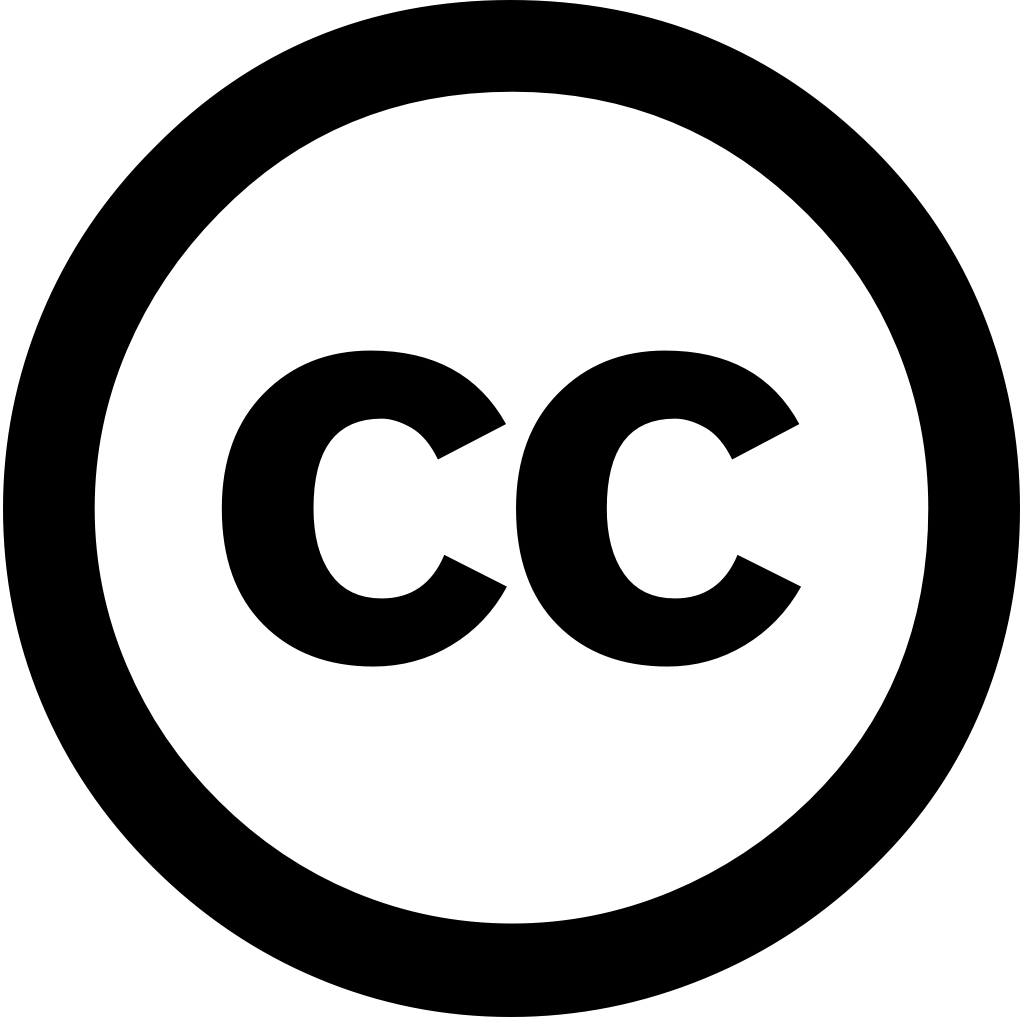
Nature Materials, Journal Year: 2025, Volume and Issue: unknown
Published: April 28, 2025
Language: Английский
Nature Materials, Journal Year: 2025, Volume and Issue: unknown
Published: April 28, 2025
Language: Английский
Crystals, Journal Year: 2025, Volume and Issue: 15(5), P. 393 - 393
Published: April 23, 2025
The potential of machine learning (ML) models for predicting crystallographic symmetry information from single-phase powder X-ray diffraction (XRD) patterns is investigated. Given the scarcity large, labeled experimental datasets, we train our using simulated XRD generated databases. A key challenge in developing reliable diffraction-based structure-solution tools lies limited availability training data and presence natural adversarial examples, which hinder model generalization. To address these issues, explore multiple pipelines testing strategies, including evaluations on data. We introduce a contrastive representation approach that significantly outperforms previous supervised terms robustness generalizability, demonstrating improved invariance to effects. These results highlight self-supervised advancing ML-driven analysis.
Language: Английский
Citations
0Nature Materials, Journal Year: 2025, Volume and Issue: unknown
Published: April 28, 2025
Language: Английский
Citations
0