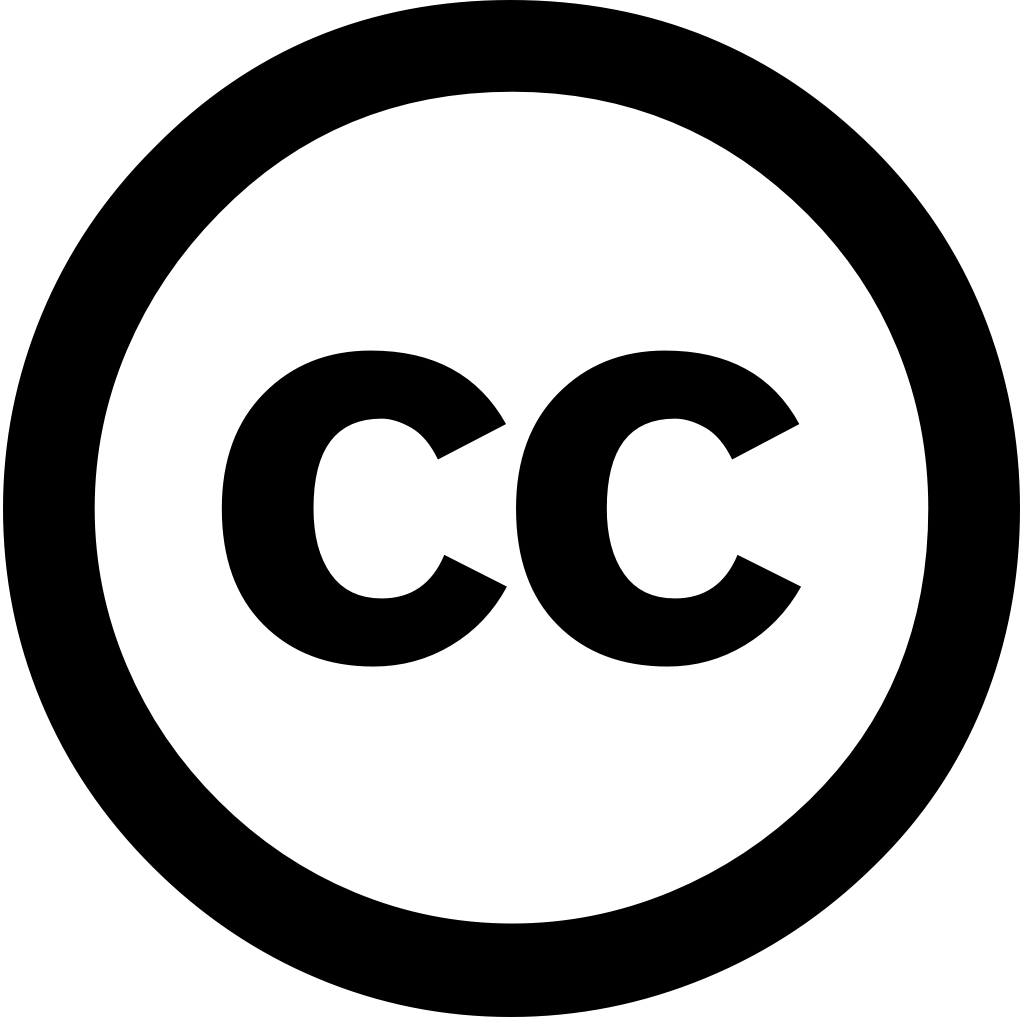
JMIR Cancer, Journal Year: 2024, Volume and Issue: unknown
Published: Dec. 8, 2024
Language: Английский
JMIR Cancer, Journal Year: 2024, Volume and Issue: unknown
Published: Dec. 8, 2024
Language: Английский
JAMA Network Open, Journal Year: 2024, Volume and Issue: 7(6), P. e2417641 - e2417641
Published: June 18, 2024
Importance Large language models (LLMs) recently developed an unprecedented ability to answer questions. Studies of LLMs from other fields may not generalize medical oncology, a high-stakes clinical setting requiring rapid integration new information. Objective To evaluate the accuracy and safety LLM answers on oncology examination Design, Setting, Participants This cross-sectional study was conducted between May 28 October 11, 2023. The American Society Clinical Oncology (ASCO) Self-Assessment Series ASCO Connection, European Medical (ESMO) Examination Trial questions, original set board-style multiple-choice questions were presented 8 LLMs. Main Outcomes Measures primary outcome percentage correct answers. oncologists evaluated explanations provided by best for accuracy, classified types errors, estimated likelihood extent potential harm. Results Proprietary 2 correctly answered 125 147 (85.0%; 95% CI, 78.2%-90.4%; P < .001 vs random answering). outperformed earlier version, proprietary 1, which 89 (60.5%; 52.2%-68.5%; .001), open-source LLM, Mixtral-8x7B-v0.1, 87 (59.2%; 50.0%-66.4%; .001). contained no or minor errors 138 (93.9%; 88.7%-97.2%). Incorrect responses most commonly associated with in information retrieval, particularly recent publications, followed erroneous reasoning reading comprehension. If acted upon practice, 18 22 incorrect (81.8%; 59.7%-94.8%) would have medium high moderate severe Conclusions Relevance In this performance remarkable performance, although raised concerns. These results demonstrated opportunity develop improve health care clinician experiences patient care, considering impact capabilities safety.
Language: Английский
Citations
17Cancer Medicine, Journal Year: 2025, Volume and Issue: 14(1)
Published: Jan. 1, 2025
ABSTRACT Purpose Caregivers in pediatric oncology need accurate and understandable information about their child's condition, treatment, side effects. This study assesses the performance of publicly accessible large language model (LLM)‐supported tools providing valuable reliable to caregivers children with cancer. Methods In this cross‐sectional study, we evaluated four LLM‐supported tools—ChatGPT (GPT‐4), Google Bard (Gemini Pro), Microsoft Bing Chat, SGE—against a set frequently asked questions (FAQs) derived from Children's Oncology Group Family Handbook expert input (In total, 26 FAQs 104 generated responses). Five experts assessed LLM responses using measures including accuracy, clarity, inclusivity, completeness, clinical utility, overall rating. Additionally, content quality was readability, AI disclosure, source credibility, resource matching, originality. We used descriptive analysis statistical tests Shapiro–Wilk, Levene's, Kruskal–Wallis H ‐tests, Dunn's post hoc for pairwise comparisons. Results ChatGPT shows high when by experts. also performed well, especially accuracy clarity responses, whereas Chat SGE had lower scores. Regarding disclosure being AI, it observed less which may have affected maintained balance between response clarity. most readable answered complexity. varied significantly ( p < 0.001) across all evaluations except inclusivity. Through our thematic free‐text comments, emotional tone empathy emerged as unique theme mixed feedback on expectations be empathetic. Conclusion can enhance caregivers' knowledge oncology. Each has strengths areas improvement, indicating careful selection based specific contexts. Further research is required explore application other medical specialties patient demographics, assessing broader applicability long‐term impacts.
Language: Английский
Citations
3Clinical and Translational Radiation Oncology, Journal Year: 2025, Volume and Issue: 51, P. 100914 - 100914
Published: Jan. 7, 2025
Language: Английский
Citations
1NEJM AI, Journal Year: 2025, Volume and Issue: unknown
Published: Jan. 13, 2025
Language: Английский
Citations
1Resuscitation, Journal Year: 2024, Volume and Issue: unknown, P. 110404 - 110404
Published: Sept. 1, 2024
Language: Английский
Citations
7Strahlentherapie und Onkologie, Journal Year: 2025, Volume and Issue: unknown
Published: Jan. 10, 2025
Abstract Background This study aims to evaluate the capabilities and limitations of large language models (LLMs) for providing patient education men undergoing radiotherapy localized prostate cancer, incorporating assessments from both clinicians patients. Methods Six questions about definitive cancer were designed based on common inquiries. These presented different LLMs [ChatGPT‑4, ChatGPT-4o (both OpenAI Inc., San Francisco, CA, USA), Gemini (Google LLC, Mountain View, Copilot (Microsoft Corp., Redmond, WA, Claude (Anthropic PBC, USA)] via respective web interfaces. Responses evaluated readability using Flesch Reading Ease Index. Five radiation oncologists assessed responses relevance, correctness, completeness a five-point Likert scale. Additionally, 35 patients ChatGPT‑4 comprehensibility, accuracy, trustworthiness, overall informativeness. Results The Index indicated that all relatively difficult understand. All provided answers found be generally relevant correct. ChatGPT‑4, ChatGPT-4o, AI also complete. However, we significant differences between performance regarding relevance completeness. Some lacked detail or contained inaccuracies. Patients perceived information as easy understand relevant, with most expressing confidence in willingness use future medical questions. ChatGPT-4’s helped feel better informed, despite initially standardized provided. Conclusion Overall, show promise tool radiotherapy. While improvements are needed terms accuracy readability, positive feedback suggests can enhance understanding engagement. Further research is essential fully realize potential artificial intelligence education.
Language: Английский
Citations
0Frontiers in Artificial Intelligence, Journal Year: 2025, Volume and Issue: 7
Published: Jan. 14, 2025
Introduction Generating physician letters is a time-consuming task in daily clinical practice. Methods This study investigates local fine-tuning of large language models (LLMs), specifically LLaMA models, for letter generation privacy-preserving manner within the field radiation oncology. Results Our findings demonstrate that base without fine-tuning, are inadequate effectively generating letters. The QLoRA algorithm provides an efficient method intra-institutional LLMs with limited computational resources (i.e., single 48 GB GPU workstation hospital). fine-tuned LLM successfully learns oncology-specific information and generates institution-specific style. ROUGE scores generated summary reports highlight superiority 8B LLaMA-3 model over 13B LLaMA-2 model. Further multidimensional evaluations 10 cases reveal that, although has capacity to generate content beyond provided input data, it salutations, diagnoses treatment histories, recommendations further treatment, planned schedules. Overall, benefit was rated highly by experts (average score 3.4 on 4-point scale). Discussion With careful review correction, automated LLM-based significant practical value.
Language: Английский
Citations
0Diagnostics, Journal Year: 2025, Volume and Issue: 15(6), P. 735 - 735
Published: March 15, 2025
Publications on the application of artificial intelligence (AI) to many situations, including those in clinical medicine, created 2023–2024 are reviewed here. Because short time frame covered, here, it is not possible conduct exhaustive analysis as would be case meta-analyses or systematic reviews. Consequently, this literature review presents an examination narrative AI’s relation contemporary topics related medicine. The landscape findings here span 254 papers published 2024 topically reporting AI which 83 articles considered present because they contain evidence-based findings. In particular, types cases deal with accuracy initial differential diagnoses, cancer treatment recommendations, board-style exams, and performance various tasks, imaging. Importantly, summaries validation techniques used evaluate presented. This focuses AIs that have a relevancy evidenced by evaluation publications. speaks both what has been promised delivered systems. Readers will able understand when generative may expressing views without having necessary information (ultracrepidarianism) responding if had expert knowledge does not. A lack awareness deliver inadequate confabulated can result incorrect medical decisions inappropriate applications (Dunning–Kruger effect). As result, certain cases, system might underperform provide results greatly overestimate any validity.
Language: Английский
Citations
0Research Square (Research Square), Journal Year: 2025, Volume and Issue: unknown
Published: April 3, 2025
Language: Английский
Citations
0Frontiers in Oral Health, Journal Year: 2025, Volume and Issue: 6
Published: April 7, 2025
Patients frequently seek dental information online, and generative pre-trained transformers (GPTs) may be a valuable resource. However, the quality of responses based on varying prompt designs has not been evaluated. As implant treatment is widely performed, this study aimed to investigate influence design GPT performance in answering commonly asked questions related implants. Thirty about dentistry - covering patient selection, associated risks, peri-implant disease symptoms, for missing teeth, prevention, prognosis were posed four different models with designs. Responses recorded independently appraised by two periodontists across six domains. All performed well, classified as good quality. The contextualized model worse treatment-related (21.5 ± 3.4, p < 0.05), but outperformed input-output, zero-shot chain thought, instruction-tuned citing appropriate sources its (4.1 1.0, 0.001). had less clarity relevance compared other models. GPTs can provide accurate, complete, useful While enhance response quality, further refinement necessary optimize performance.
Language: Английский
Citations
0