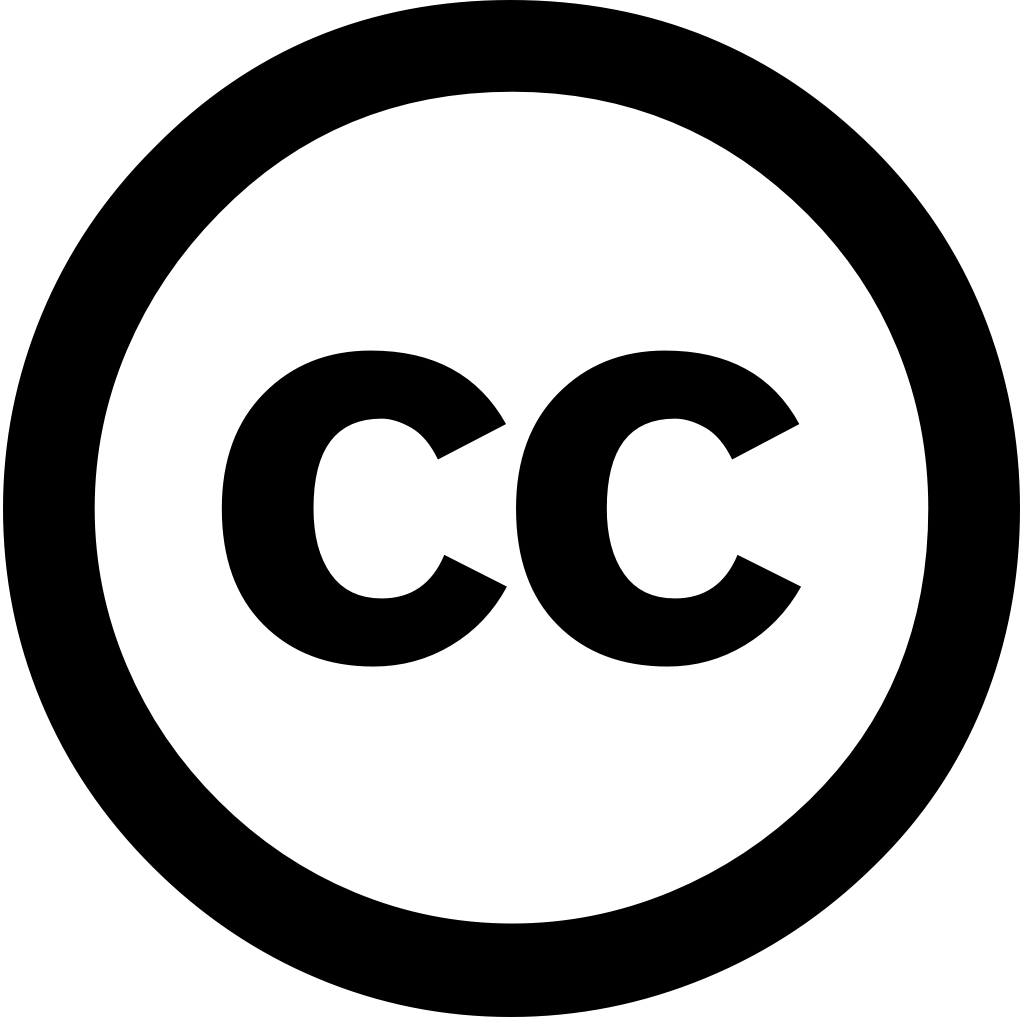
Symmetry, Journal Year: 2025, Volume and Issue: 17(2), P. 160 - 160
Published: Jan. 22, 2025
Imbalanced data have become an essential stumbling block in the field of machine learning. In this paper, a novel oversampling method based on local density estimation, namely LD-SMOTE, is presented to address constraints popular rebalance technique SMOTE. LD-SMOTE initiates with k-means clustering quantificationally measure classification contribution each feature. Subsequently, distance metric grounded Jaccard similarity defined, which accentuates features that are more intricately linked minority class. Utilizing metric, we estimate Gaussian-like function control quantity synthetic samples around every sample, thus simulating distribution Additionally, generation occurs within triangular region constructed by sample and its two chosen neighbors instead line connecting one neighbors. Experimental comparisons between 16 existing resampling methods 19 datasets reveal significant average increase 6.4% accuracy, 4.4% F-measure, 5.4% G-mean, 4.0% AUC. This result indicates can be alternative for imbalanced datasets.
Language: Английский