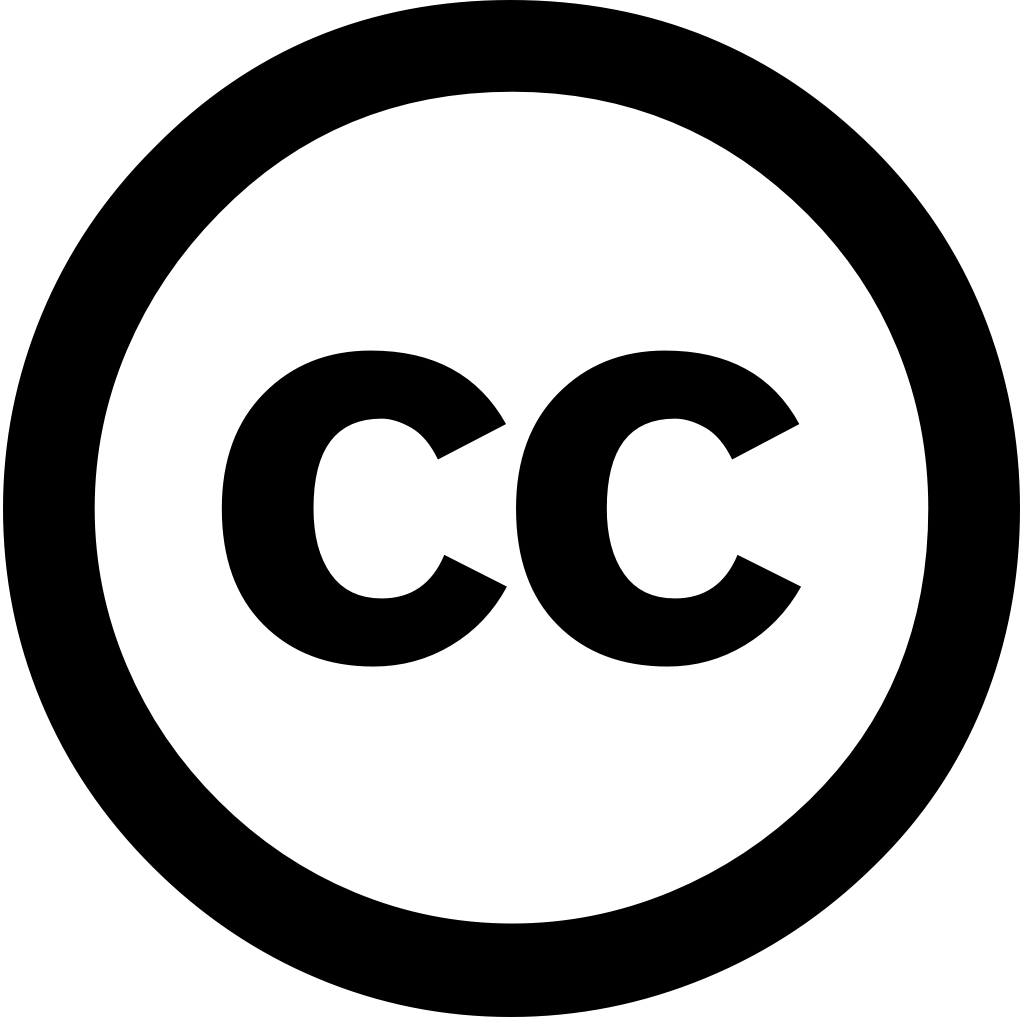
Meta-Radiology, Journal Year: 2024, Volume and Issue: unknown, P. 100102 - 100102
Published: Sept. 1, 2024
Language: Английский
Meta-Radiology, Journal Year: 2024, Volume and Issue: unknown, P. 100102 - 100102
Published: Sept. 1, 2024
Language: Английский
Advanced Science, Journal Year: 2024, Volume and Issue: 11(32)
Published: June 23, 2024
Mesoscopic photoacoustic imaging (PAI) enables label-free visualization of vascular networks in tissues with high contrast and resolution. Segmenting these from 3D PAI data interpreting their physiological pathological significance is crucial yet challenging due to the time-consuming error-prone nature current methods. Deep learning offers a potential solution; however, supervised analysis frameworks typically require human-annotated ground-truth labels. To address this, an unsupervised image-to-image translation deep model introduced, Vessel Segmentation Generative Adversarial Network (VAN-GAN). VAN-GAN integrates synthetic blood vessel that closely resemble real-life anatomy into its training process learns replicate underlying physics system order learn how segment vasculature images. Applied diverse range silico, vitro, vivo data, including patient-derived breast cancer xenograft models clinical angiograms, demonstrates capability facilitate accurate unbiased segmentation networks. By leveraging reduces reliance on manual labeling, thus lowering barrier entry for high-quality (F1 score: vs. U-Net = 0.84 0.87) enhancing preclinical research structure function.
Language: Английский
Citations
2Meta-Radiology, Journal Year: 2024, Volume and Issue: unknown, P. 100102 - 100102
Published: Sept. 1, 2024
Language: Английский
Citations
1